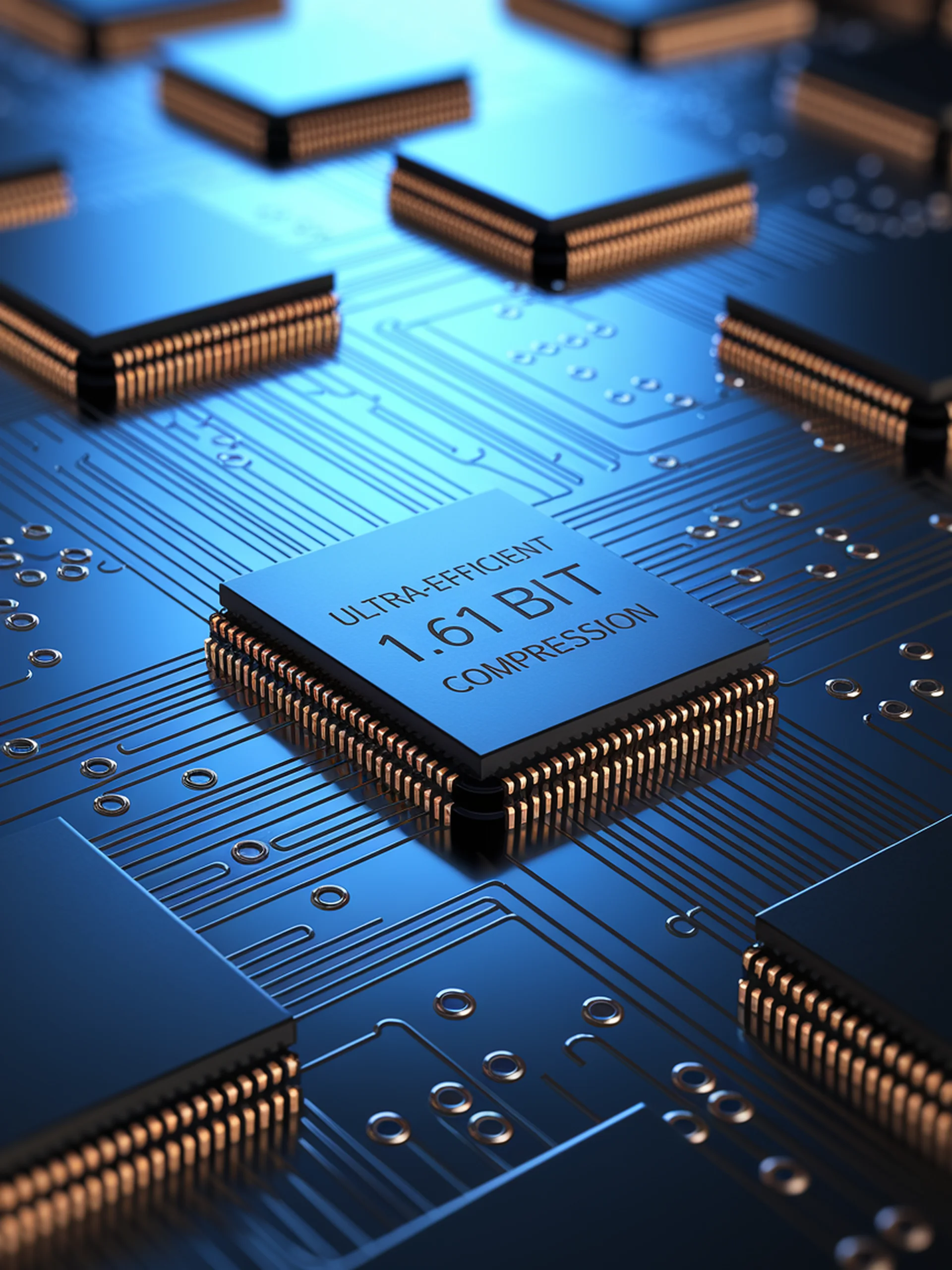
Breaking the 2-bit Barrier in LLM Compression
Pushing the limits of model efficiency with PTQ1.61
PTQ1.61 introduces a groundbreaking approach to extreme low-bit quantization for Large Language Models without performance collapse, achieving true 1.61 bits-per-weight compression.
- Eliminates the need for extra mask bits, delivering true sub-2-bit efficiency
- Preserves model performance where previous compression methods failed
- Enables deployment of powerful LLMs on resource-constrained devices
- Represents a significant engineering breakthrough for practical AI deployment
This research matters because it dramatically reduces the computational and memory requirements for running advanced language models, making AI more accessible and deployable across a wider range of hardware platforms.