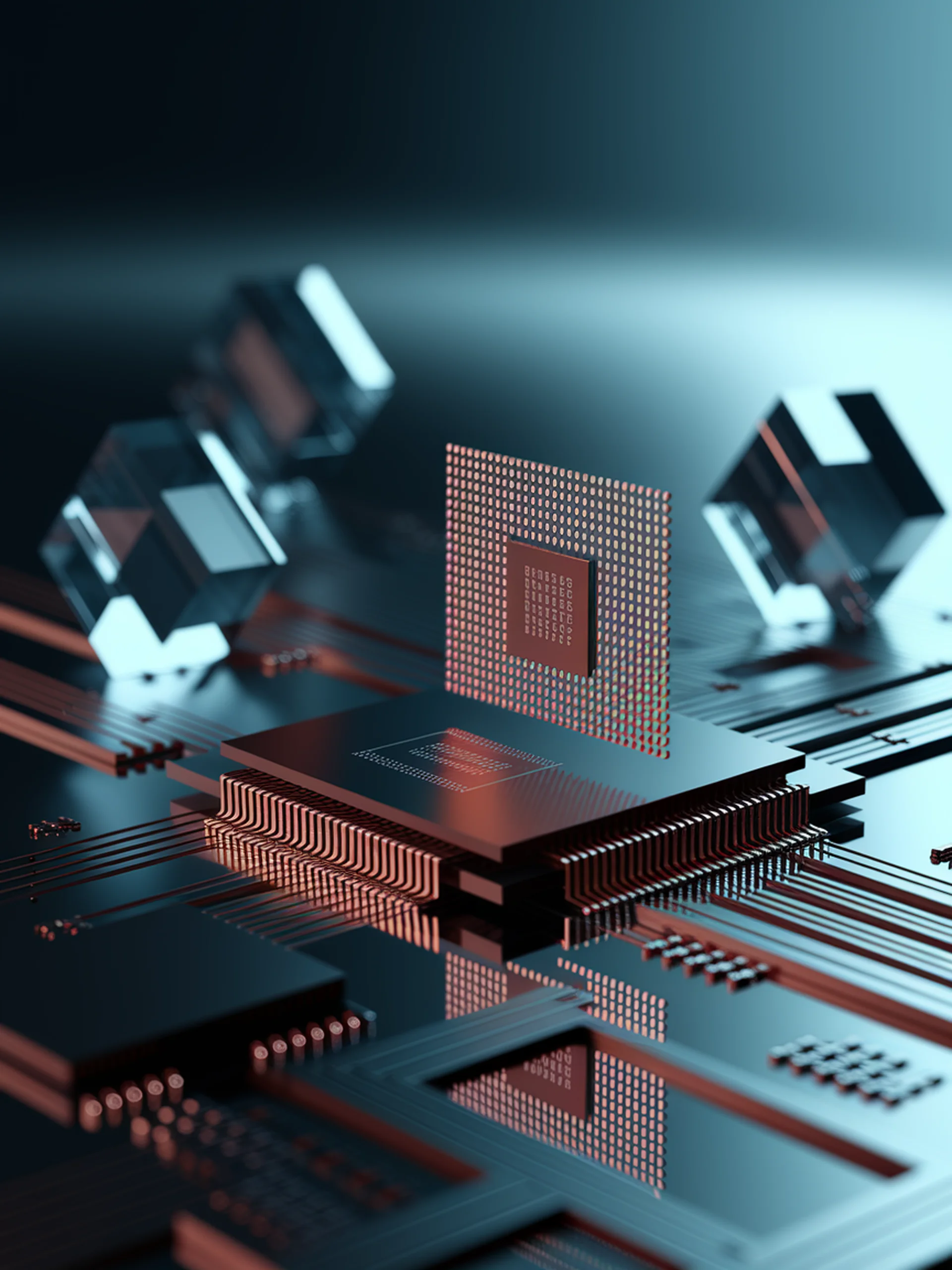
Optimizing LLMs Through Quantization
A Comprehensive Analysis of Post-Training Quantization Strategies
This research provides a systematic framework for evaluating and comparing Post-Training Quantization (PTQ) techniques for large language models, addressing the critical trade-offs between model size, performance, and quantization bitwidth.
- Introduces a novel benchmark methodology for categorizing and evaluating PTQ strategies
- Identifies optimal scenarios for deploying different quantization approaches
- Provides practical insights on efficiency-performance tradeoffs across various model sizes and bitwidths
- Delivers actionable guidance for engineering teams implementing LLM compression
For engineering teams deploying LLMs at scale, this research offers valuable direction on selecting the most appropriate quantization techniques to optimize deployment constraints while maintaining model performance.