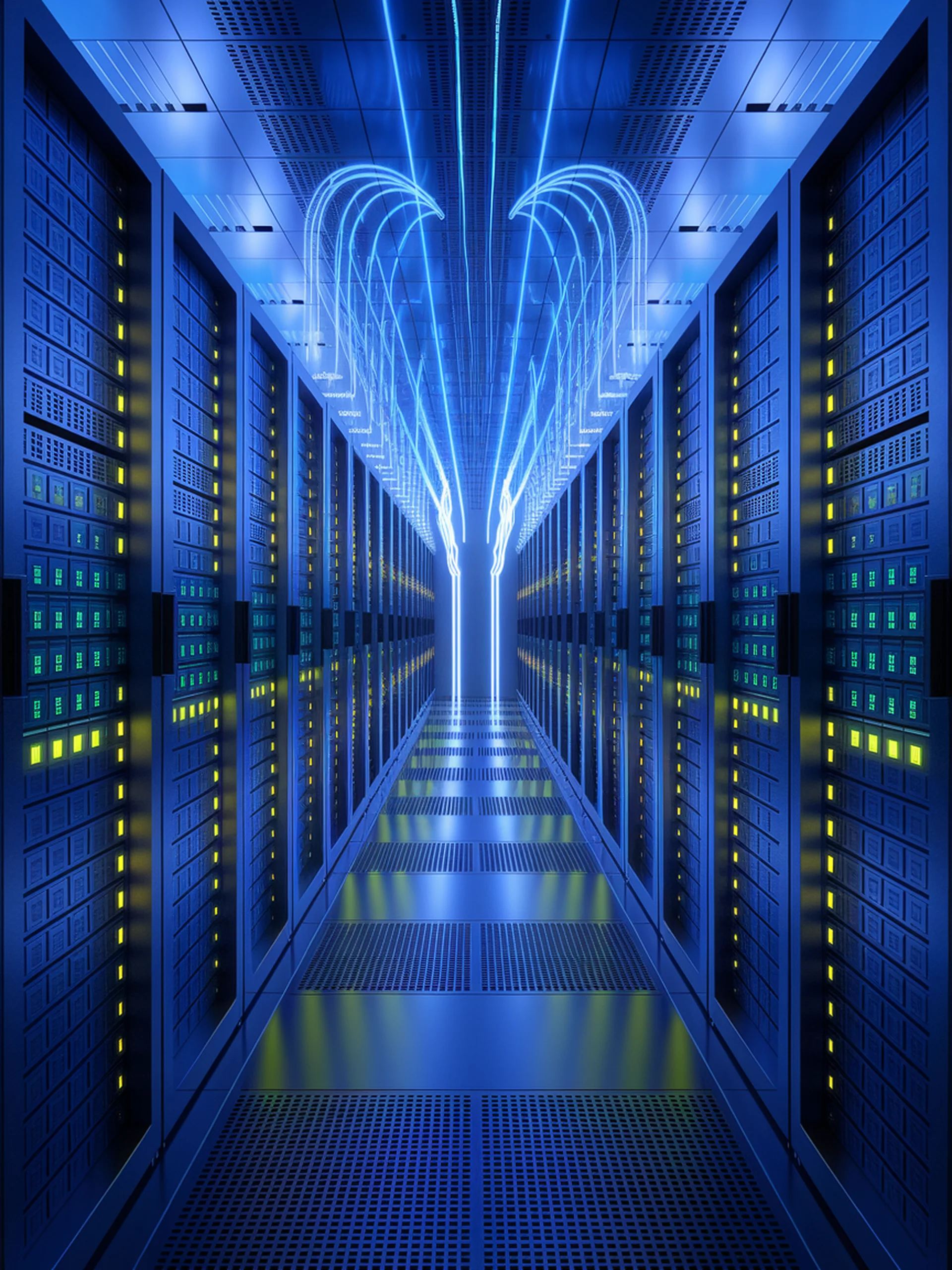
Accelerating Distributed AI Training
Efficient Communication-Computation Overlap in DiLoCo
This paper introduces eager updates to significantly improve distributed training of large AI models by overlapping communication and computation phases.
- Reduces training time by performing parameter updates in parallel with communication
- Achieves up to 50% reduction in communication overhead compared to standard DiLoCo
- Maintains convergence guarantees while improving hardware utilization
- Particularly valuable for multi-datacenter training scenarios with high network latency
This engineering advancement addresses a critical bottleneck in distributed systems, enabling more efficient scaling of very large model training across datacenters with minimal modification to existing infrastructure.
Eager Updates For Overlapped Communication and Computation in DiLoCo