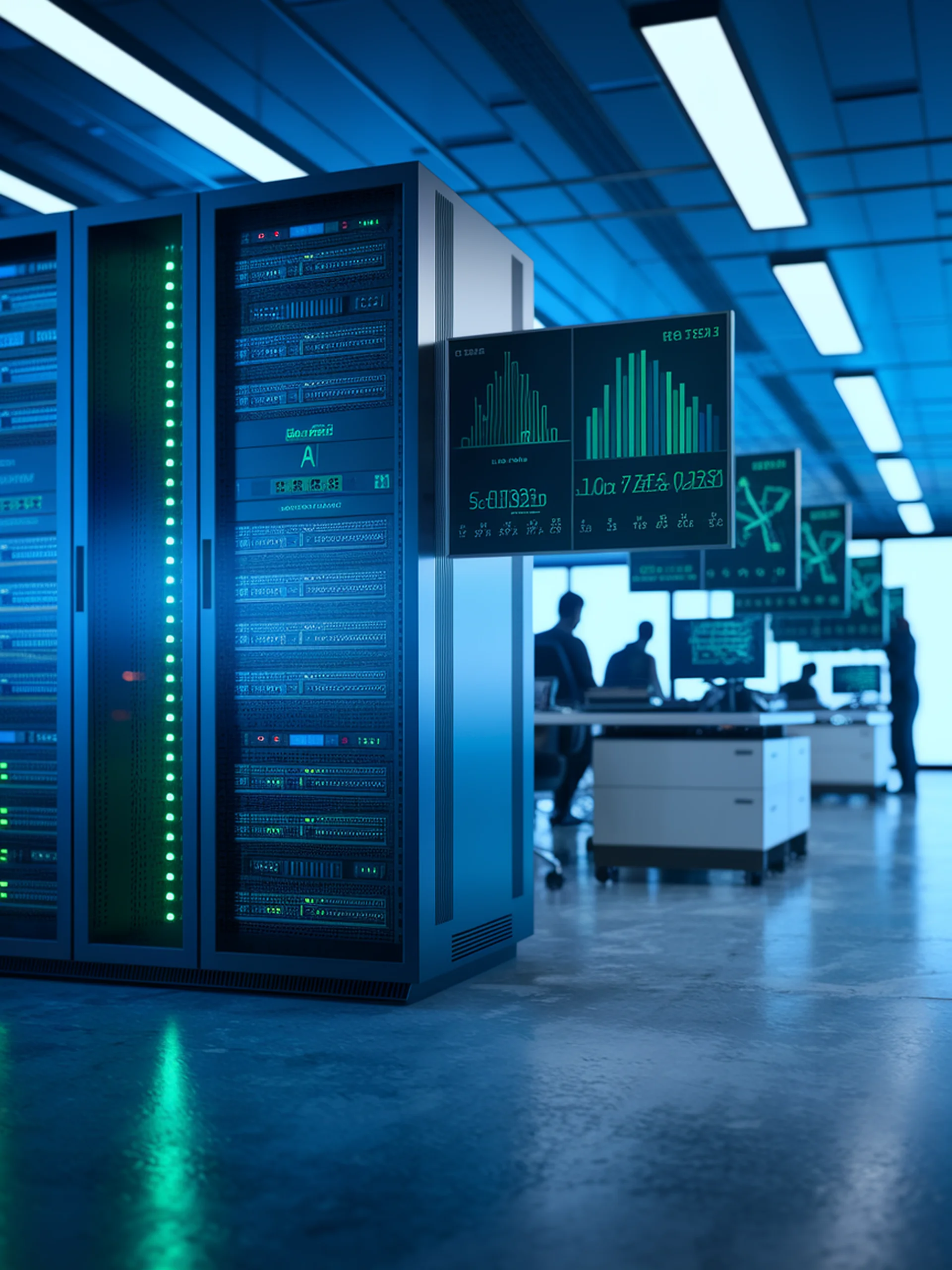
Optimizing LLMs for Inference Speed
Rethinking scaling laws to balance performance and efficiency
This research introduces a novel approach to optimize large language models by considering inference efficiency alongside model size and training data.
Key Findings:
- Models of identical size can have up to 3.5x difference in latency based on architecture alone
- Modified Chinchilla scaling laws to co-optimize parameter count, training tokens, and architecture
- Developed models that maintain accuracy while significantly improving inference speed
For engineering teams, this research provides a practical framework to develop LLMs that not only perform well but are also cost-effective to deploy in production environments, addressing a critical gap in current scaling approaches.