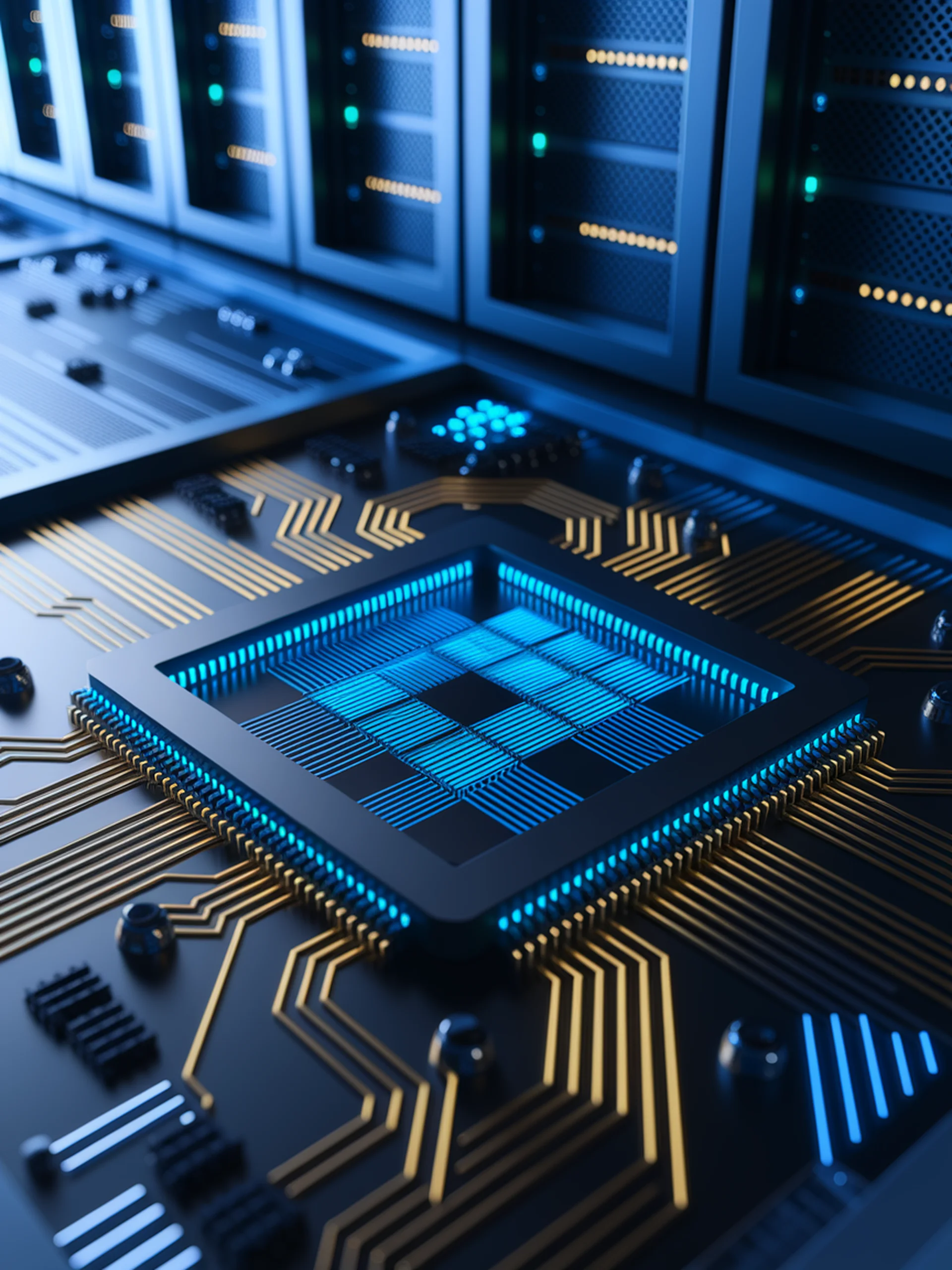
Smarter Parameter Updates for LLMs
Enhancing training efficiency with selective parameter optimization
AlphaAdam introduces a novel optimization framework that selectively updates parameters within layers of large language models, improving training efficiency without sacrificing model quality.
- Achieves comparable performance to full updates while requiring fewer computational resources
- Implements dynamic masking mechanism to identify and update only the most important parameters
- Demonstrates stability improvements across various model architectures and training scenarios
- Provides a practical solution for reducing the computational burden of training large language models
This research matters because it addresses a critical engineering challenge in AI: making LLM training more accessible by reducing computational requirements, potentially democratizing access to state-of-the-art language models.
AlphaAdam: Asynchronous Masked Optimization with Dynamic Alpha for Selective Updates