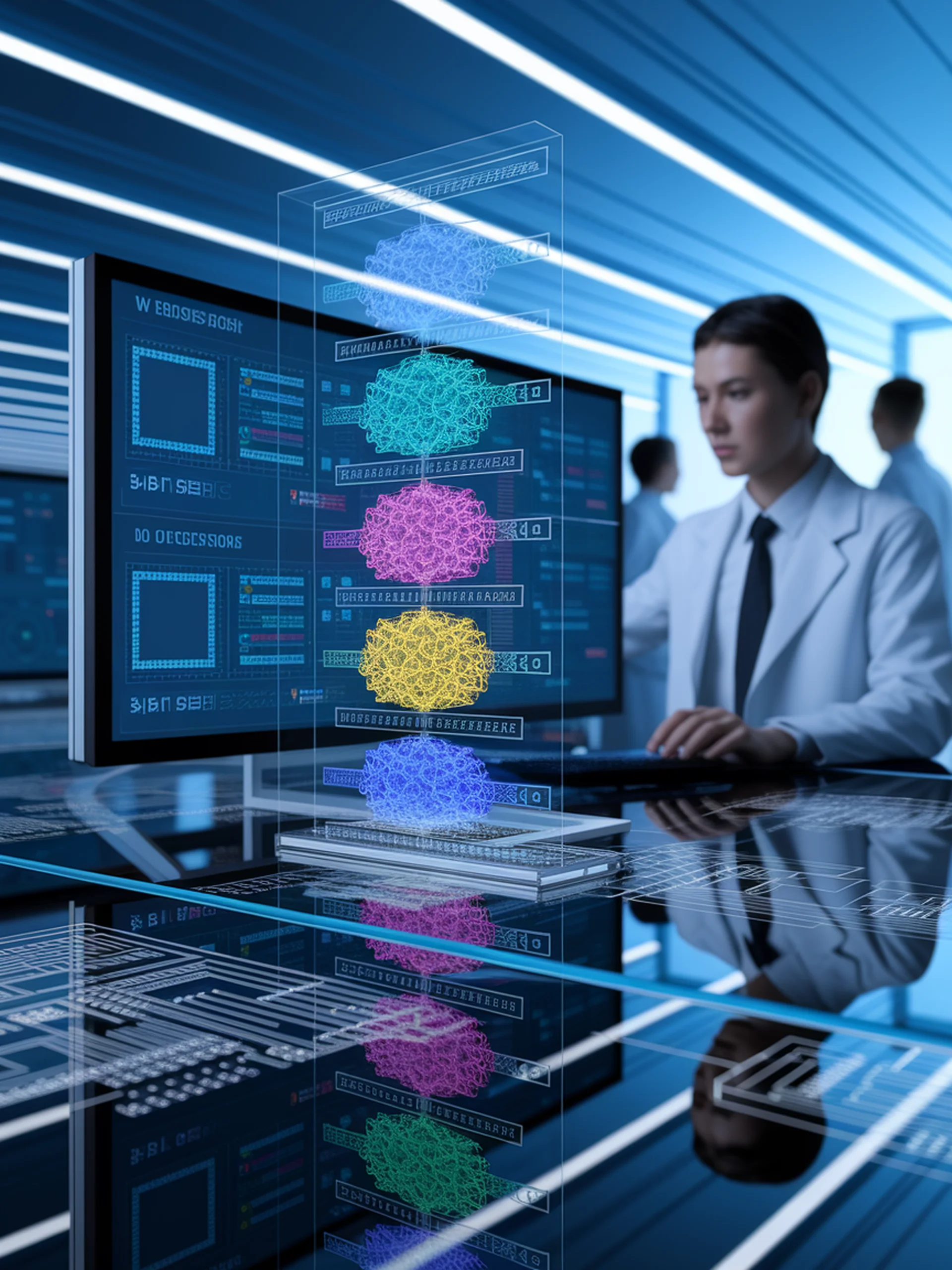
Self-Improving Multimodal LLMs
Enhancing reasoning capabilities through cascaded self-evaluation
This research introduces Self-Evaluation Augmented Training (SEAT), a novel approach that significantly improves reasoning capabilities in lightweight multimodal language models by teaching them to evaluate their own reasoning processes.
- Creates a cascaded training process where powerful models guide smaller ones
- Addresses limitations in Chain-of-Thought (CoT) reasoning through structured self-evaluation
- Achieves substantial performance gains while maintaining model efficiency
- Implements a three-tier evaluation structure to maximize reasoning quality
For educational applications, this approach enables more accurate and explainable AI systems that can better support student learning through improved reasoning and self-correction capabilities.
Cascaded Self-Evaluation Augmented Training for Lightweight Multimodal LLMs