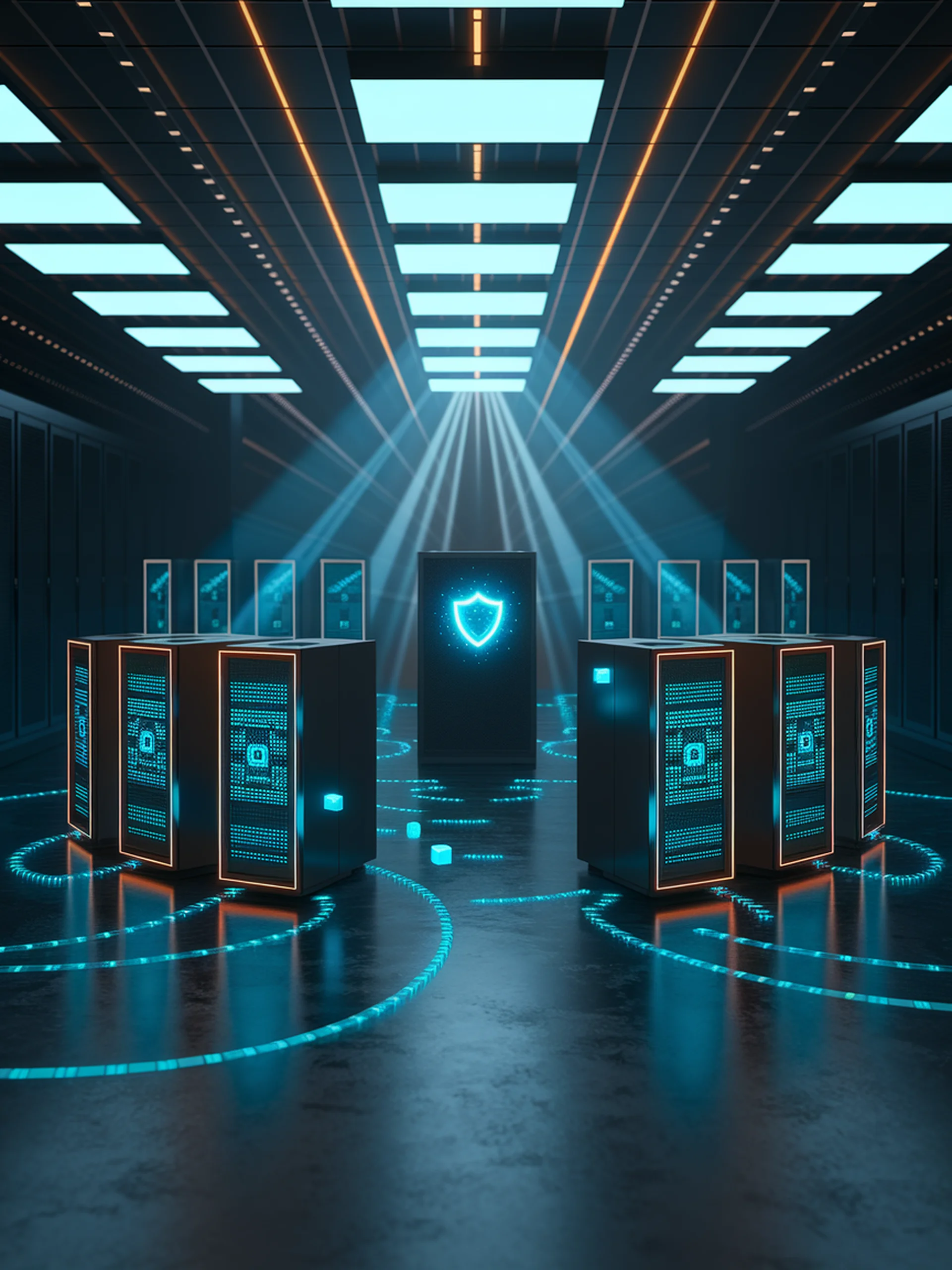
RoLoRA: Making Federated LLM Fine-tuning More Robust
Alternating optimization approach for secure collaborative model training
RoLoRA introduces an innovative framework for robust federated fine-tuning of large language models using Low-Rank Adaptation (LoRA) techniques to reduce computational costs while preserving security.
- Employs alternating optimization between up and down projection matrices to enhance expressiveness
- Significantly improves robustness in distributed training environments
- Reduces communication costs while maintaining model performance
- Provides theoretical foundations and experimental validation for secure collaborative training
This research is critical for security professionals as it enables private, secure model training across distributed entities without sacrificing performance, addressing key vulnerabilities in federated learning environments.
Robust Federated Finetuning of LLMs via Alternating Optimization of LoRA