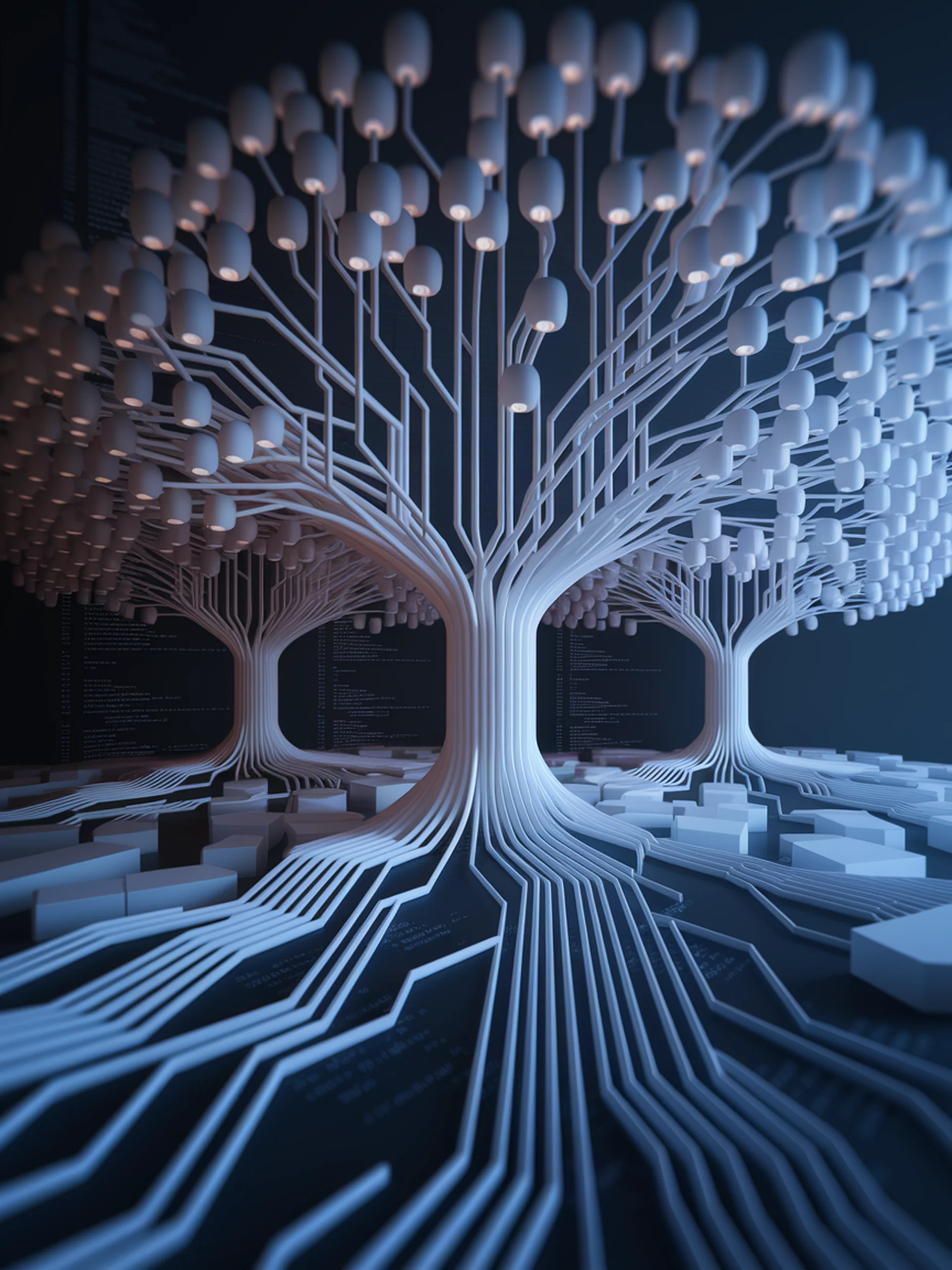
Speeding Up LLMs with Dynamic Tree Attention
A smarter approach to parallel token prediction
This research introduces a dynamic tree attention approach that significantly improves inference speed for large language models without sacrificing quality.
- Replaces fixed tree structures with adaptive prediction paths based on token probabilities
- Achieves faster inference by predicting multiple tokens simultaneously
- Reduces computational complexity through intelligent candidate selection
- Demonstrates practical engineering improvements for real-world LLM deployment
This innovation matters for engineering teams building LLM applications where response time is critical, offering a path to more efficient, responsive AI systems.
Original Paper: Acceleration Multiple Heads Decoding for LLM via Dynamic Tree Attention