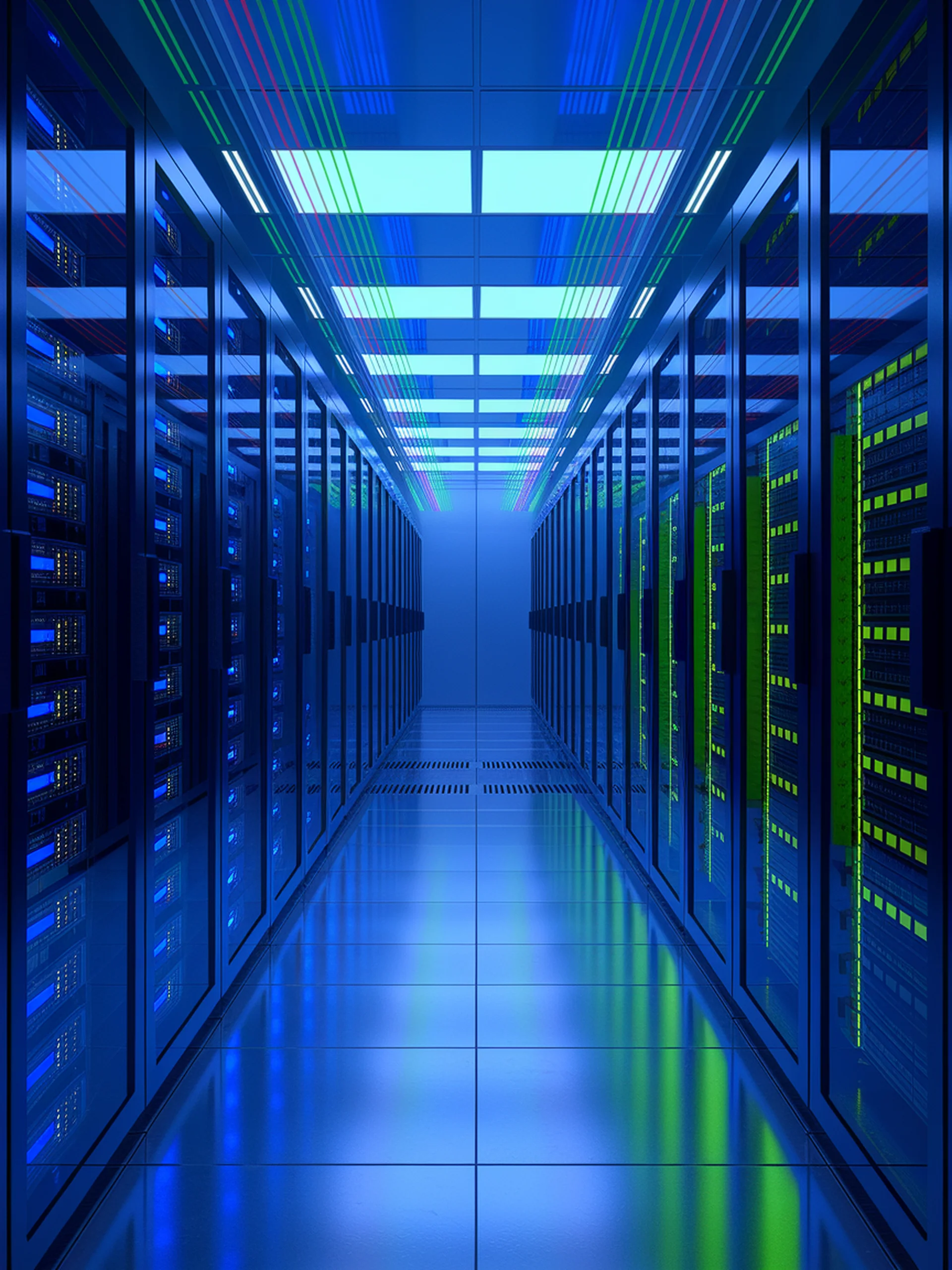
Optimizing LLM Performance with Glinthawk
A two-tiered approach to enhance offline LLM inference efficiency
Glinthawk introduces a novel architecture that strategically separates LLM inference across two compute tiers, optimizing resource utilization and cost-efficiency.
- Tier 1: High-end accelerators focus solely on processing model weights
- Tier 2: Lower-end compute resources handle attention mechanism and key-value cache
- This separation enables larger batch sizes and more efficient accelerator usage
- The architecture allows memory demands to scale independently from model weights
For engineering teams, Glinthawk represents a significant advancement in LLM deployment architecture, potentially reducing infrastructure costs while improving throughput for offline inference tasks.
Glinthawk: A Two-Tiered Architecture for Offline LLM Inference