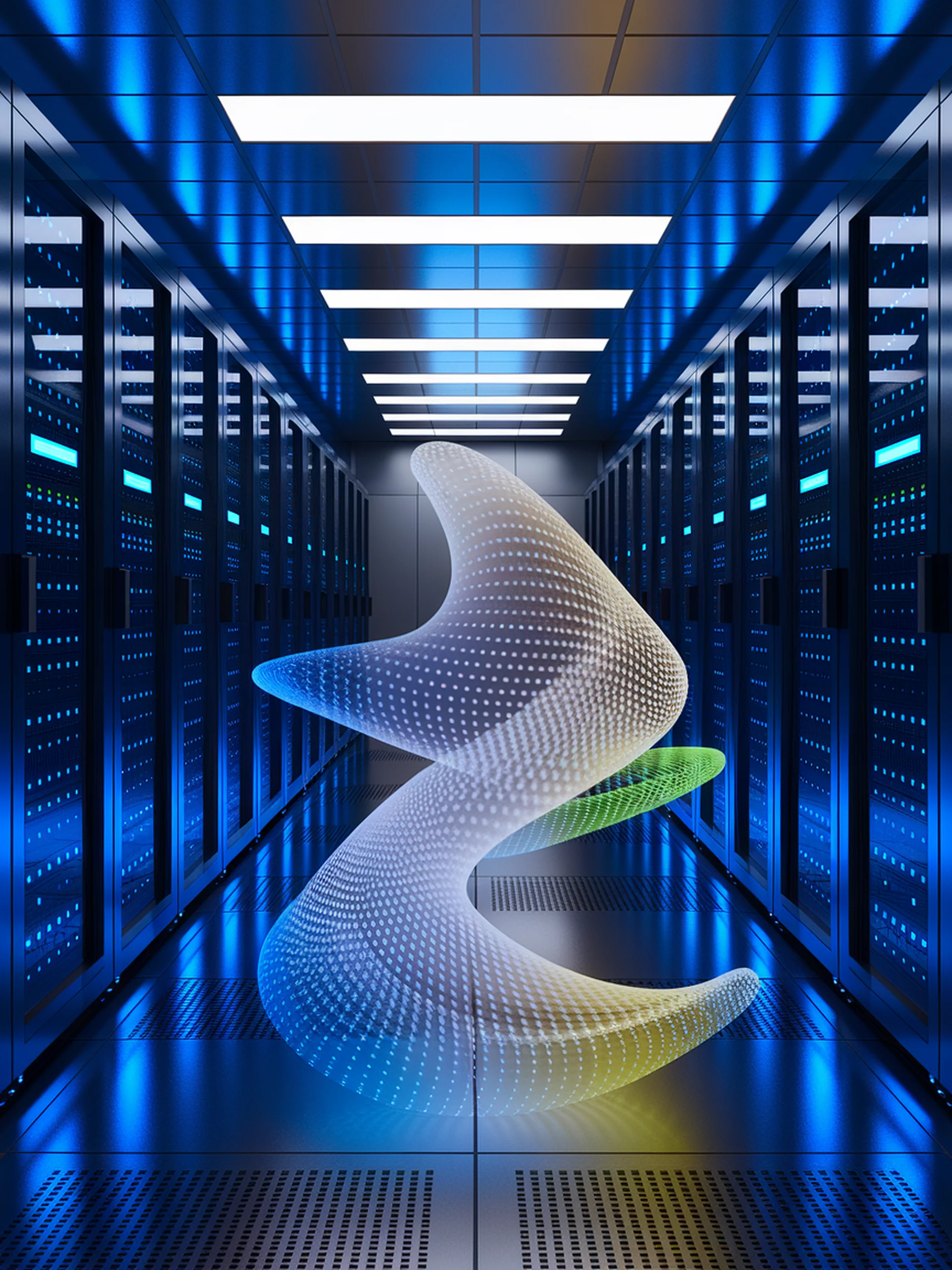
PolarQuant: Slashing Memory Costs in LLMs
A breakthrough approach to key cache quantization
PolarQuant introduces a novel transformation technique to efficiently compress the memory-intensive KV cache in large language models, addressing the critical outlier challenge that limited previous approaches.
- Transforms key vectors into polar space to better manage outliers that typically appear in only one dimension
- Reduces memory consumption while maintaining model performance
- Enables broader deployment of LLMs on resource-constrained devices
- Achieves significant inference acceleration with minimal accuracy loss
This engineering innovation matters because memory usage represents a major bottleneck for LLM deployment in practical applications, particularly on edge devices and in scenarios with limited computational resources.