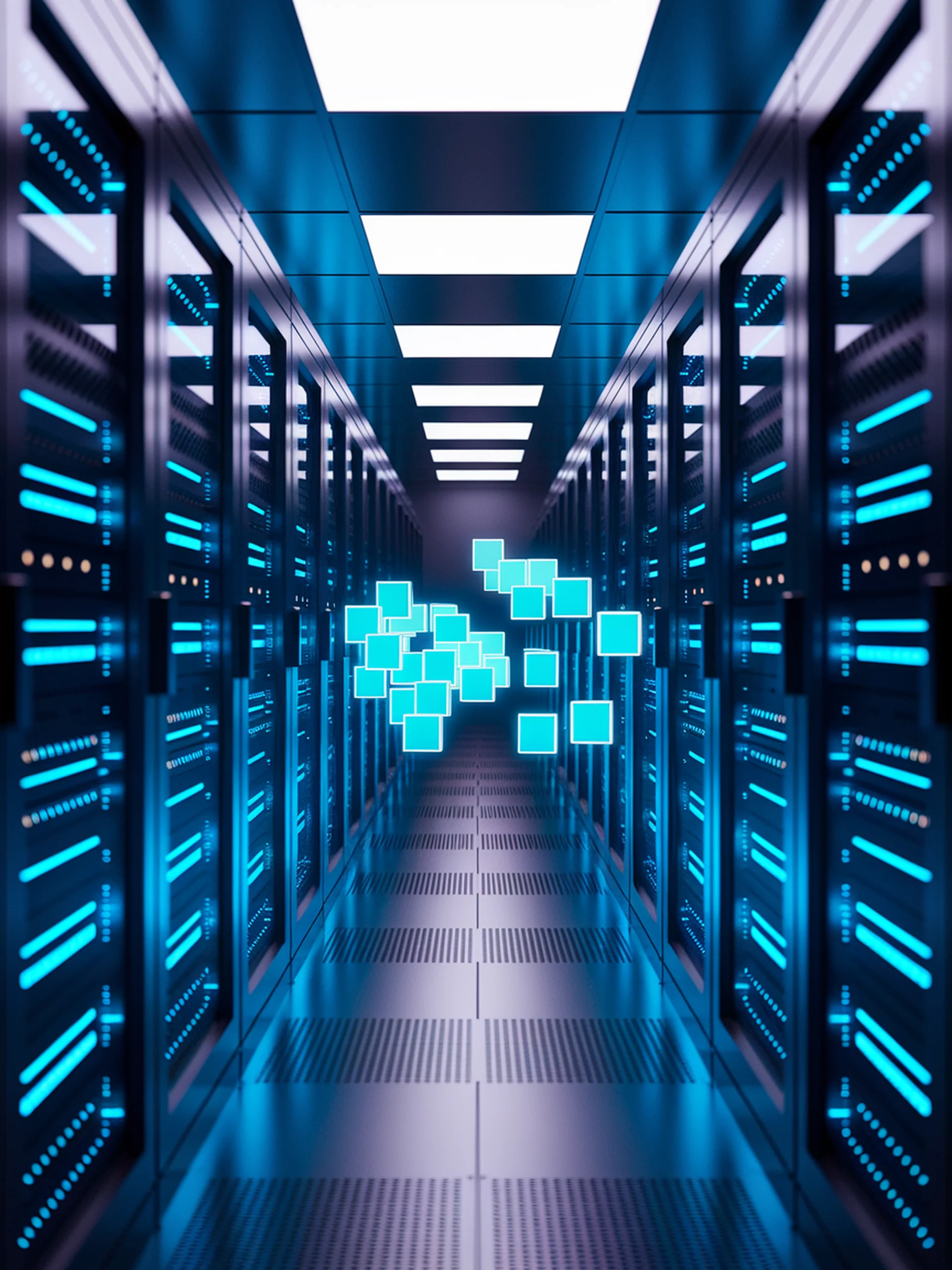
Making LLMs Faster with Smarter Memory Management
Novel techniques to reduce KV cache overhead for long-context models
This research introduces A²ATS (Attentive Agent Aware Token Selection), a system that dramatically improves efficiency for serving large language models with long contexts.
- Combines windowed rotary position embedding with query-aware vector quantization to reduce memory footprint
- Achieves more efficient retrieval of KV cache elements during inference
- Maintains accuracy while reducing computational burden compared to existing methods
- Addresses a critical bottleneck in deploying long-context LLMs at scale
For engineering teams, this approach offers practical solutions to the memory challenges that currently limit LLM deployment in production environments, potentially enabling more efficient serving of models with extremely long context windows.