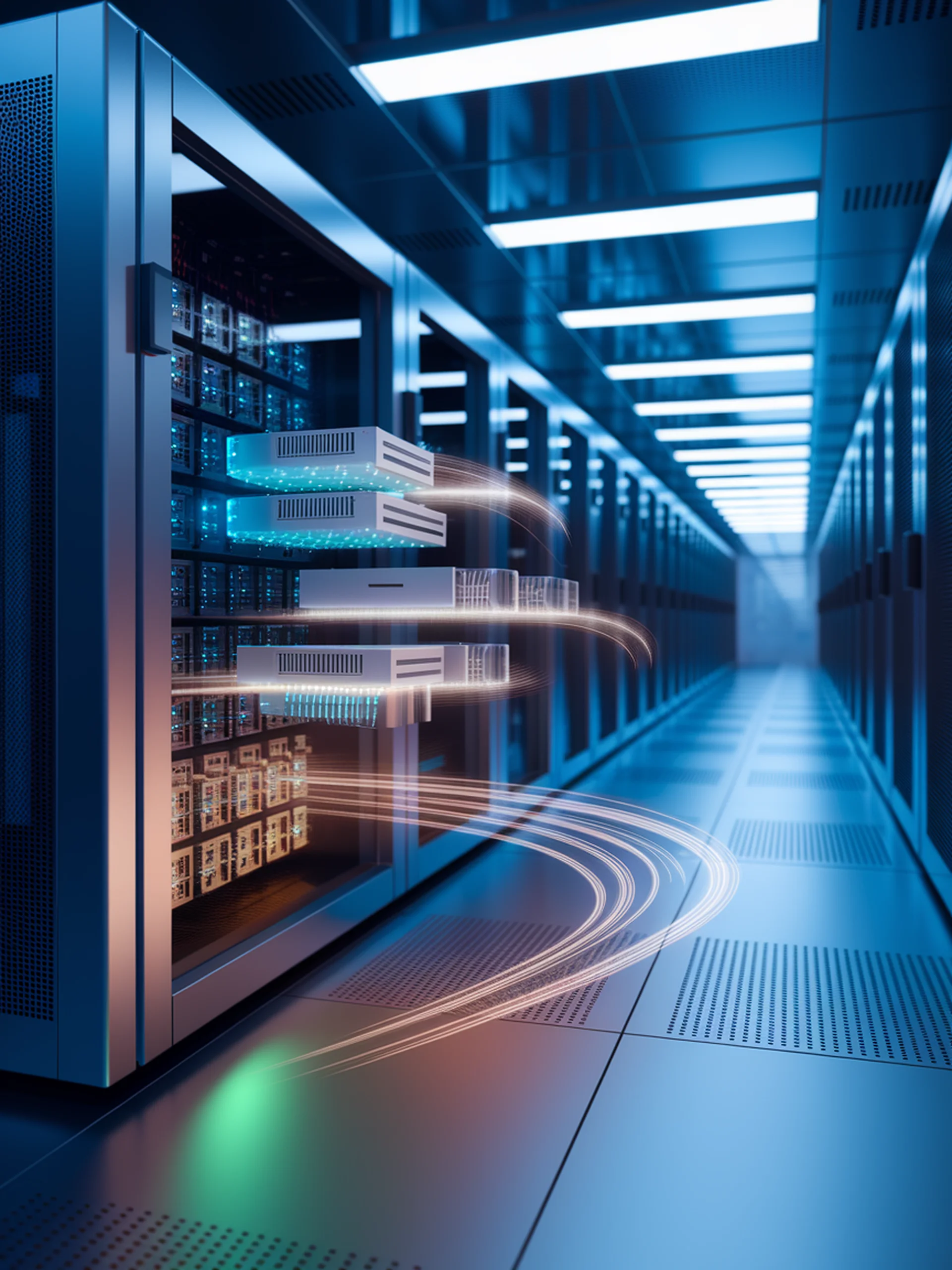
Making Multimodal AI Faster & Lighter
Full Static Quantization for Efficient Multimodal LLMs
MQuant introduces a breakthrough approach to significantly reduce the computational demands of multimodal large language models without sacrificing performance.
- Addresses the unique challenges of quantizing vision-language models
- Achieves up to 76% reduction in model size and 2.3x faster inference
- Proposes a novel calibration process specifically designed for multimodal architectures
- Maintains comparable performance to full-precision models across various benchmarks
This research enables practical deployment of powerful multimodal AI systems on resource-constrained devices, making advanced vision-language capabilities more accessible for real-world applications.