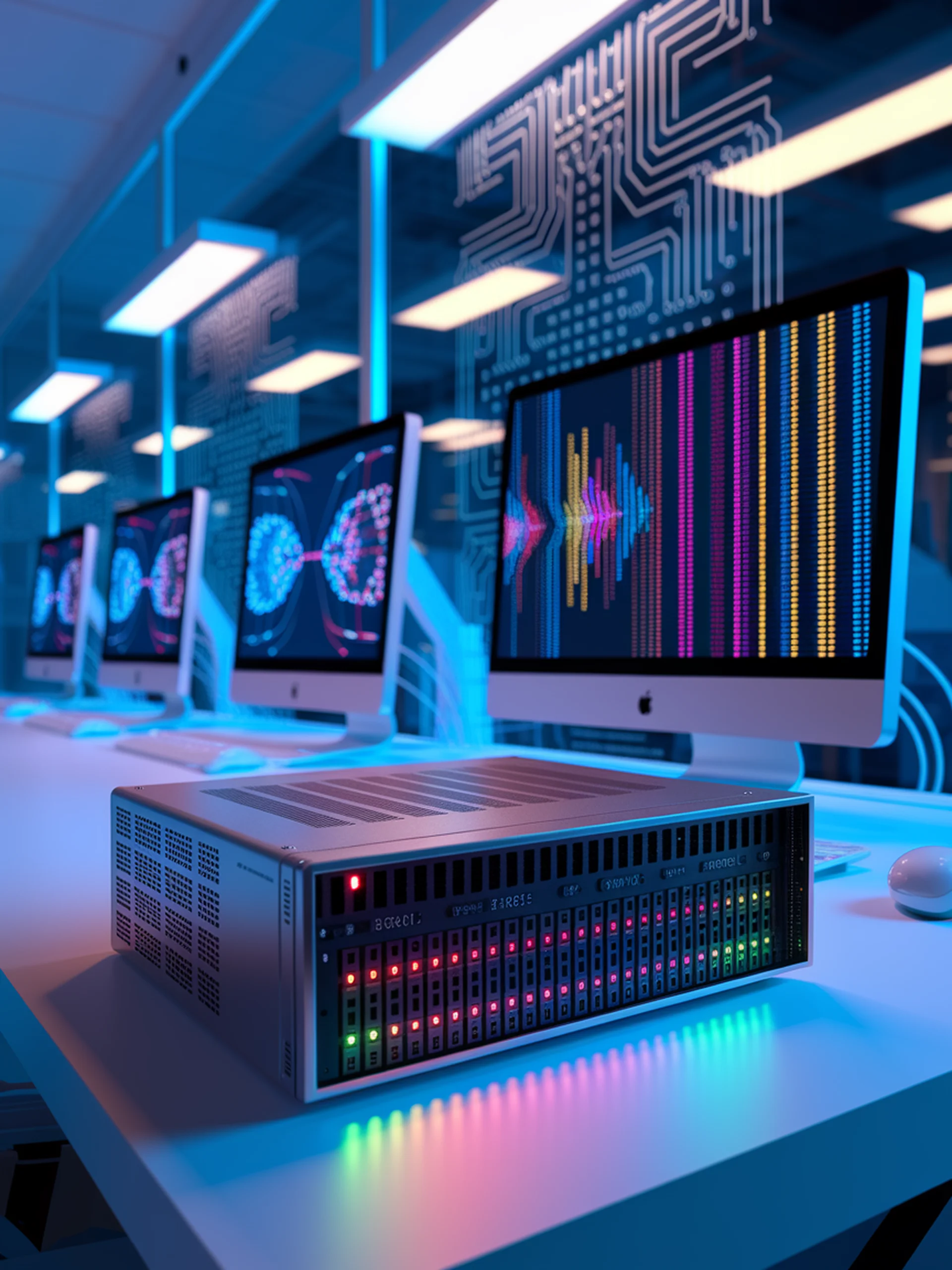
Efficient LLM Fine-Tuning for Resource-Constrained Teams
A Row-Based Sparse Approach to Reduce Memory & Computational Demands
This research introduces a new Sparse Fine-Tuning (SFT) framework that makes adapting foundation models more accessible to teams with limited computational resources.
- Develops a row-based sparse fine-tuning technique that achieves high efficiency
- Builds upon existing SFT and Low-rank adaptation (LoRA) approaches
- Reduces memory and computational requirements while maintaining model performance
- Enables broader adoption of fine-tuning for specialized applications
For engineering teams, this advancement means more efficient model customization with fewer resources, potentially democratizing access to state-of-the-art LLM adaptations.