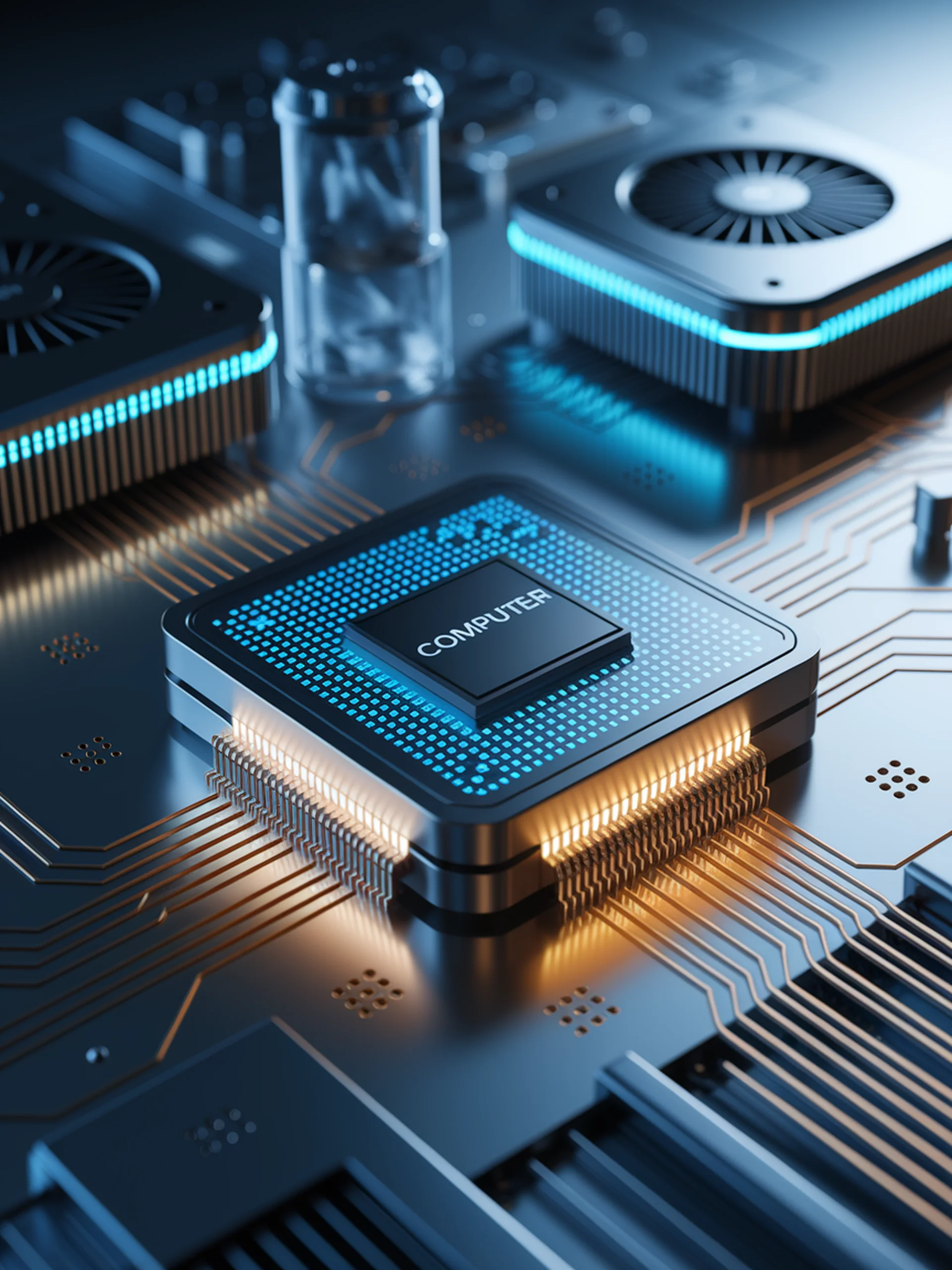
Smarter AI Compression: The One-Shot Approach
Policy-based pruning eliminates calibration dataset requirements
This research introduces a novel calibration-free LLM compression technique that employs reinforcement learning to determine optimal pruning strategies without external datasets.
- Achieves up to 3× compression ratio without significant performance loss
- Uses a neural policy network to identify which parameters to prune
- Adapts automatically to different compression requirements without retraining
- Outperforms existing methods across multiple benchmarks with faster runtime
Engineering Impact: This approach significantly reduces deployment barriers for resource-constrained environments by enabling more efficient AI systems while maintaining performance integrity.
You Only Prune Once: Designing Calibration-Free Model Compression With Policy Learning