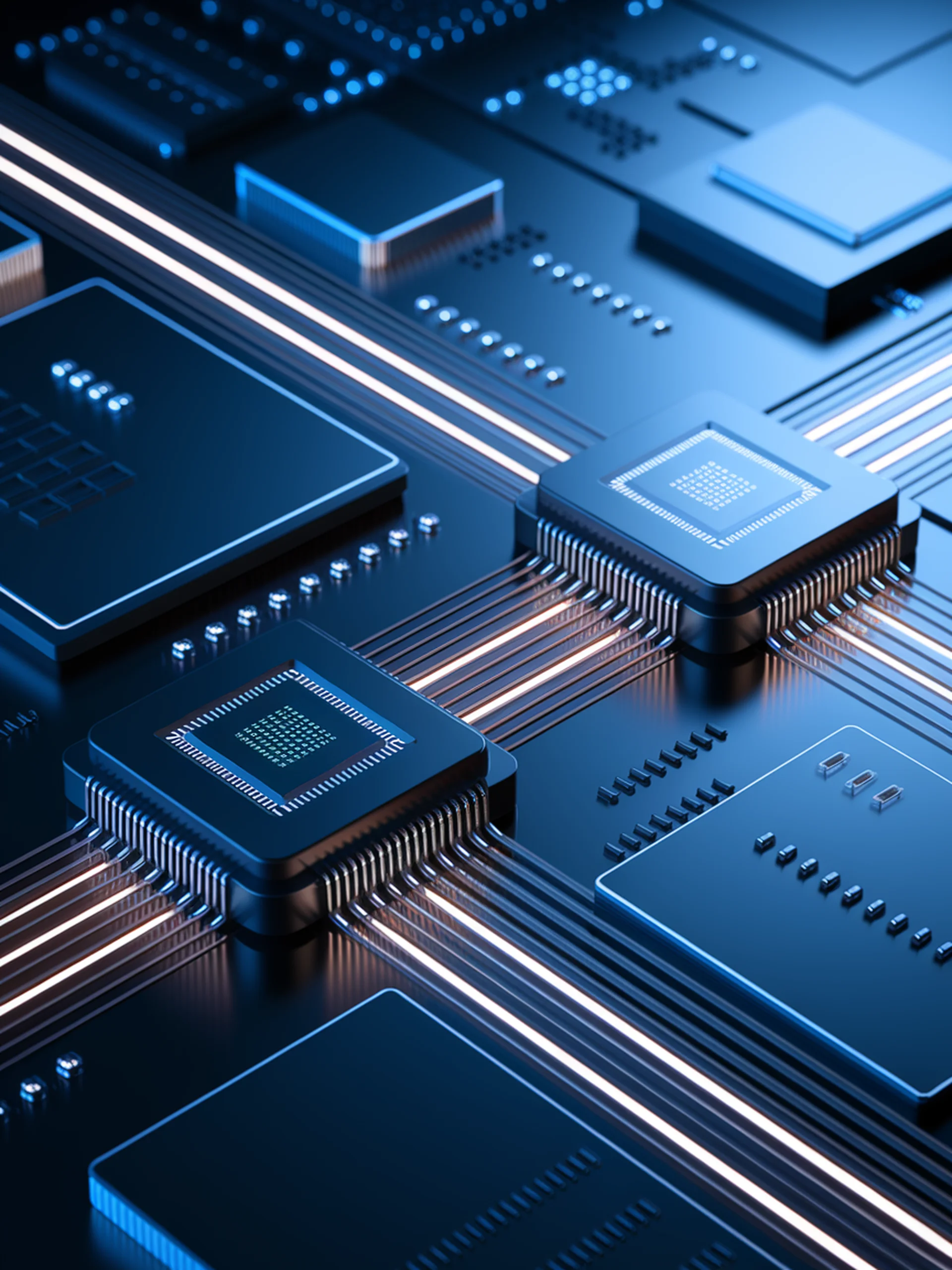
Breaking Vocabulary Barriers in LLM Inference
New speculative decoding algorithms accelerate LLMs without vocabulary constraints
This research introduces lossless speculative decoding methods that eliminate vocabulary matching requirements between drafter and target models, significantly enhancing LLM inference speed.
- Removes the constraint that drafter and target models must share the same vocabulary
- Enables using a wider range of existing pre-trained models as drafters
- Eliminates the need to train drafters from scratch
- Maintains the quality of outputs while improving inference efficiency
For engineering teams, these algorithms provide practical solutions to a critical bottleneck in generative AI deployment, allowing more flexible and cost-effective acceleration of LLM inference in production environments.