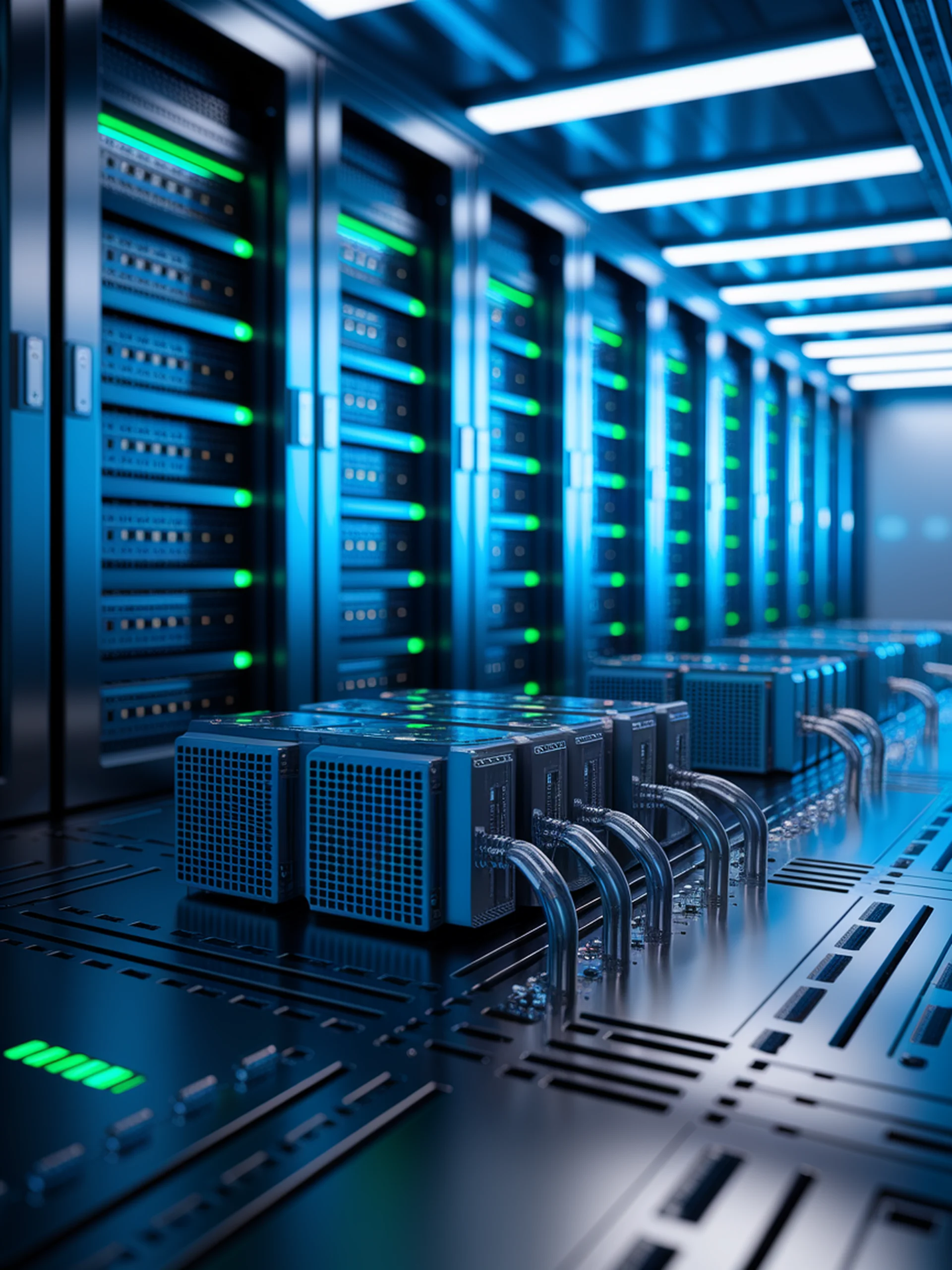
HALO: Efficient Low-Precision LLM Training
Enabling accurate quantized training for large language models
HALO introduces a Hadamard-assisted optimization technique that solves the challenge of training LLMs in low precision without accuracy loss.
- Maintains model performance while reducing computational requirements
- Particularly effective when fine-tuning pre-trained models with outlier values
- Successfully handles quantization challenges that previous methods struggled with
- Represents a significant engineering advancement for more efficient LLM development
This research matters because it directly addresses one of the major computational bottlenecks in AI development: the enormous resources required to train and fine-tune large language models. By enabling lower-precision operations without sacrificing accuracy, HALO could substantially reduce hardware requirements and energy consumption for AI research and deployment.
HALO: Hadamard-Assisted Lower-Precision Optimization for LLMs