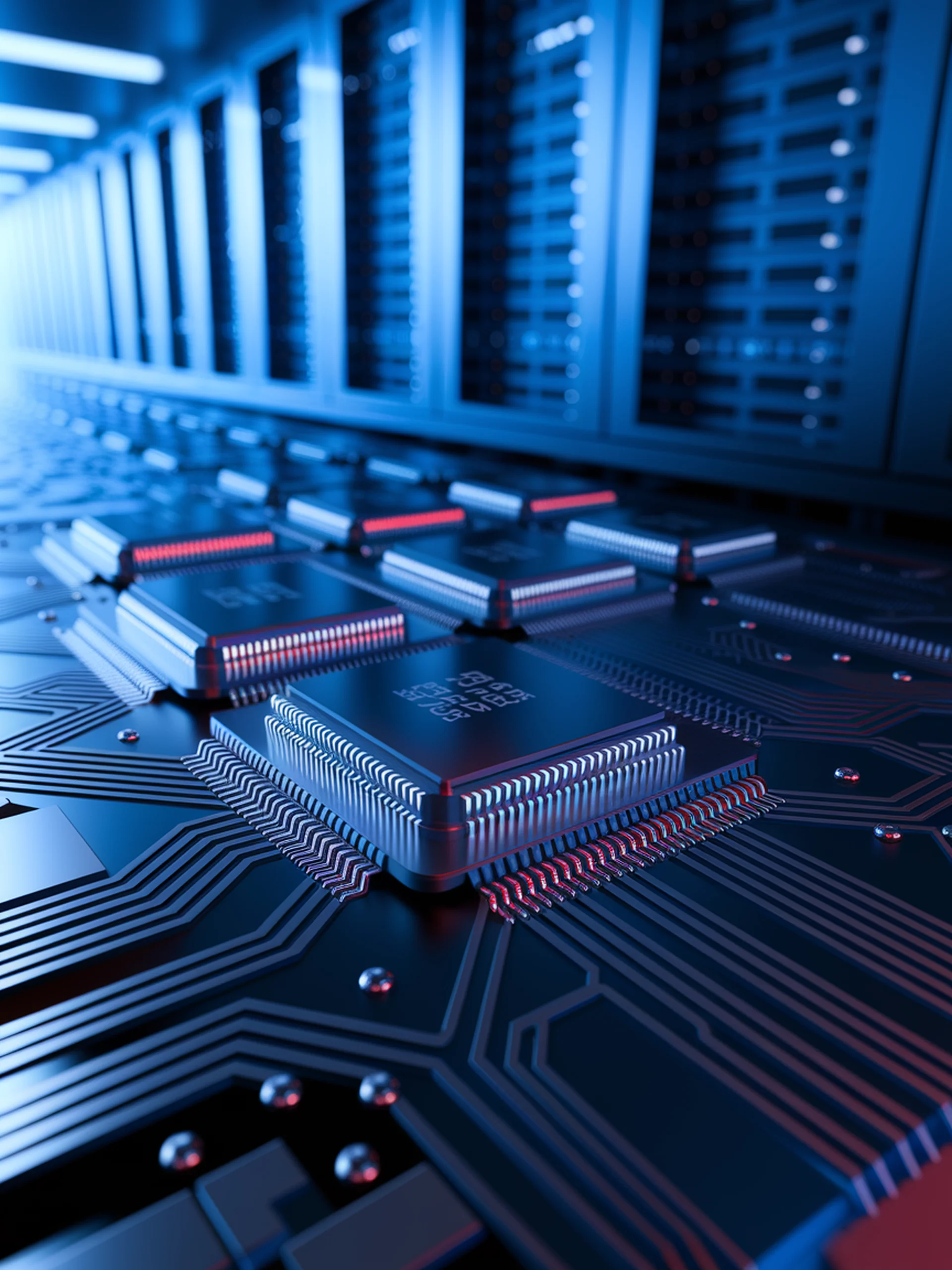
4-bit LLM Inference Breakthrough
Enabling Ultra Low-Precision Models Without Retraining
Block Clustered Quantization (BCQ) is a novel technique that enables accurate 4-bit LLM inference without expensive fine-tuning or quantization-aware training.
- Achieves W4A4 quantization (4-bit weights and activations) without accuracy degradation
- Leverages block-level clustering to optimize quantization parameters across different model regions
- Minimizes computational and memory requirements while preserving model performance
- Provides a practical path to deploying efficient LLMs on resource-constrained hardware
This research represents a significant engineering advancement for deploying large language models in edge computing, mobile applications, and other scenarios with limited computational resources.
BCQ: Block Clustered Quantization for 4-bit (W4A4) LLM Inference