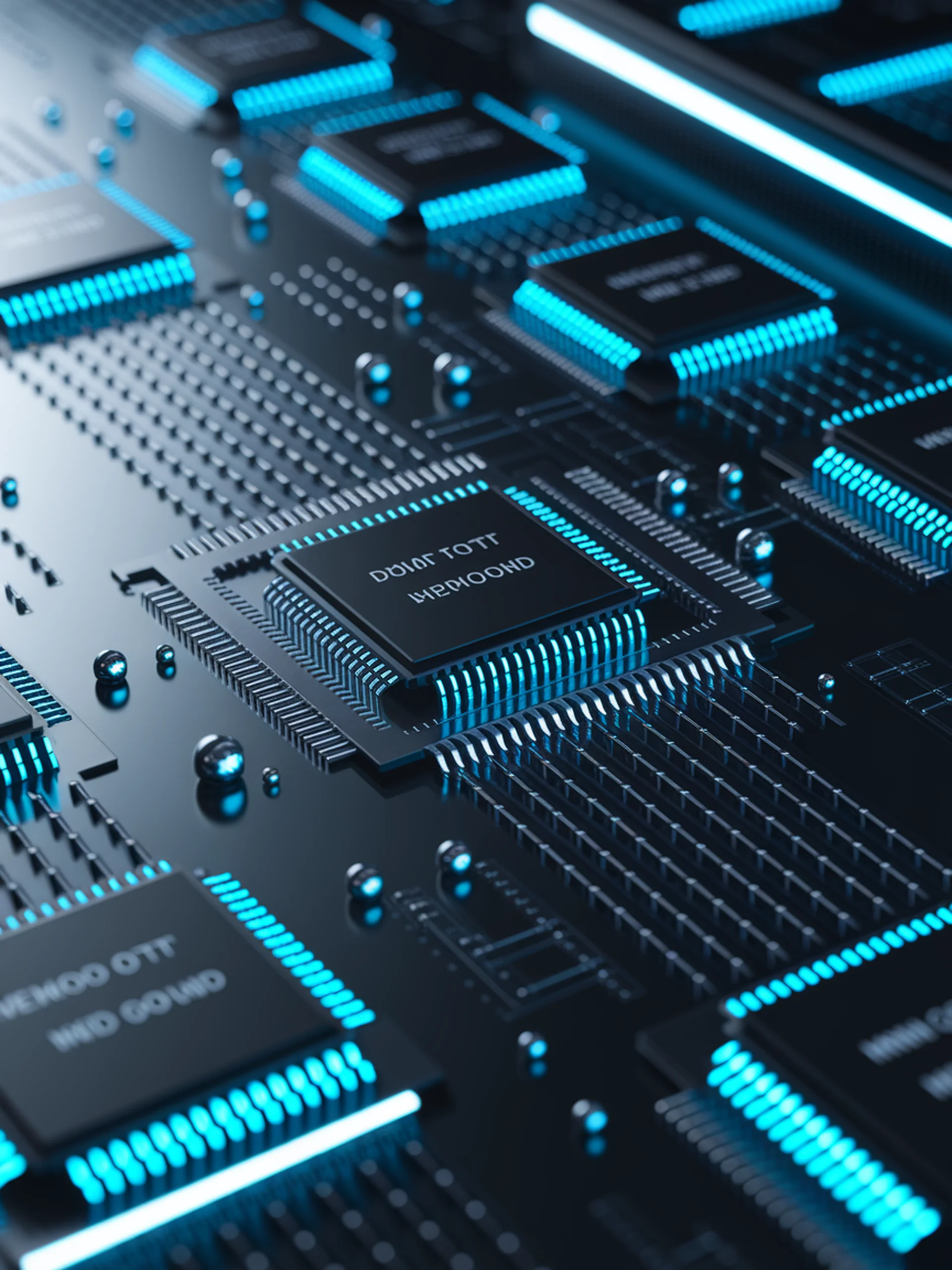
Squeezing More from LLM Memory
2-bit KV Cache Compression with Robust Performance
RotateKV introduces a novel technique for extreme compression of Key-Value caches in Large Language Models while maintaining performance quality, addressing a critical memory bottleneck for LLM inference.
- Achieves accurate 2-bit quantization by using adaptive rotations to handle outlier values
- Removes the memory bottleneck for serving LLMs with long contexts or large batch sizes
- Maintains robustness at extreme compression ratios without compromising model performance
- Provides practical engineering solution for more efficient LLM deployment
This innovation enables significant memory savings during inference, making LLMs more practical to deploy in resource-constrained environments and potentially reducing cloud computing costs for AI applications.