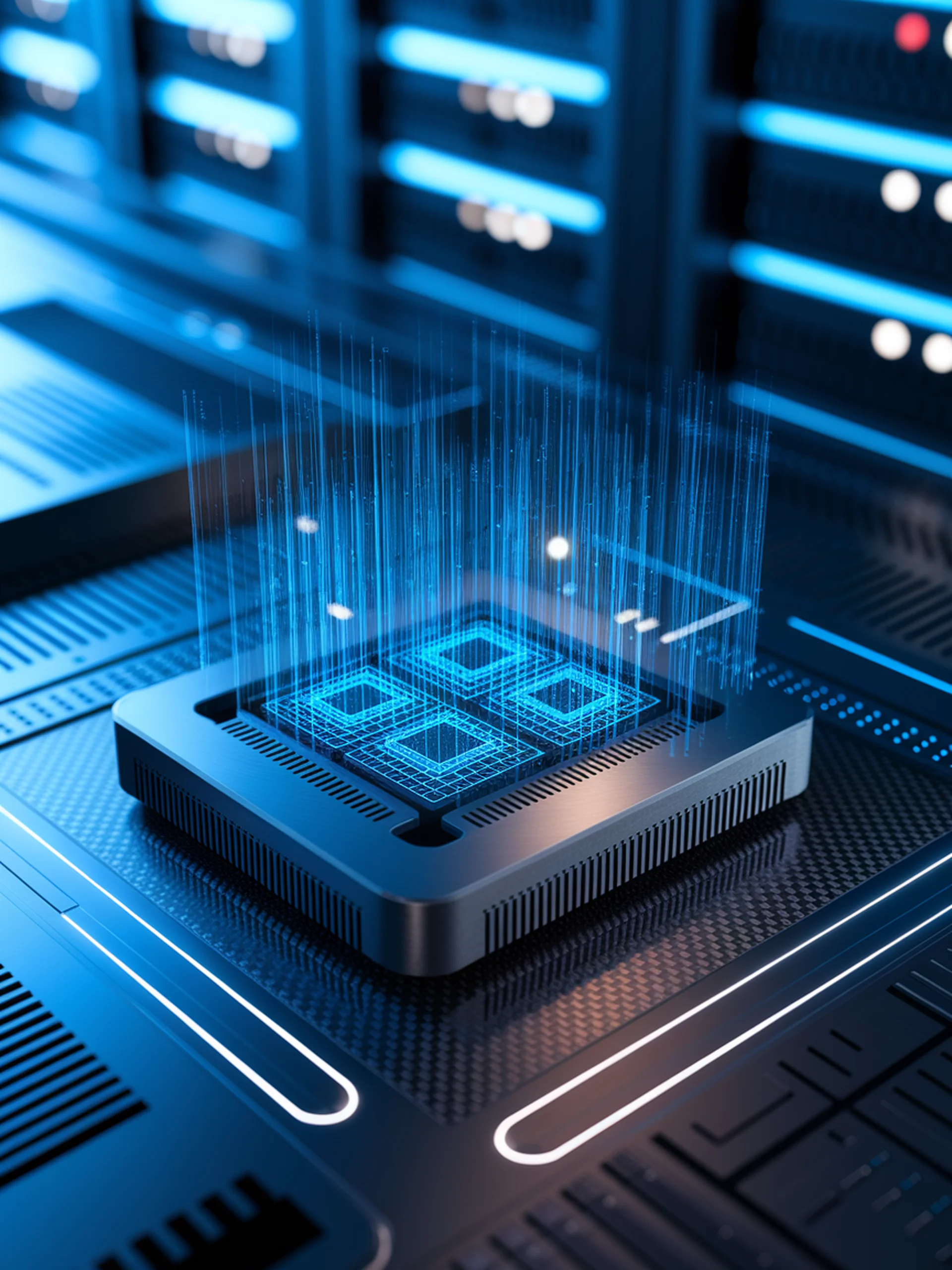
Streamlining LLMs with Tensor Operators
How PLDR-LLMs can replace their own neural networks at inference time
This research introduces a novel approach where Large Language Models can generate invariant tensor outputs and eventually replace their own deep neural networks during inference.
Key innovations:
- PLDR-LLMs learn a generalizable tensor operator that can substitute the model's deep neural network
- The approach focuses on identifying singularity conditions for deductive outputs
- Implementation includes a cache system for the energy-curvature tensor
- Potentially offers significant efficiency gains at inference time
Engineering Impact: This work represents a fundamental architectural advancement that could dramatically reduce computational requirements for LLMs during deployment, making them more efficient and practical for real-world applications.