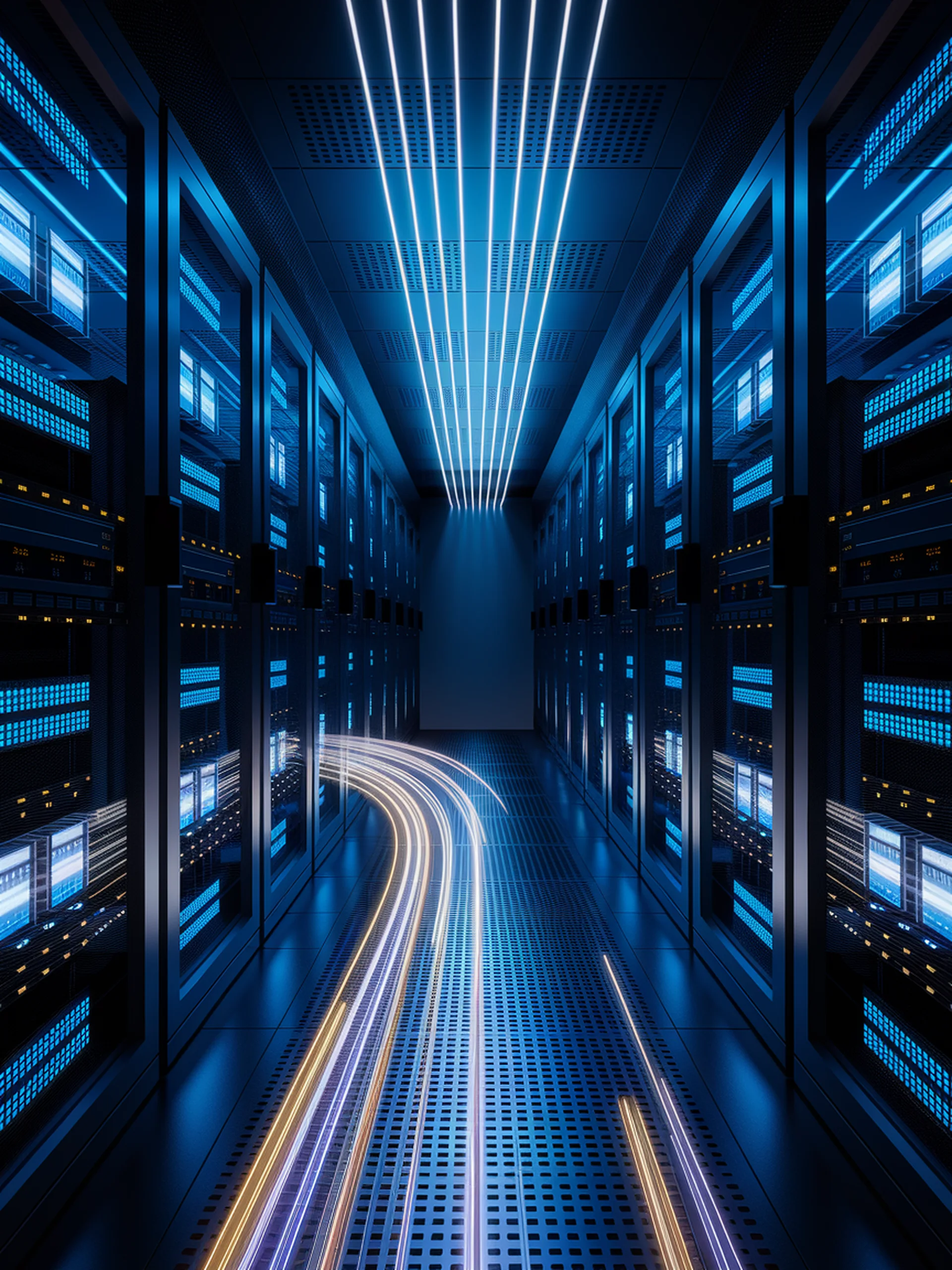
Breaking the Long-Context Bottleneck
Accelerating LLM inference by optimizing context block compression across GPUs
APB introduces a novel approach to distributed inference that significantly improves processing speed for long-context prompts in large language models.
- Addresses the critical prefill bottleneck in LLM inference by intelligently compressing and distributing context across multiple GPUs
- Achieves up to 4.5x speedup compared to existing sequence parallelism approaches
- Maintains high accuracy while reducing computational overhead through optimized attention mechanisms
- Enables practical deployment of truly long-context applications with responsive performance
This research matters because it makes long-context LLM applications viable for real-time business use cases, reducing latency without sacrificing quality.