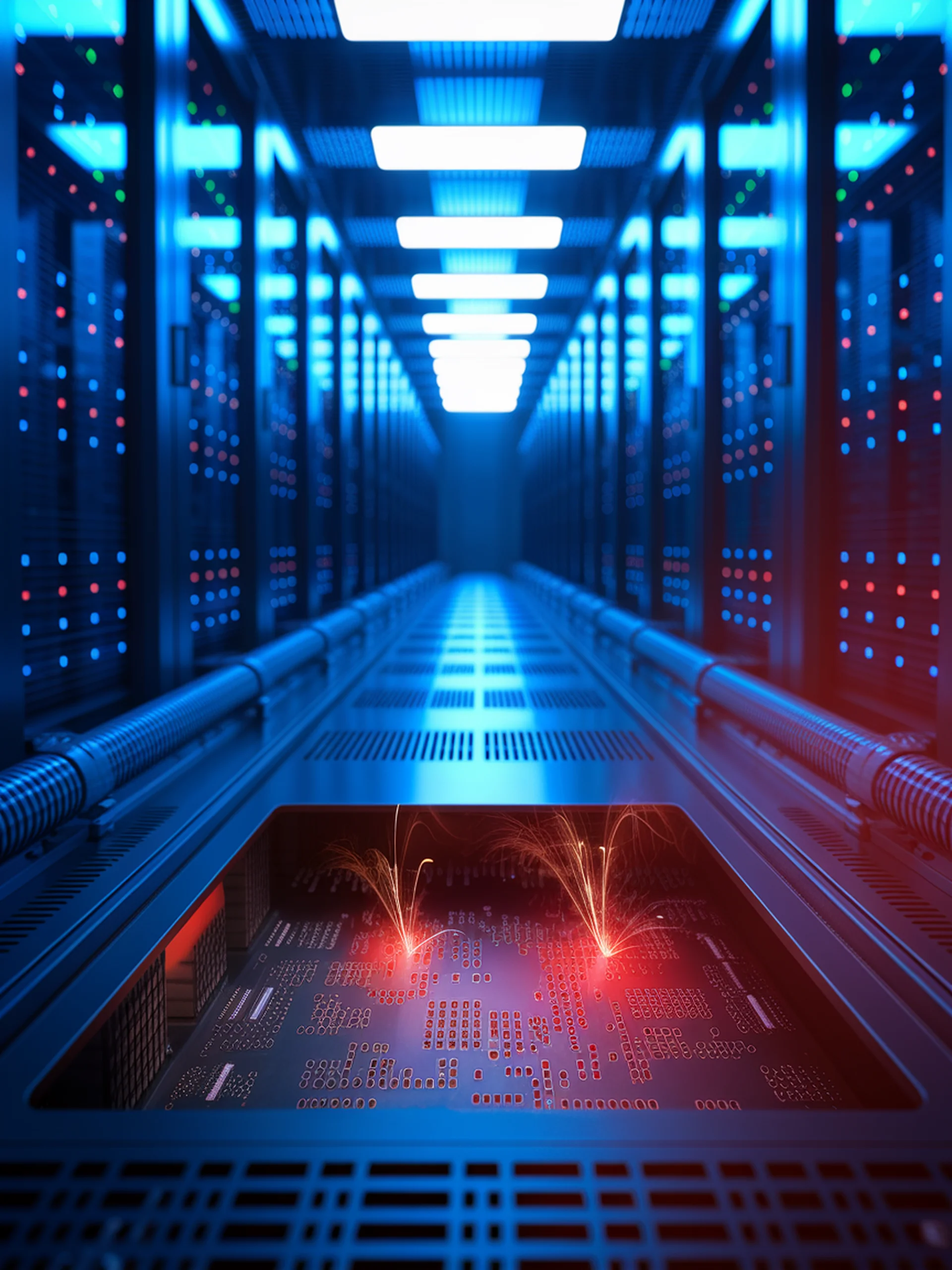
When Hardware Lies: Silent Data Corruption in LLM Training
First comprehensive analysis of hardware-induced corruption effects on large language models
This groundbreaking research identifies and analyzes the impact of Silent Data Corruption (SDC) during LLM training, where hardware produces incorrect computations without triggering failure signals.
Key Findings:
- Researchers compared model training between healthy nodes and nodes exhibiting SDCs in real production environments
- SDCs can significantly degrade model performance without obvious warning signs
- Hardware-level failures introduce unique challenges in massive-scale LLM training
- The findings enable more robust training infrastructure design
Engineering Significance: As LLM training scales continue to increase, understanding hardware failure modes becomes critical for maintaining training integrity and ensuring reliable model performance. This research provides essential insights for building more resilient AI infrastructure.