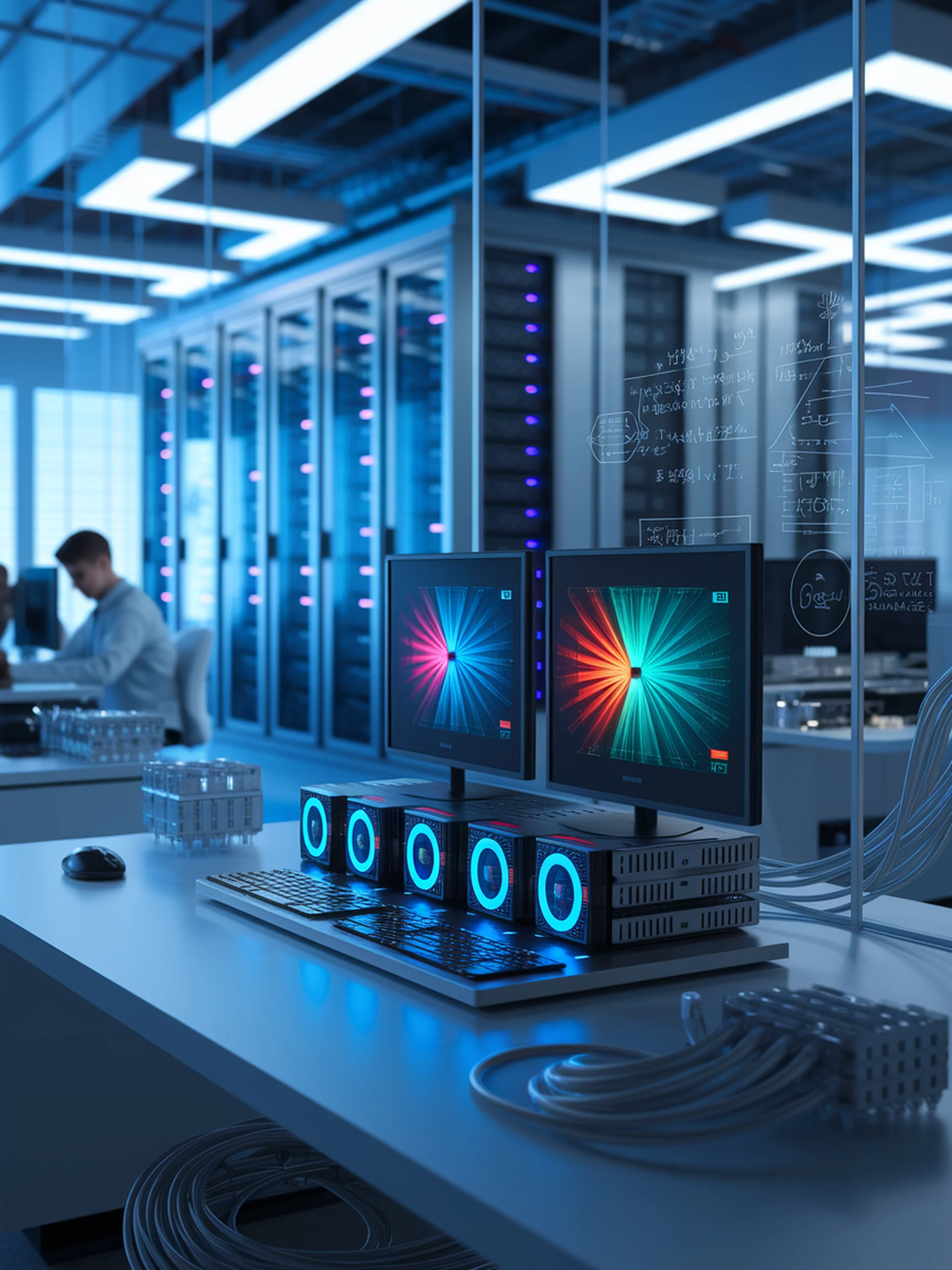
Memory-Efficient LLM Fine-Tuning Breakthrough
Accelerating Zero-Order Optimization for Real-World Deployment
DiZO introduces a novel approach to fine-tune large language models with minimal memory requirements while maintaining high performance.
- 3-10× faster convergence than previous zeroth-order methods
- Comparable accuracy to standard first-order fine-tuning techniques
- Significantly reduced memory footprint enabling deployment in resource-constrained environments
- Practical implementation with proven effectiveness for real-world LLM adaptation
This engineering advance addresses a critical bottleneck in LLM deployment, allowing organizations to efficiently customize models without massive computational resources. The technique provides particular value for applications with limited GPU memory or where rapid adaptation is required.
Harmony in Divergence: Towards Fast, Accurate, and Memory-efficient Zeroth-order LLM Fine-tuning