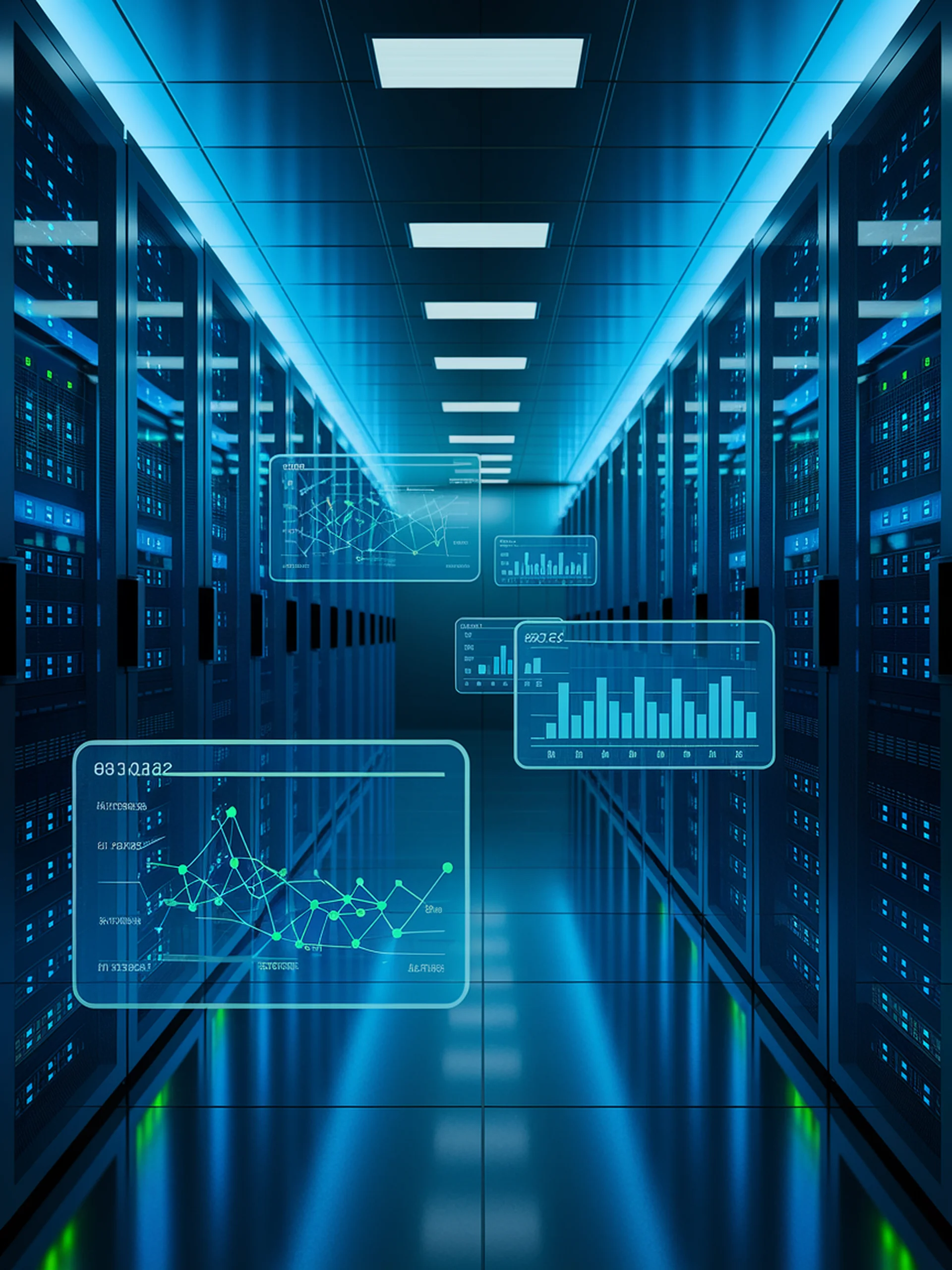
Supercharging Data Deduplication for LLMs
GPU-Accelerated Framework for Faster, More Efficient Dataset Processing
FED introduces a highly optimized GPU-accelerated framework that significantly improves dataset deduplication performance for training large language models.
- Implements non-cryptographic hash functions and GPU cluster optimization to achieve superior speed and efficiency
- Outperforms existing GPU-based deduplication methods through innovative technical optimizations
- Enhances data quality for LLM training with minimal computational overhead
- Demonstrates practical engineering solutions to a critical AI infrastructure challenge
This research addresses a key bottleneck in AI model development by providing a more efficient way to deduplicate massive datasets, ultimately improving both training efficiency and model performance.
FED: Fast and Efficient Dataset Deduplication Framework with GPU Acceleration