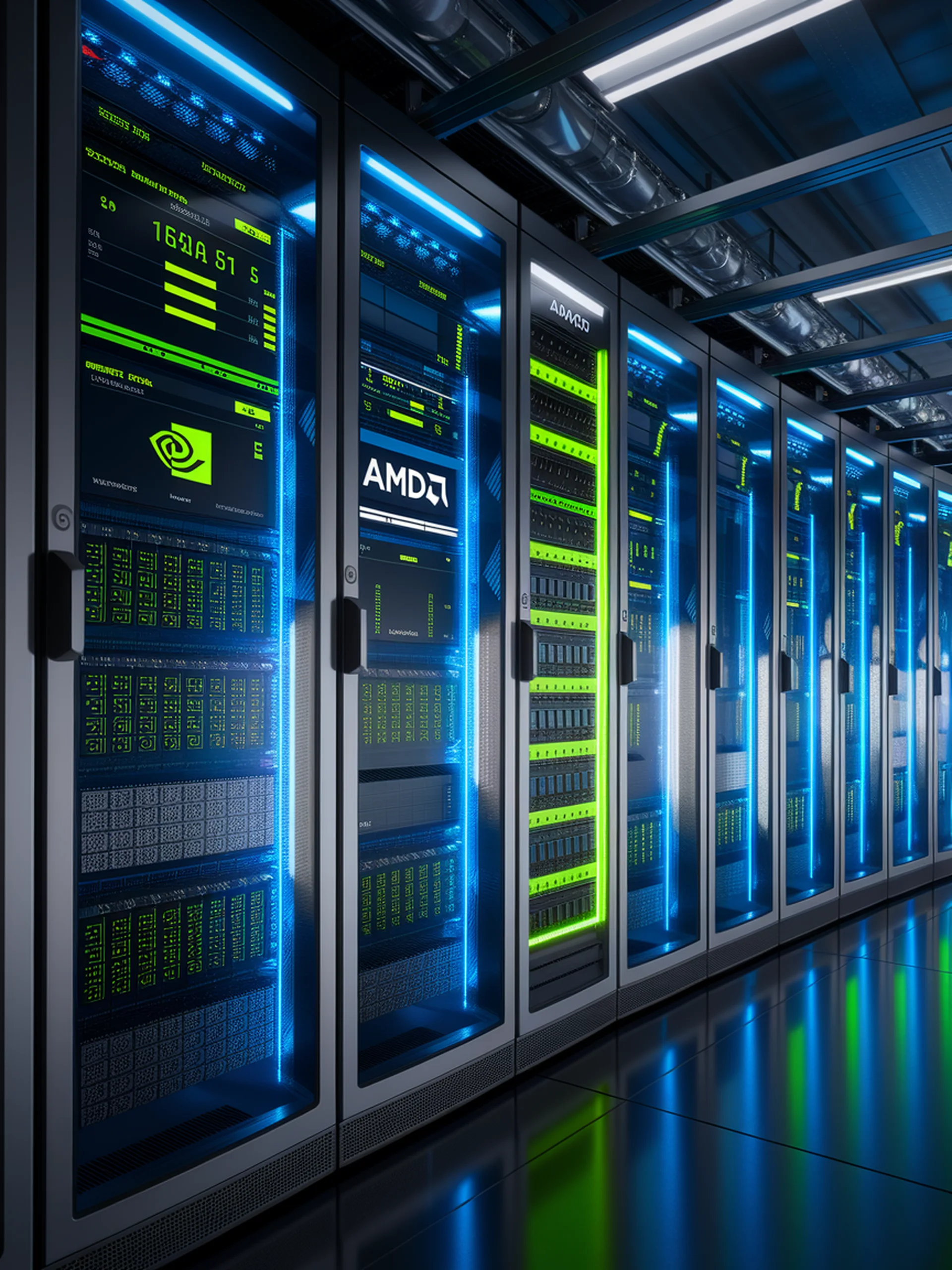
Optimizing LLM Costs with Heterogeneous GPUs
Improving cost-efficiency by matching LLM requests with appropriate GPU resources
This research presents a comprehensive approach to reduce costs when serving Large Language Models by leveraging heterogeneous GPU resources instead of one-size-fits-all solutions.
- Resource-Request Alignment: Different GPU types offer distinct performance-cost tradeoffs for various LLM requests
- Cost Optimization: Properly matching LLM requests to appropriate GPU hardware significantly improves cost-efficiency
- Cloud Deployment: Framework specifically designed for modern cloud platforms with diverse GPU options
- Resource Utilization: Addresses the growing challenge of efficiently handling diverse LLM requests with varying resource demands
This engineering advance matters as organizations seek to scale LLM deployments while controlling infrastructure costs, potentially enabling more cost-effective AI implementation across industries.
Original Paper: Demystifying Cost-Efficiency in LLM Serving over Heterogeneous GPUs