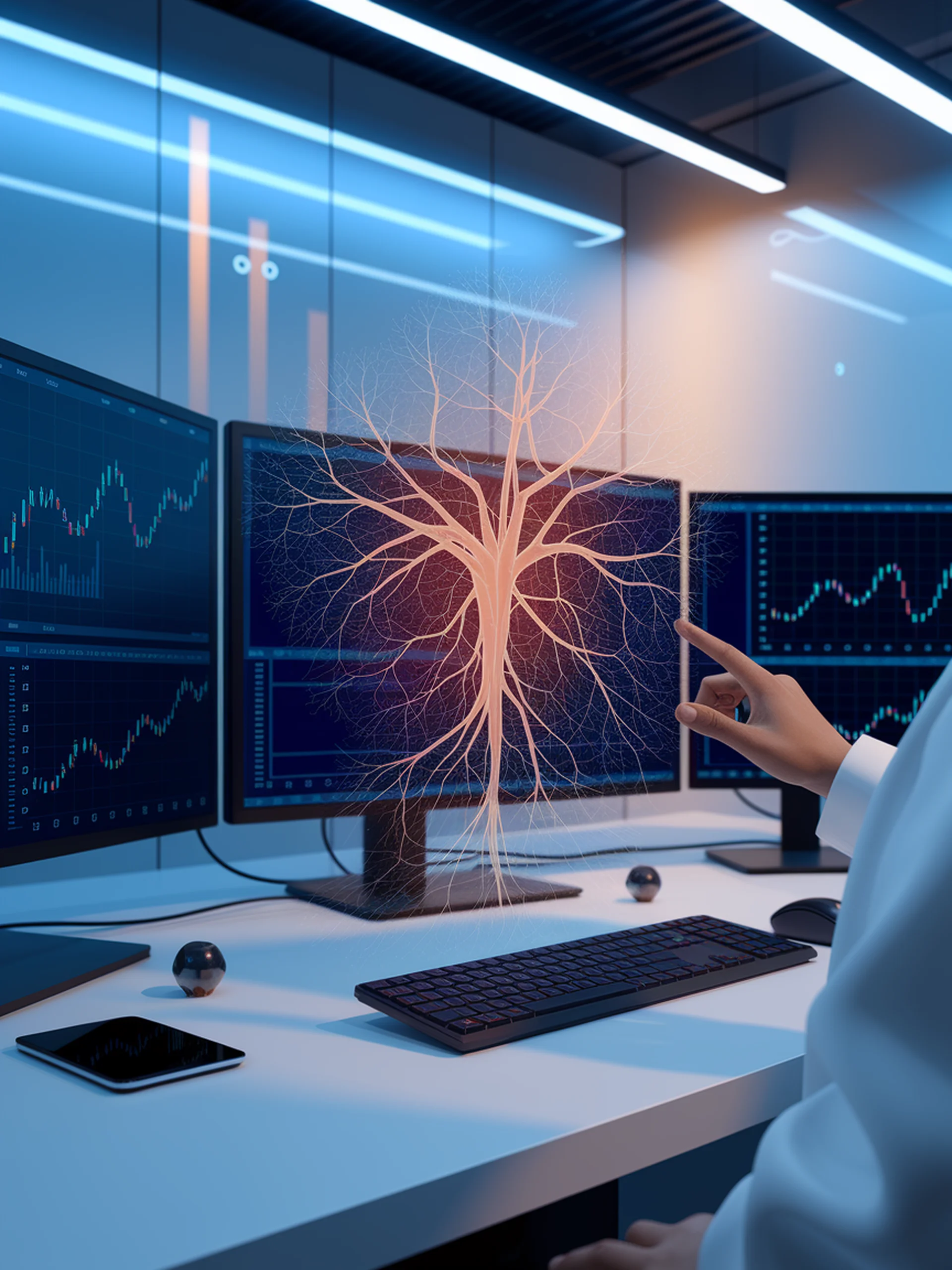
Smarter Recovery for Pruned LLMs
Efficient data selection for recovering pruned language models
PASER introduces a targeted approach to recover pruned language model capabilities with minimal computational cost through selective data use.
- Addresses uneven capability degradation after model pruning
- Uses importance sampling to select optimal recovery data
- Achieves 94% of full fine-tuning performance using only 25% of the data
- Reduces negative transfer that can harm recovery efforts
This research enables more efficient deployment of compressed models in resource-constrained environments, offering practical solutions for engineering teams looking to balance model size and performance.
PASER: Post-Training Data Selection for Efficient Pruned Large Language Model Recovery