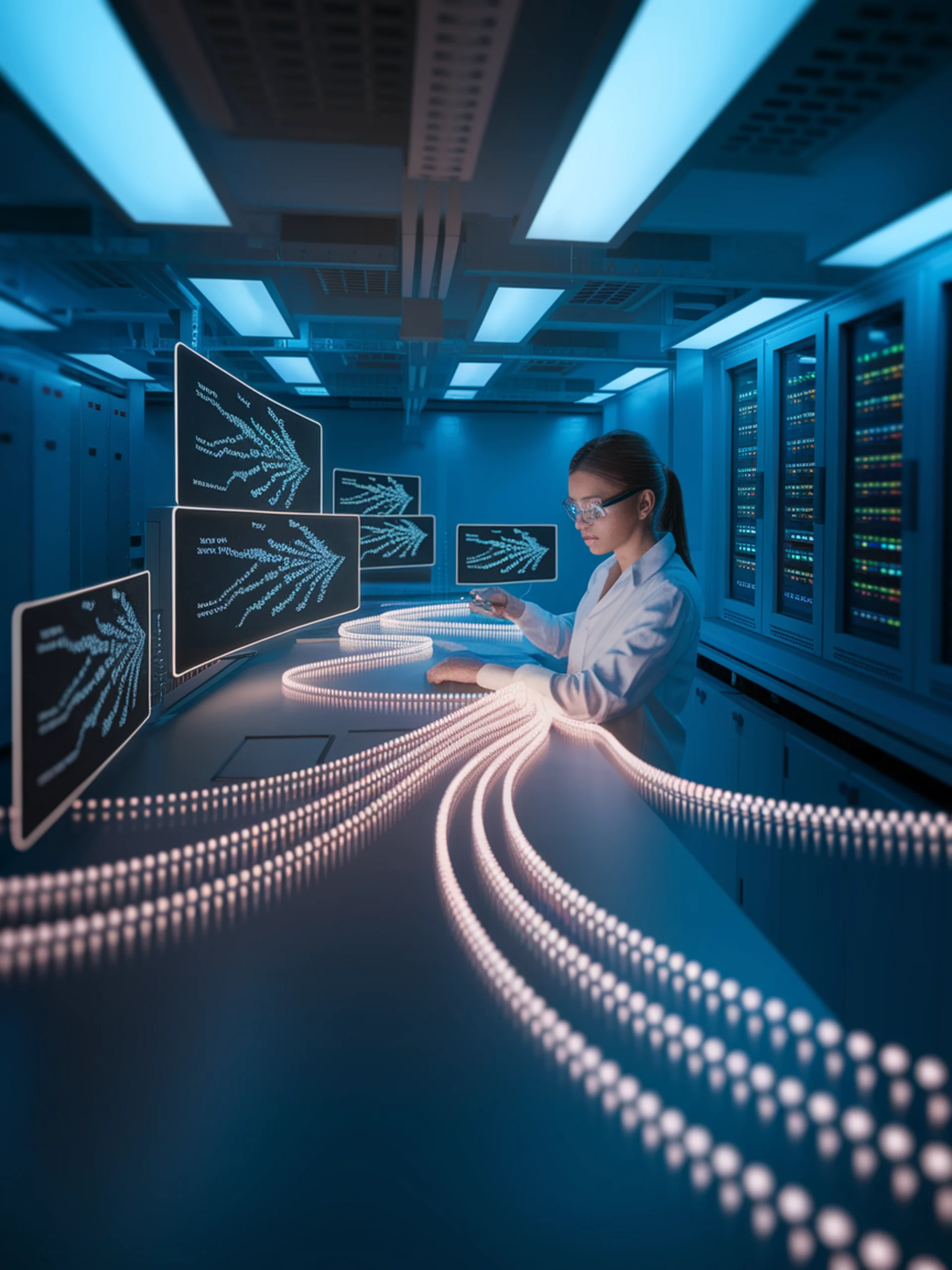
Rethinking LLM Scaling: Beyond Model Size
A probabilistic approach to inference-time optimization using Monte Carlo methods
This research introduces a novel probabilistic framework for scaling LLMs at inference time rather than increasing model size, addressing diminishing returns from traditional scaling approaches.
- Frames LLM output generation as a probabilistic inference problem rather than a search problem
- Utilizes particle-based Monte Carlo methods to explore multiple inference paths efficiently
- Reduces vulnerability to reward hacking common in existing inference-time scaling approaches
- Demonstrates a more computationally efficient approach to improving LLM performance
Engineering significance: This work opens new pathways for enhancing LLM capabilities without requiring larger models or more training data, potentially making advanced AI more accessible and sustainable for practical applications.