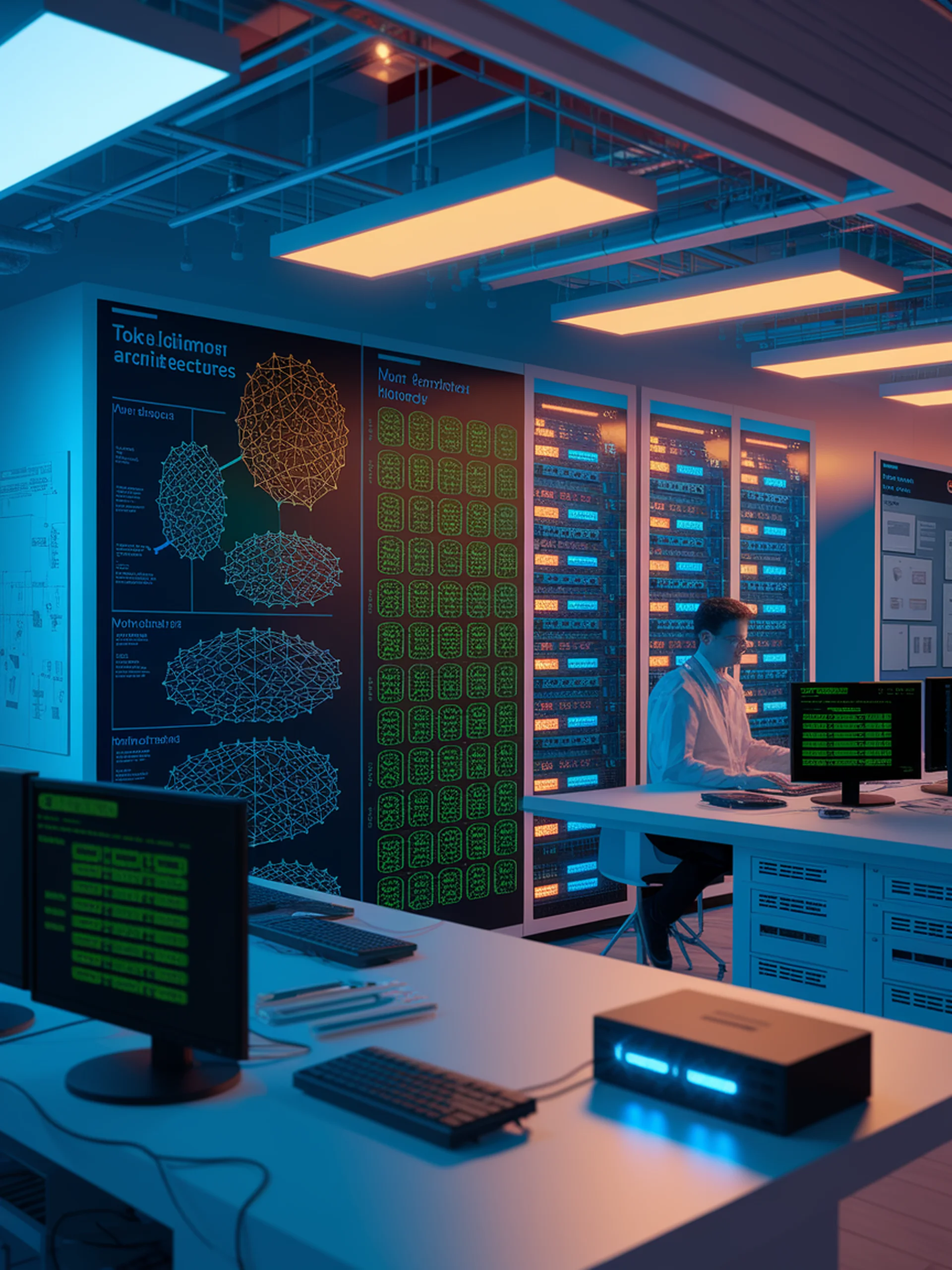
Rethinking Token Pruning in Multimodal LLMs
Why token importance may be the wrong focus for efficiency
This research challenges conventional wisdom about token pruning in multimodal language models, revealing that token duplication has greater impact than token importance.
Key Findings:
- Vision tokens create significant computational overhead compared to linguistic tokens
- Traditional pruning methods that focus on token importance may be suboptimal
- Token duplication emerges as a more critical factor in determining which tokens to prune
- This insight provides a new direction for making multimodal language models more computationally efficient
Engineering Impact: This research offers AI engineers a fundamentally different approach to optimizing multimodal models, potentially reducing computational requirements while maintaining performance in vision-language systems.
Stop Looking for Important Tokens in Multimodal Language Models: Duplication Matters More