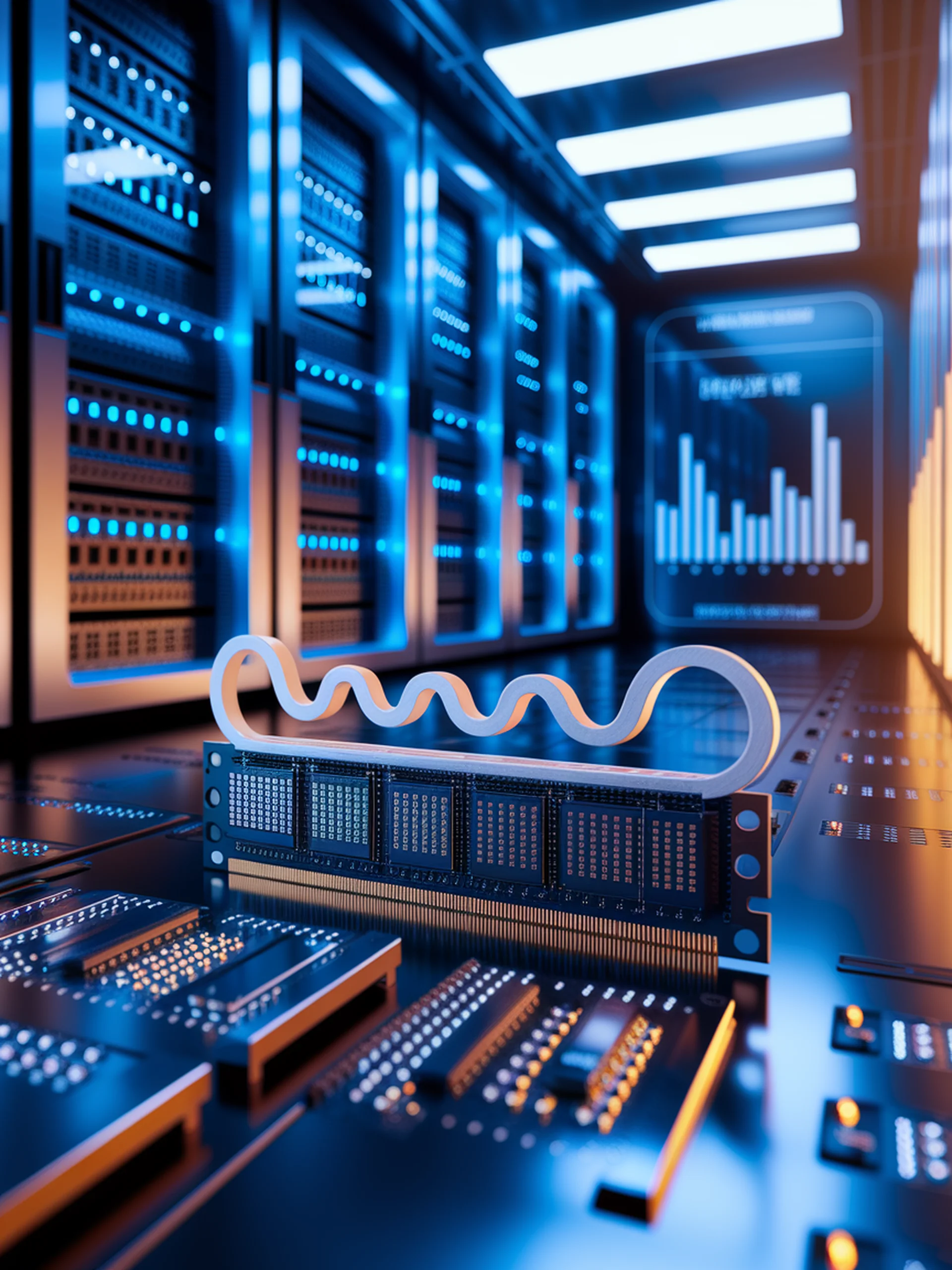
Optimizing Memory for LLMs
PolarQuant: A Novel Approach to KV Cache Compression
PolarQuant introduces an innovative quantization technique that transforms KV embeddings into polar coordinates, significantly reducing memory requirements for large language models.
- Uses random preconditioning and polar transformation to compress KV caches efficiently
- Employs a recursive algorithm to transform embeddings into polar coordinates before quantization
- Addresses a critical bottleneck in LLM deployment: memory consumption when handling long-range contexts
- Represents an engineering breakthrough for more efficient LLM operation
This research matters because memory constraints remain a significant barrier to LLM deployment in resource-limited environments. PolarQuant offers a practical solution for engineers looking to optimize LLM performance without sacrificing quality.