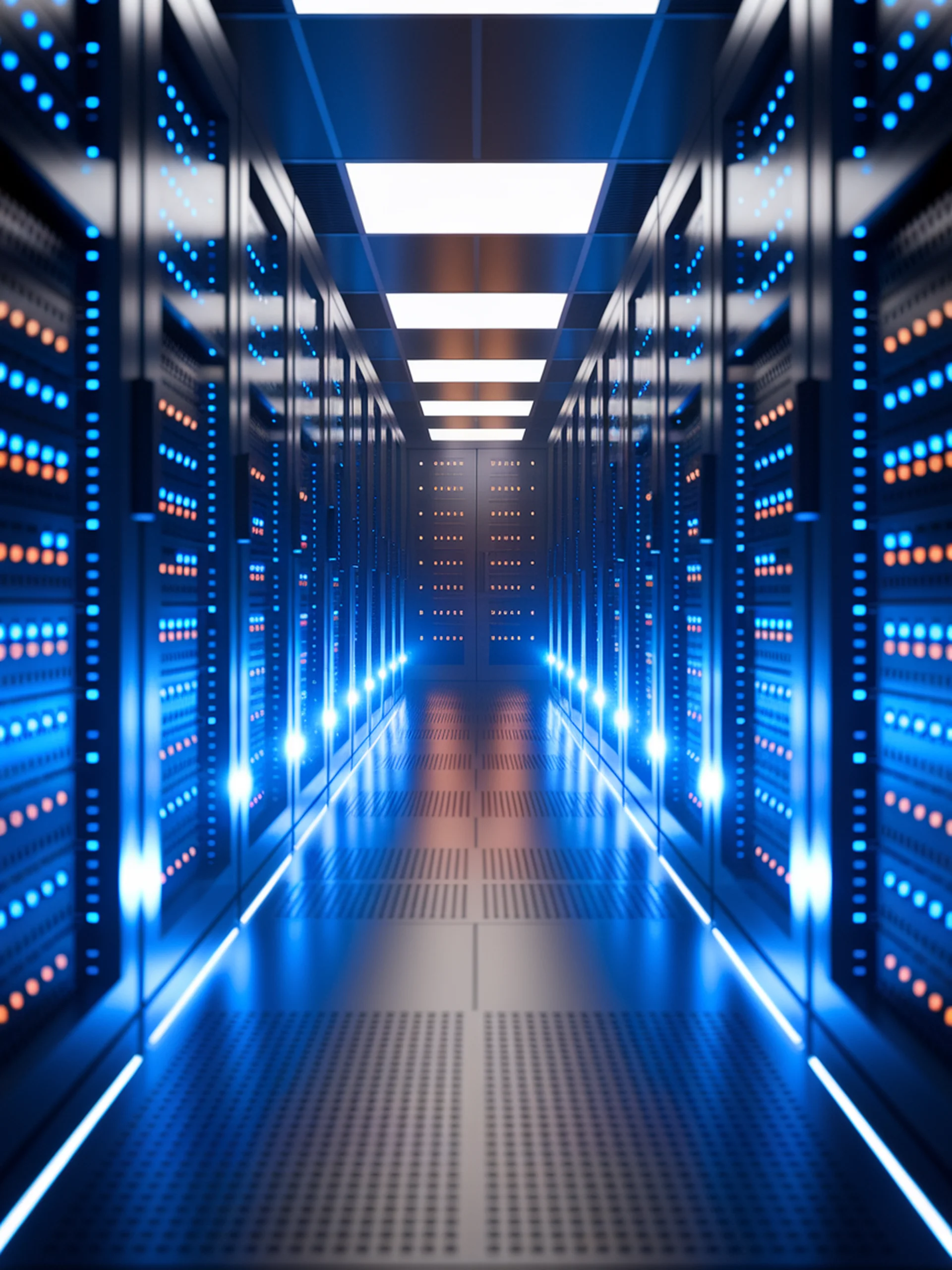
Adaptive Attention Sparsity for LLMs
Dynamic efficiency for long-context language models
Twilight introduces an adaptive approach to attention sparsity that dynamically balances accuracy and efficiency in LLMs.
- Implements hierarchical top-p pruning that adapts to varying computational budgets
- Achieves up to 2.2× speedup while maintaining model performance
- Automatically adjusts sparsity patterns based on input context requirements
- Outperforms fixed-budget approaches in real-world deployment scenarios
This innovation enables more efficient processing of long-context inputs, making advanced LLMs more practical for production environments with varying computational resources.
Twilight: Adaptive Attention Sparsity with Hierarchical Top-$p$ Pruning