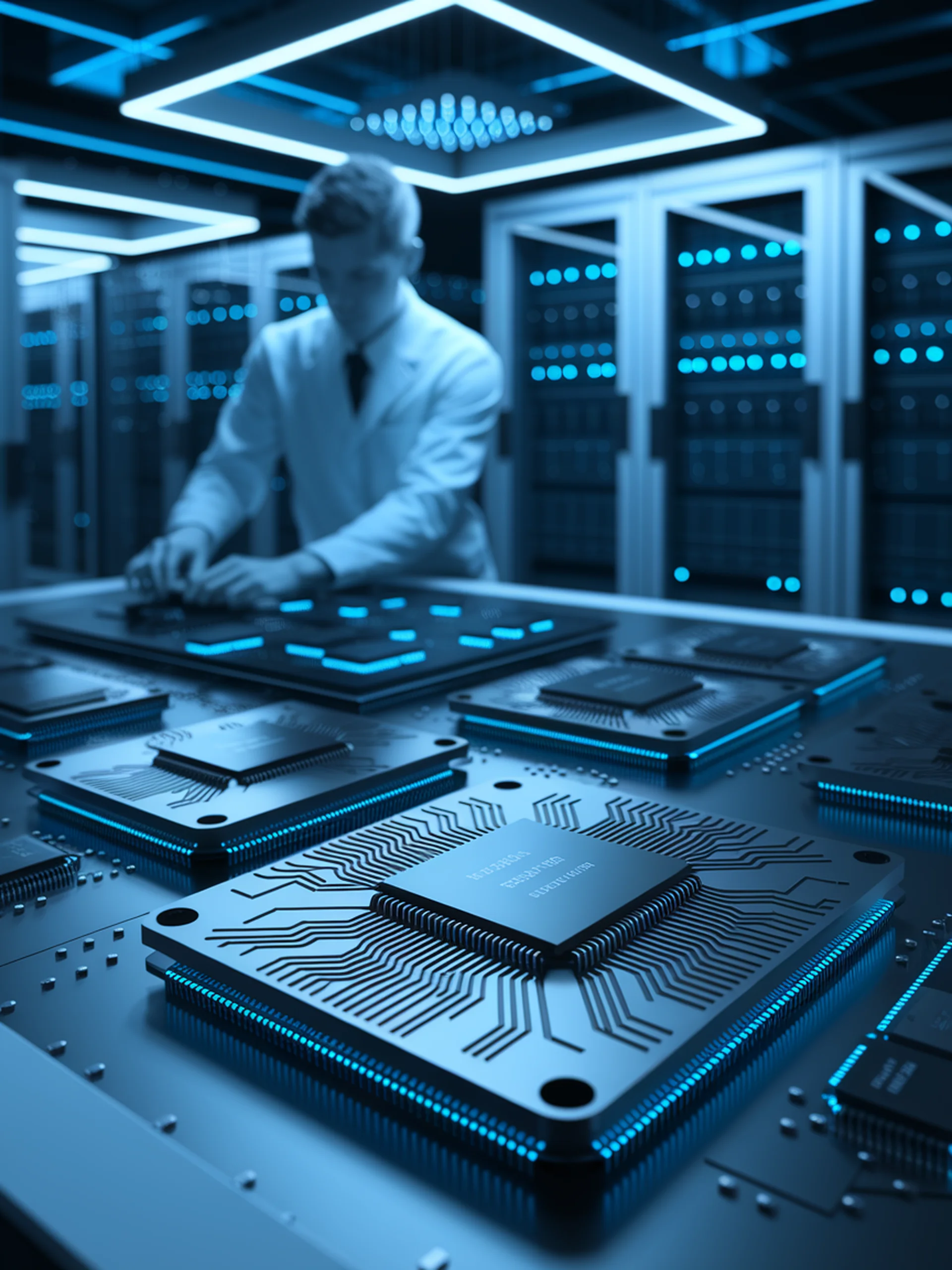
Optimizing LLMs with Block Floating Point
Breaking efficiency barriers for nonlinear operations in LLMs
This research advances Block Floating Point (BFP) techniques to address computational bottlenecks in large language model deployment, particularly for nonlinear operations.
- Extends BFP optimization beyond linear operations to challenging nonlinear functions
- Tackles the quadratic computational complexity in Attention mechanisms
- Enables more efficient hardware implementation through specialized formats
- Significantly reduces memory and computational demands for LLM inference
For engineering teams, this work represents a crucial advancement in hardware-software co-design that could enable more efficient LLM deployment on resource-constrained systems and specialized hardware like FPGAs and ASICs.