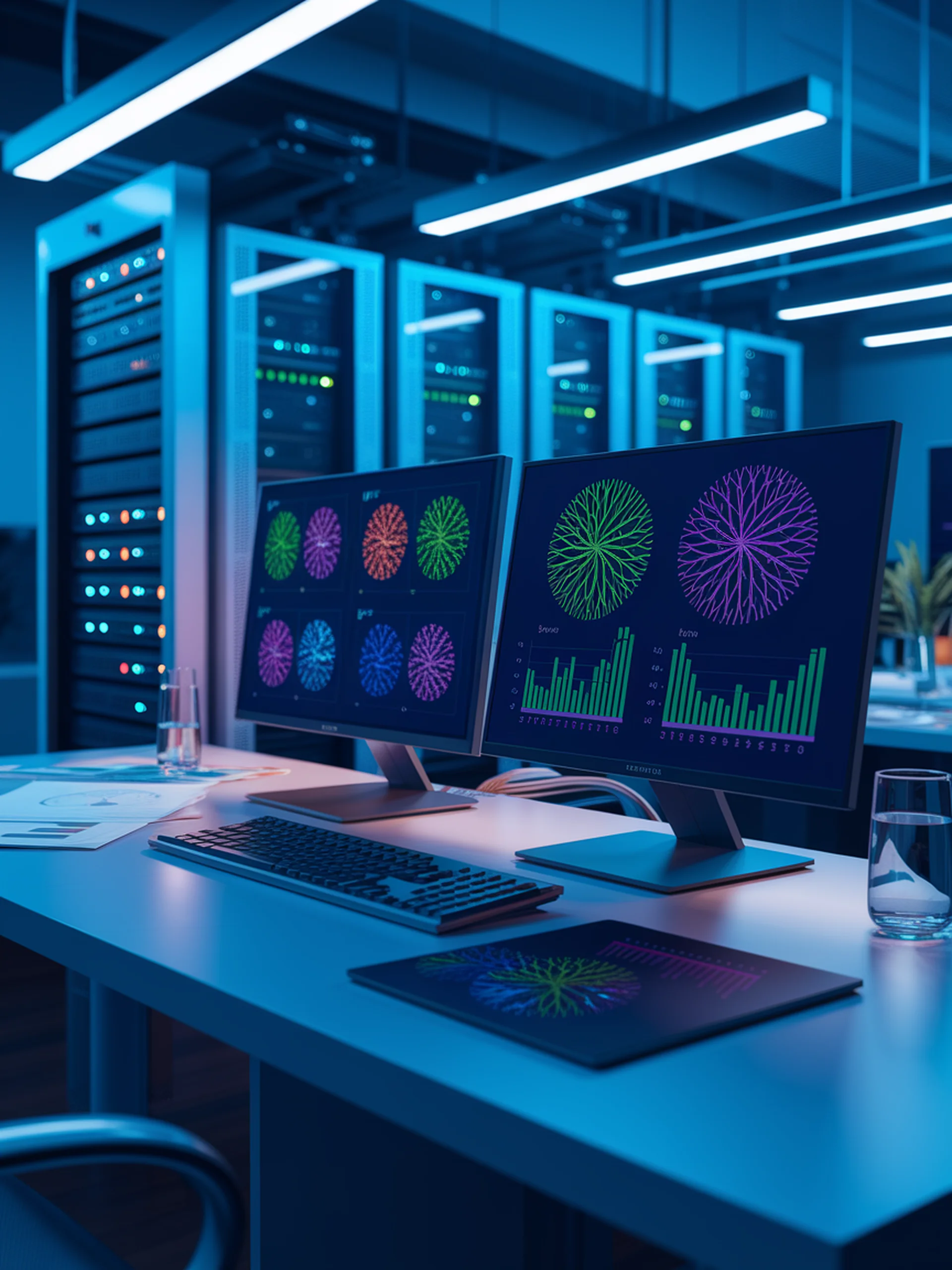
Streamlining LLMs for Better Efficiency
A data-driven approach to pruning without performance loss
This research introduces a novel regularized structured pruning method that reduces LLM size while preserving performance, avoiding the typical degradation seen in traditional prune-then-finetune approaches.
- Achieves up to 32.5% model size reduction with minimal performance impact
- Uses data-driven regularization to identify and maintain important model components
- Implements structured pruning for practical deployment benefits
- Demonstrates effectiveness across various model sizes and tasks
This innovation addresses critical engineering challenges in LLM deployment by significantly reducing computational and memory requirements without sacrificing capabilities—making advanced AI more accessible for resource-constrained applications.
DReSS: Data-driven Regularized Structured Streamlining for Large Language Models