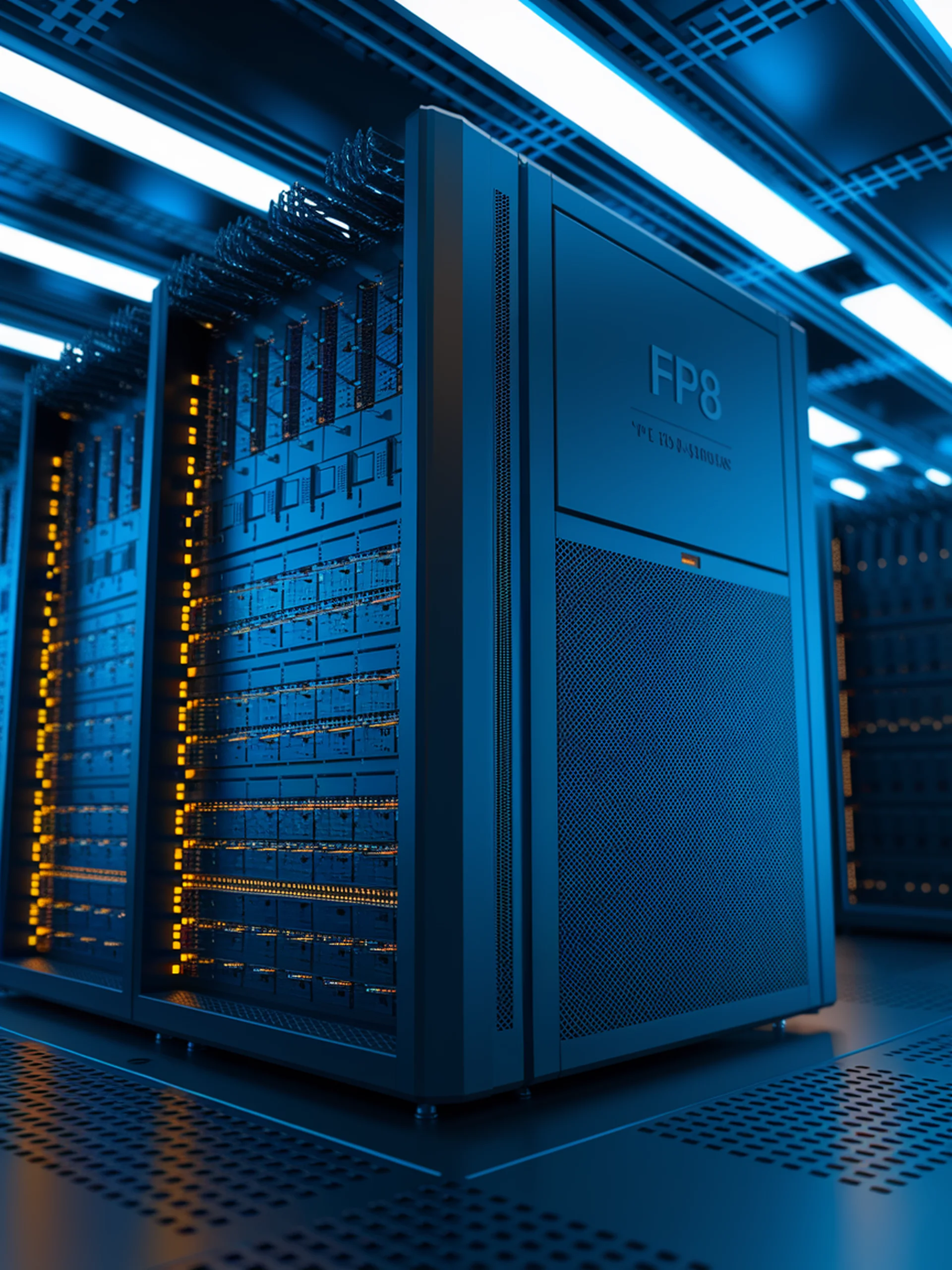
Breaking the FP8 Barrier in LLM Training
First successful implementation of FP4 precision for efficient LLM training
This research achieves a breakthrough in computational efficiency for large language models by successfully implementing FP4 precision training, potentially cutting hardware requirements by half.
- Introduces a differentiable quantization estimator to mitigate quantization errors
- Develops a mixed-precision quantization scheme that selectively applies FP4 to less sensitive operations
- Achieves comparable model quality to FP16 training while using significantly less memory and computation
- Demonstrates practical viability with models up to 7B parameters
This engineering advance represents a critical step toward more accessible LLM training, potentially enabling more organizations to develop custom models with reduced infrastructure costs.
Optimizing Large Language Model Training Using FP4 Quantization