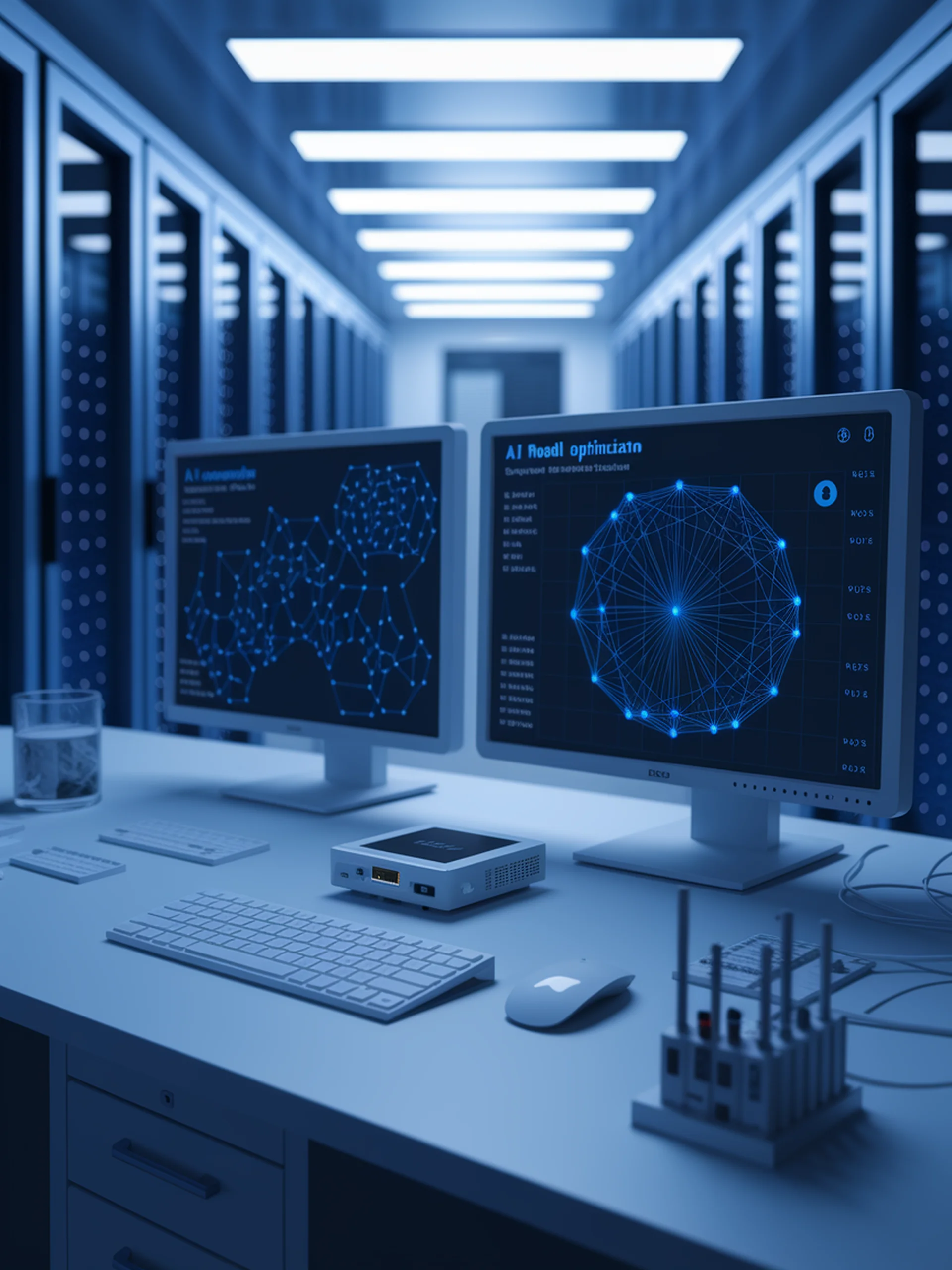
Shrinking LLMs Without Sacrificing Performance
A novel approach to efficient AI with selective pruning and weight-sharing
FlexiGPT introduces a principled methodology for creating memory-efficient large language models that can operate on resource-constrained devices.
- Selectively prunes model blocks based on calculated importance scores
- Replaces pruned blocks with innovative low-parameter alternatives
- Implements weight-sharing mechanisms to maintain performance while reducing size
- Enables deployment of powerful language models on memory-limited hardware
This research addresses a critical engineering challenge: how to make increasingly complex AI systems accessible across a broader range of devices without compromising their capabilities. By enabling more efficient deployment, FlexiGPT helps bridge the gap between cutting-edge AI research and practical applications.
FlexiGPT: Pruning and Extending Large Language Models with Low-Rank Weight Sharing