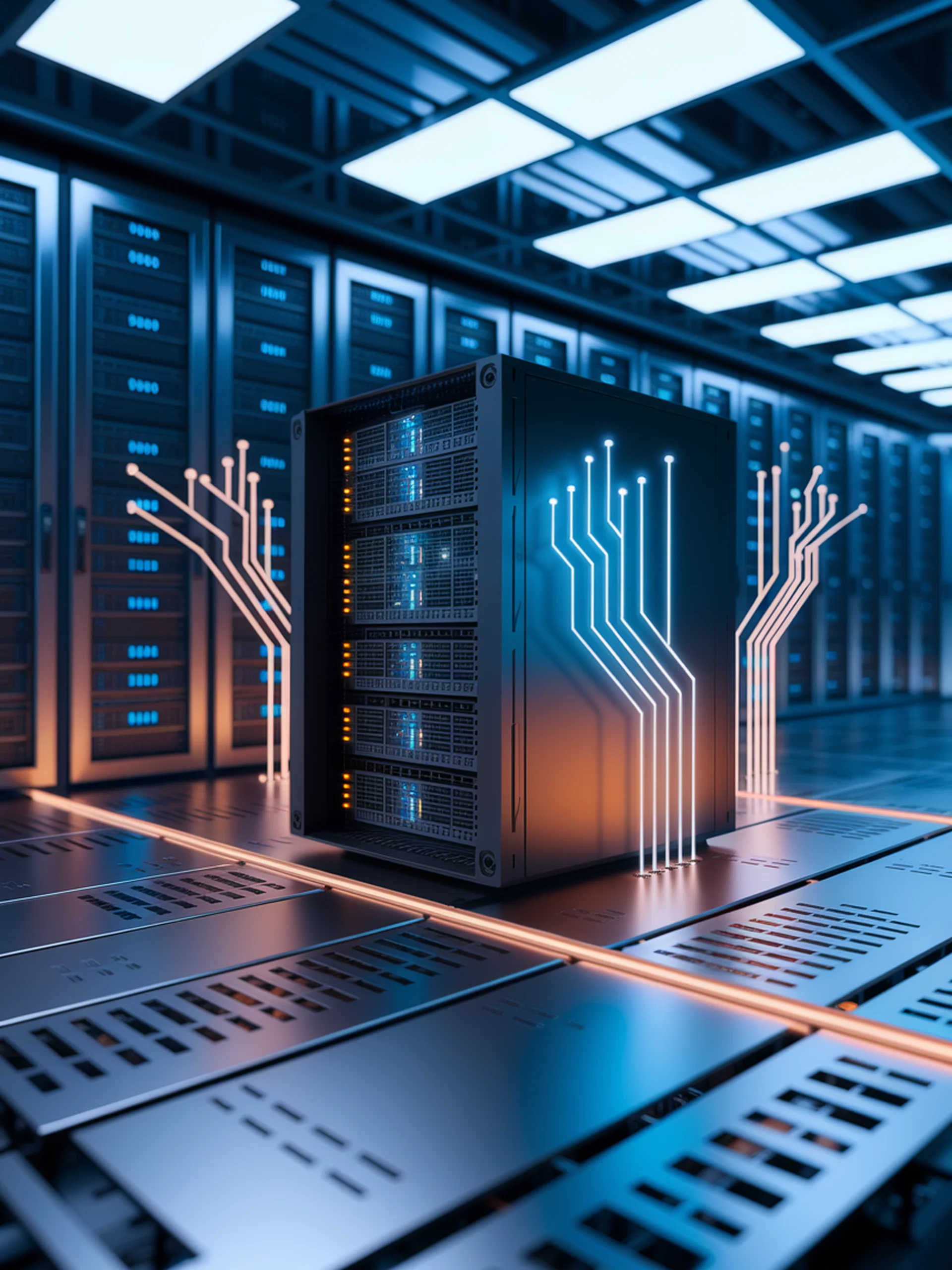
Accelerating LLM Inference with Smart Prediction
C2T: A classifier-based approach to optimize speculative decoding
C2T introduces an innovative classifier-based method for tree construction in speculative decoding, significantly reducing inference latency and computational costs of large language models.
- Uses a lightweight classifier to dynamically predict token probabilities
- Constructs optimized token trees for more efficient verification processes
- Demonstrates superior performance compared to chain mode and static/dynamic tree approaches
- Addresses critical inference bottlenecks as LLMs continue to scale in size
This engineering advancement matters because it makes large language models more practical and cost-effective for real-world applications, enabling faster response times without sacrificing quality.
C2T: A Classifier-Based Tree Construction Method in Speculative Decoding