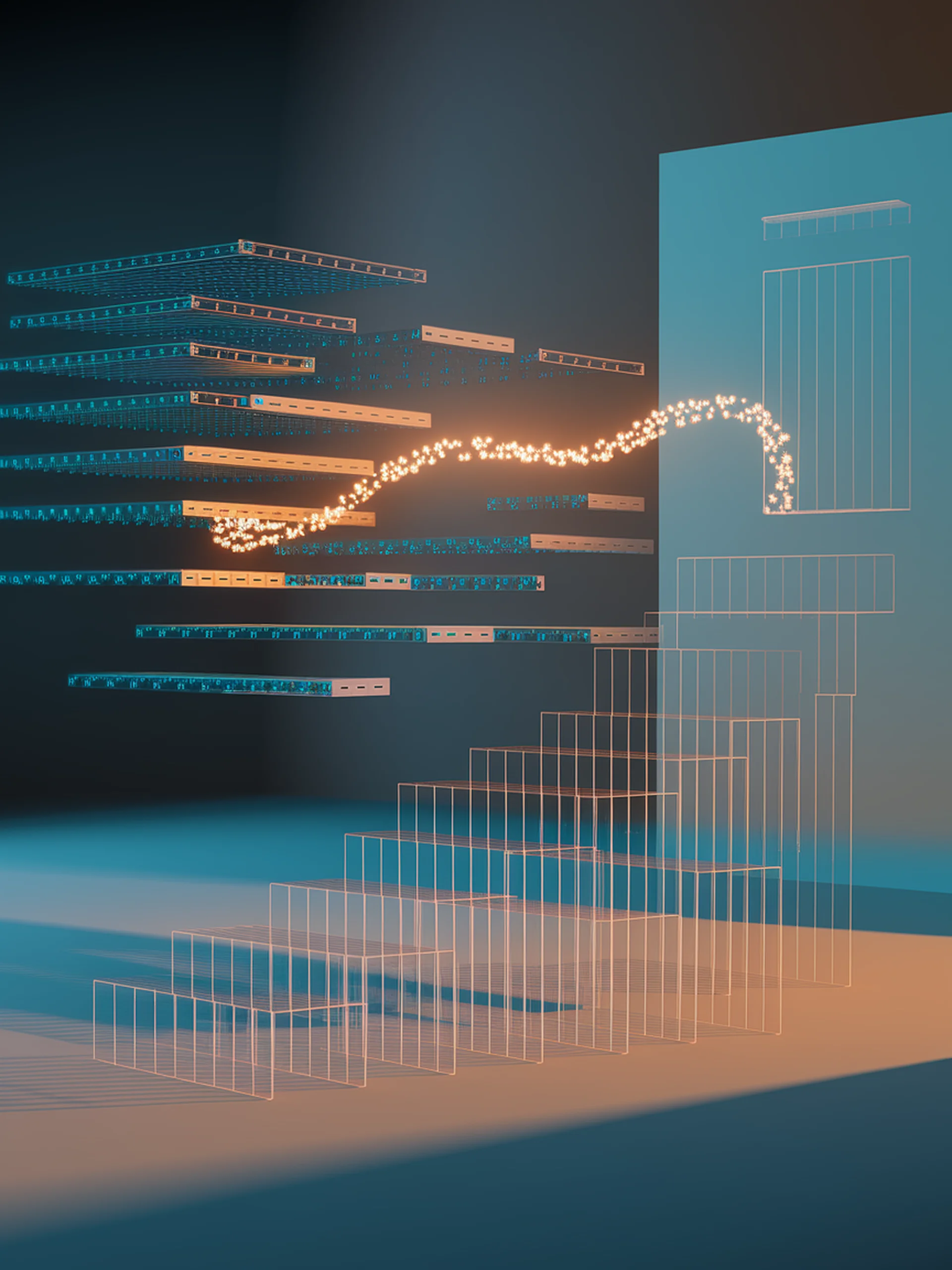
Adaptive Sparse Attention for Long-Context LLMs
Dynamic token selection that adapts to content importance
Tactic introduces a novel approach to sparse attention that dynamically adapts to varying importance across different attention heads, layers, and contexts.
- Eliminates fixed token budgets by using clustering and distribution fitting to determine optimal sparsity patterns
- Provides a calibration-free solution that automatically adjusts to content importance
- Achieves better performance while reducing the computational load of KV caches
- Maintains high accuracy for long-context applications without manual tuning
This engineering breakthrough enables more efficient deployment of long-context LLMs in practical applications, reducing computational costs while preserving model quality for complex tasks.
Tactic: Adaptive Sparse Attention with Clustering and Distribution Fitting for Long-Context LLMs