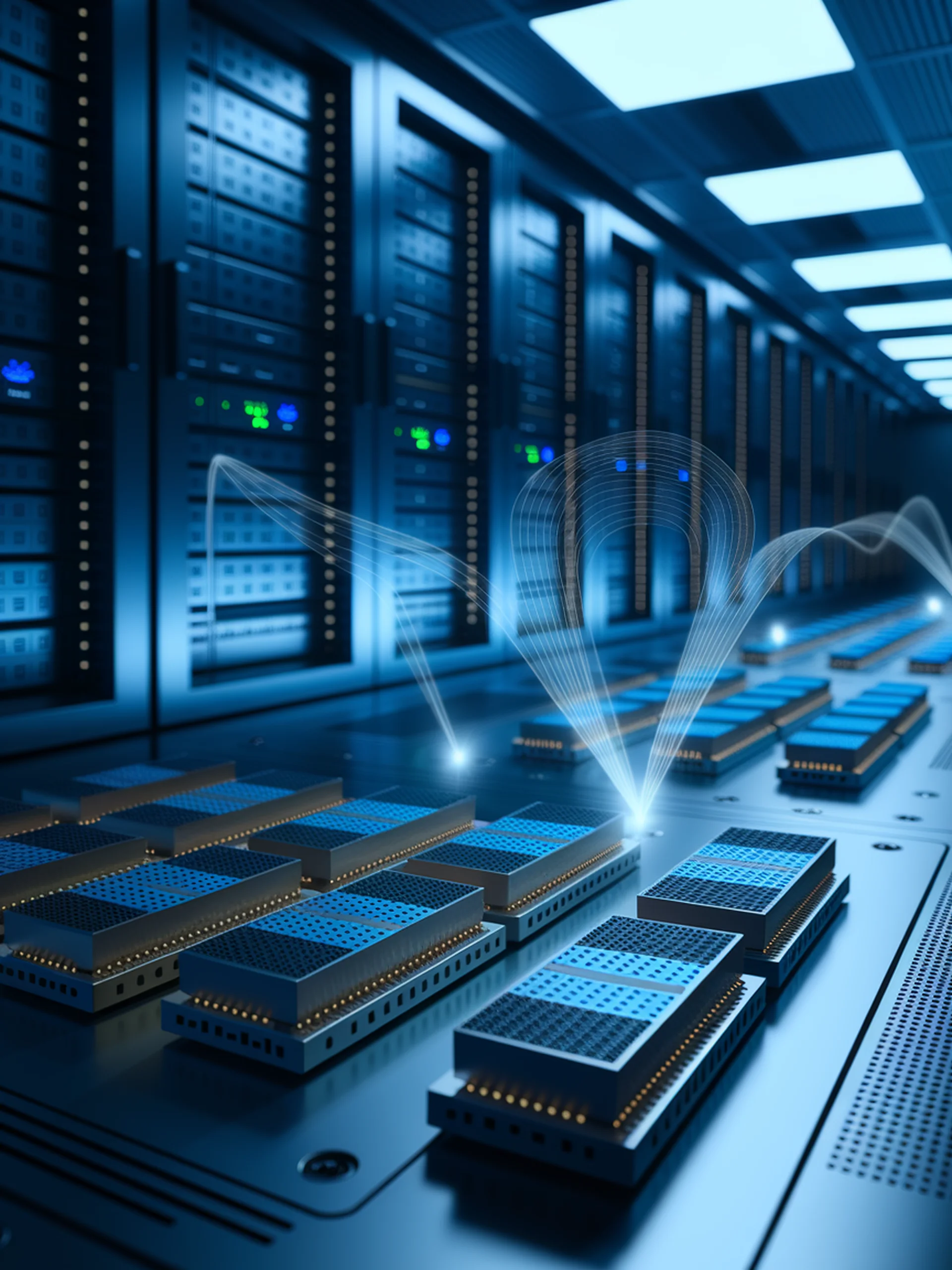
Making LLMs Memory-Efficient
Semantic-Aware Compression for Long-Context AI
ChunkKV introduces a novel approach to compress memory requirements for large language models by preserving semantic relationships between tokens.
- Treats groups of tokens as compression units rather than individual tokens
- Improves efficiency in long-context inference while maintaining model performance
- Implements layer-wise index reuse to further reduce computational overhead
- Demonstrates superior performance compared to existing KV cache compression methods
This engineering breakthrough enables more efficient deployment of LLMs for applications requiring long context windows, reducing computational costs while preserving model capabilities.
ChunkKV: Semantic-Preserving KV Cache Compression for Efficient Long-Context LLM Inference