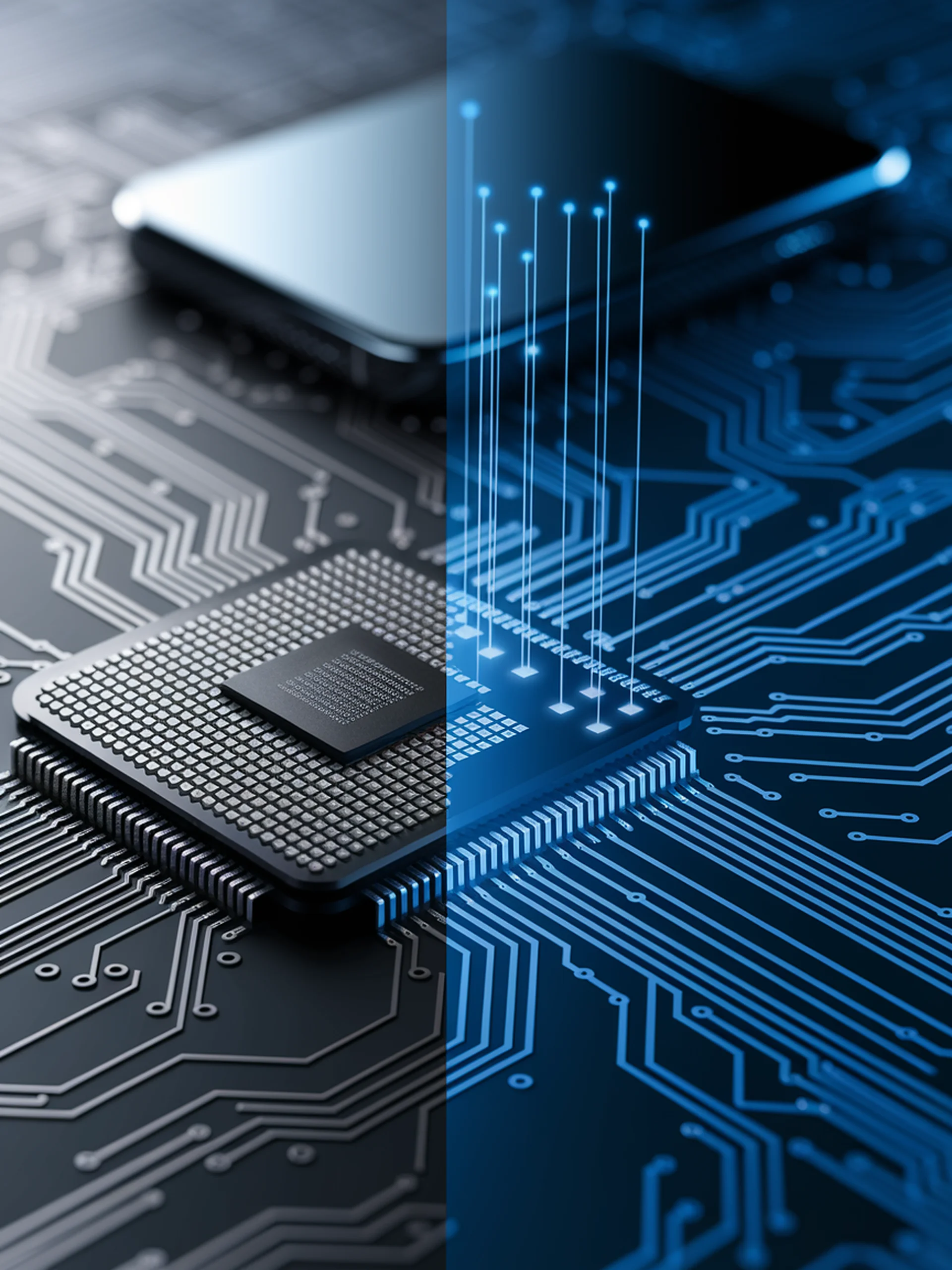
Optimizing LLMs for Resource-Constrained Devices
A novel approach combining binarization with semi-structured pruning
This research introduces a progressive compression technique for large language models that dramatically reduces computational and memory requirements while preserving performance.
- Converts model weights to just 1-bit representation through binarization
- Applies semi-structured pruning to further eliminate redundancy
- Achieves significant memory footprint reduction making LLMs deployable on edge devices
- Demonstrates a balanced approach between model size and performance
This engineering breakthrough enables deployment of powerful language models on resource-constrained environments like mobile phones and IoT devices, potentially democratizing access to AI capabilities across diverse hardware conditions.
Progressive Binarization with Semi-Structured Pruning for LLMs