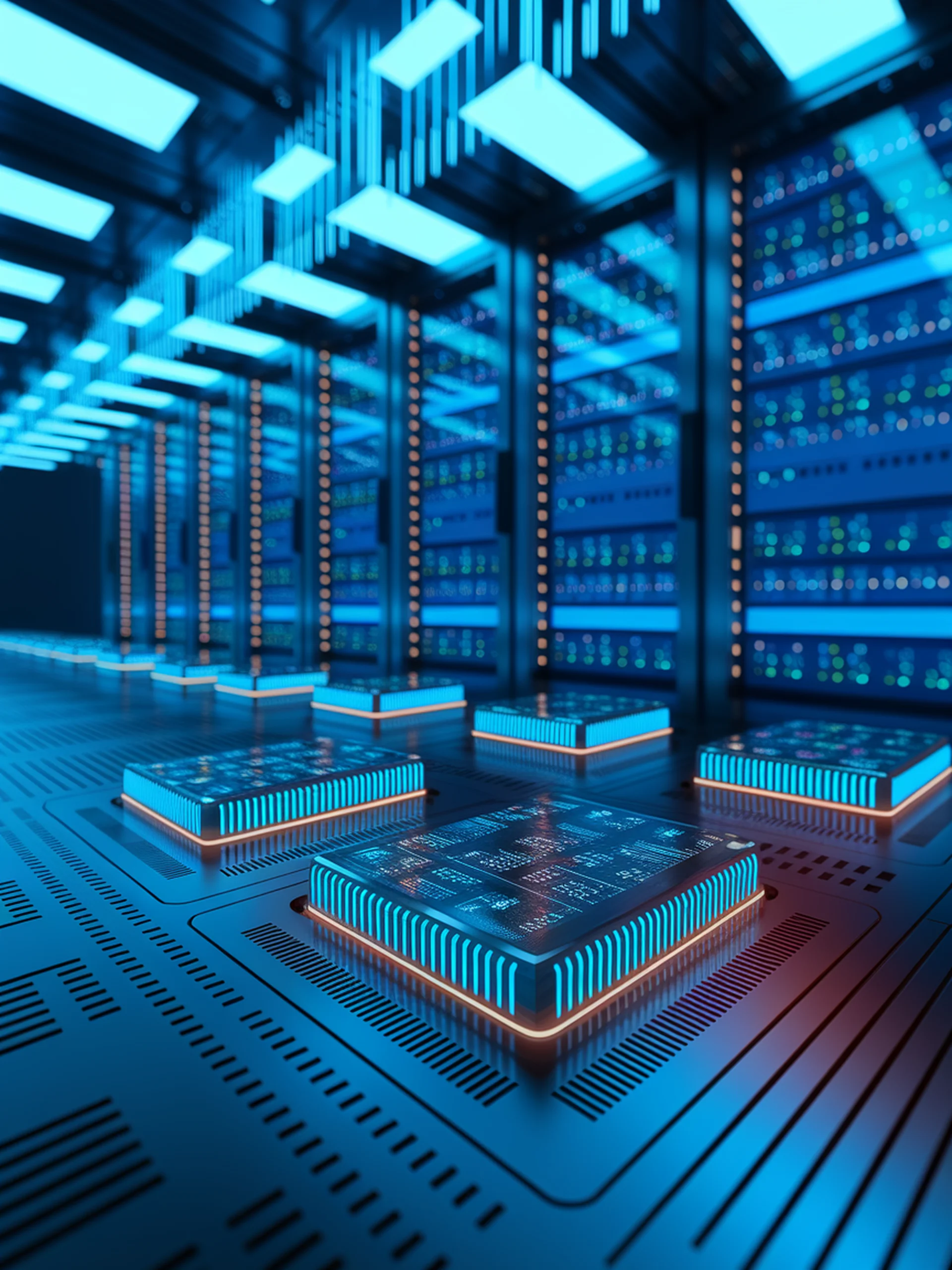
Near-Storage Processing: Supercharging LLM Inference
Boosting throughput for large language model deployment
INF² introduces a novel framework that processes data directly within storage devices to drastically improve LLM inference performance.
- Achieves 5.3-36.9× higher inference throughput compared to conventional offloading methods
- Reduces I/O bottlenecks by processing key-value (KV) cache data at the storage level
- Implements intelligent workload partitioning and dynamic load balancing across storage devices
- Demonstrates practical viability with both simulated and real computational storage device implementations
This research solves a critical engineering challenge for LLM deployment at scale, making large models more accessible and cost-effective for real-world applications without requiring expensive GPU hardware upgrades.
INF²: High-Throughput Generative Inference of Large Language Models using Near-Storage Processing