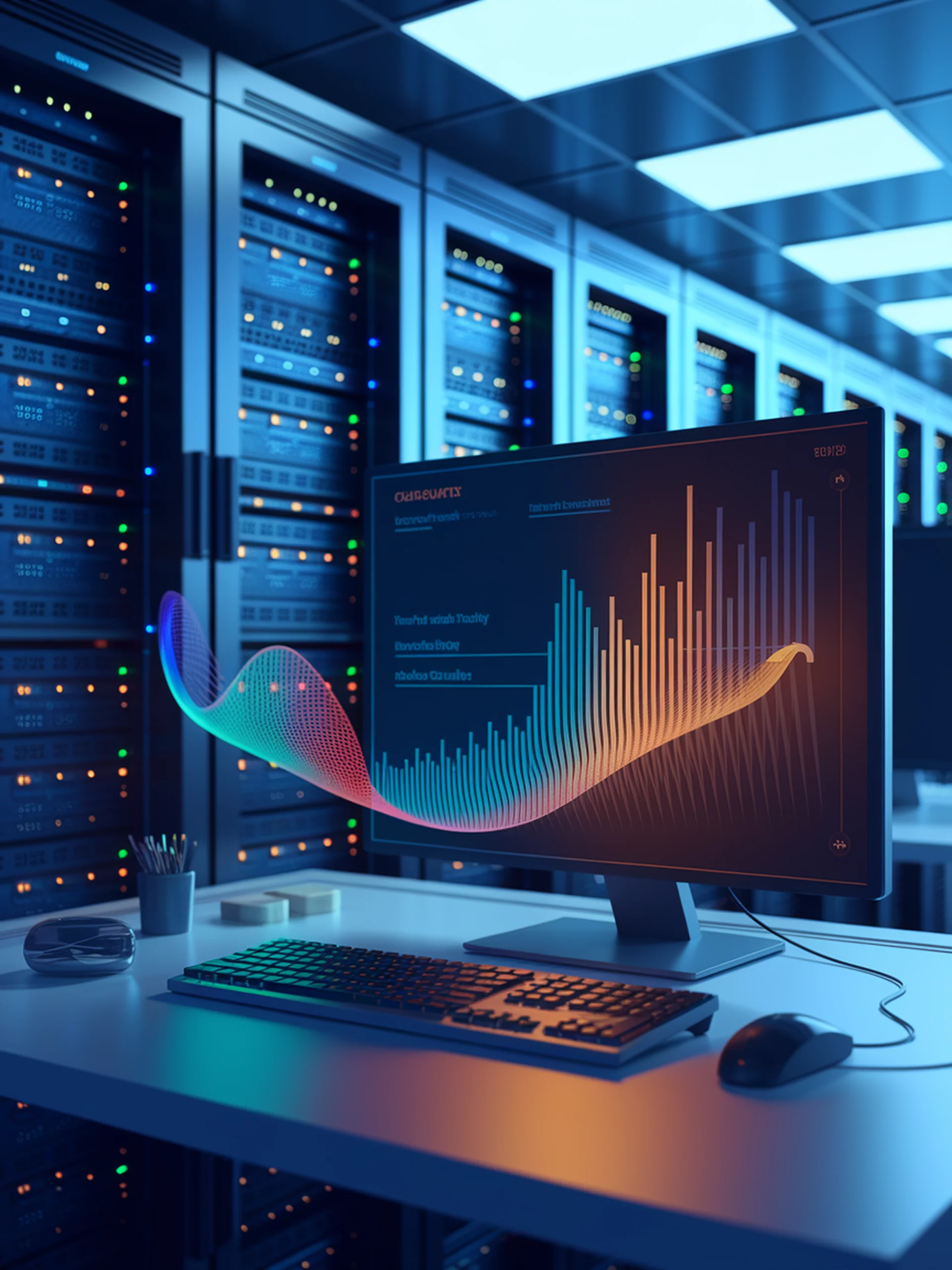
AdaGC: Stabilizing LLM Training
Adaptive gradient clipping for more efficient AI model development
A novel technique that tackles the critical problem of loss spikes during large language model training, enhancing stability and performance.
- Parameter-specific adjustment replaces traditional global gradient clipping with adaptive local thresholds
- Automatic calibration through exponential moving average of gradient norms
- Enhanced convergence with theoretical guarantees and empirical validation
- Improves scalability for engineering teams building increasingly larger AI systems
This innovation directly addresses a major engineering challenge in AI, potentially reducing training costs and improving model quality by preventing destructive gradient updates during the critical pretraining phase.
AdaGC: Improving Training Stability for Large Language Model Pretraining