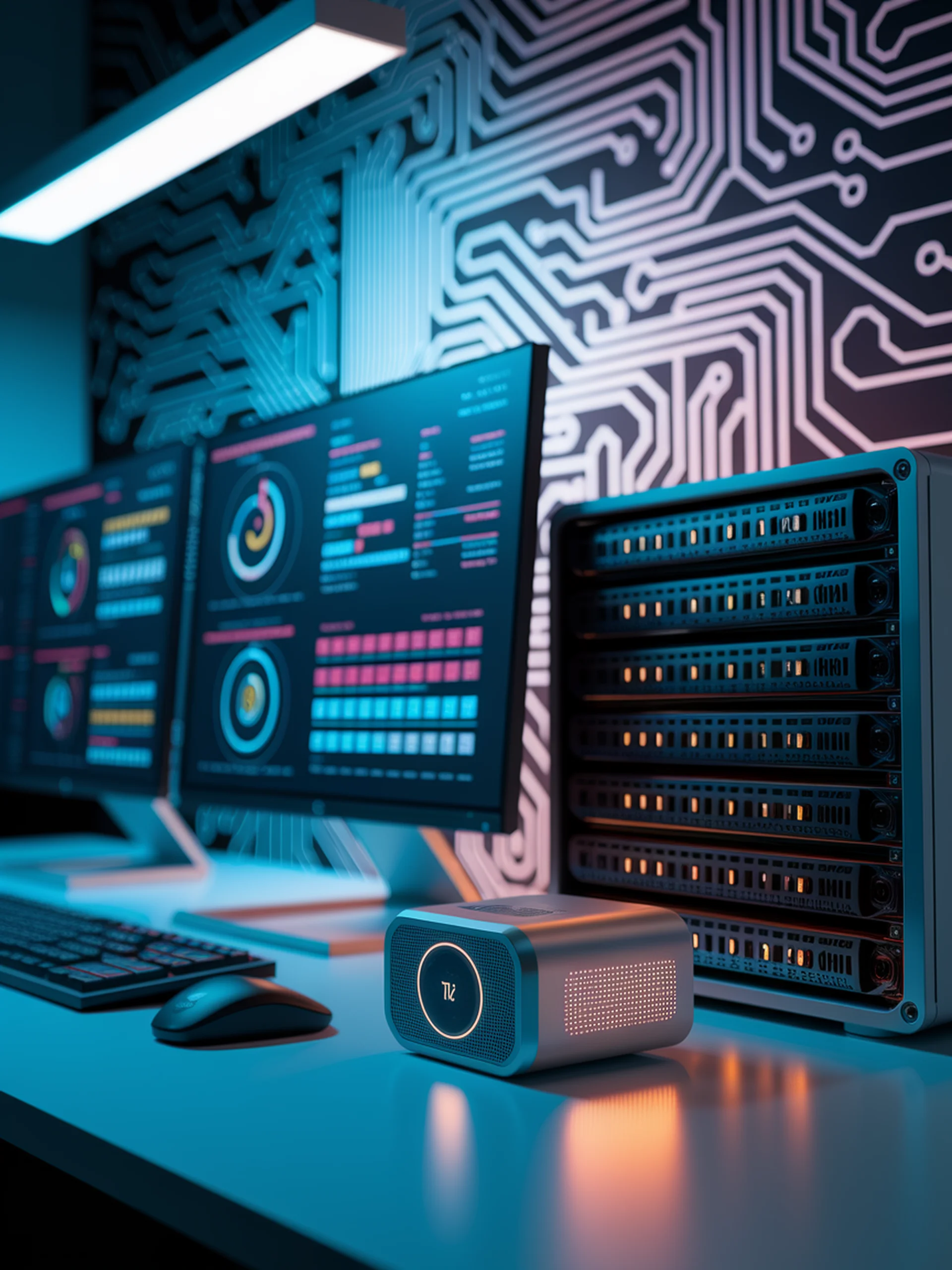
Streamlining LLM Deployment with RoSTE
A unified approach to quantization and fine-tuning
RoSTE introduces an efficient quantization-aware supervised fine-tuning approach that optimizes LLMs for deployment while maintaining performance.
- Combines fine-tuning and quantization in a single unified process rather than as separate sequential steps
- Achieves superior performance compared to conventional methods that fine-tune first, then quantize
- Enables low-bit quantization of weights, activations, and KV cache for efficient deployment
- Delivers practical efficiency gains for real-world LLM applications
This research matters because it addresses a critical engineering challenge: deploying powerful language models with reduced computational requirements while preserving their capabilities.
RoSTE: An Efficient Quantization-Aware Supervised Fine-Tuning Approach for Large Language Models