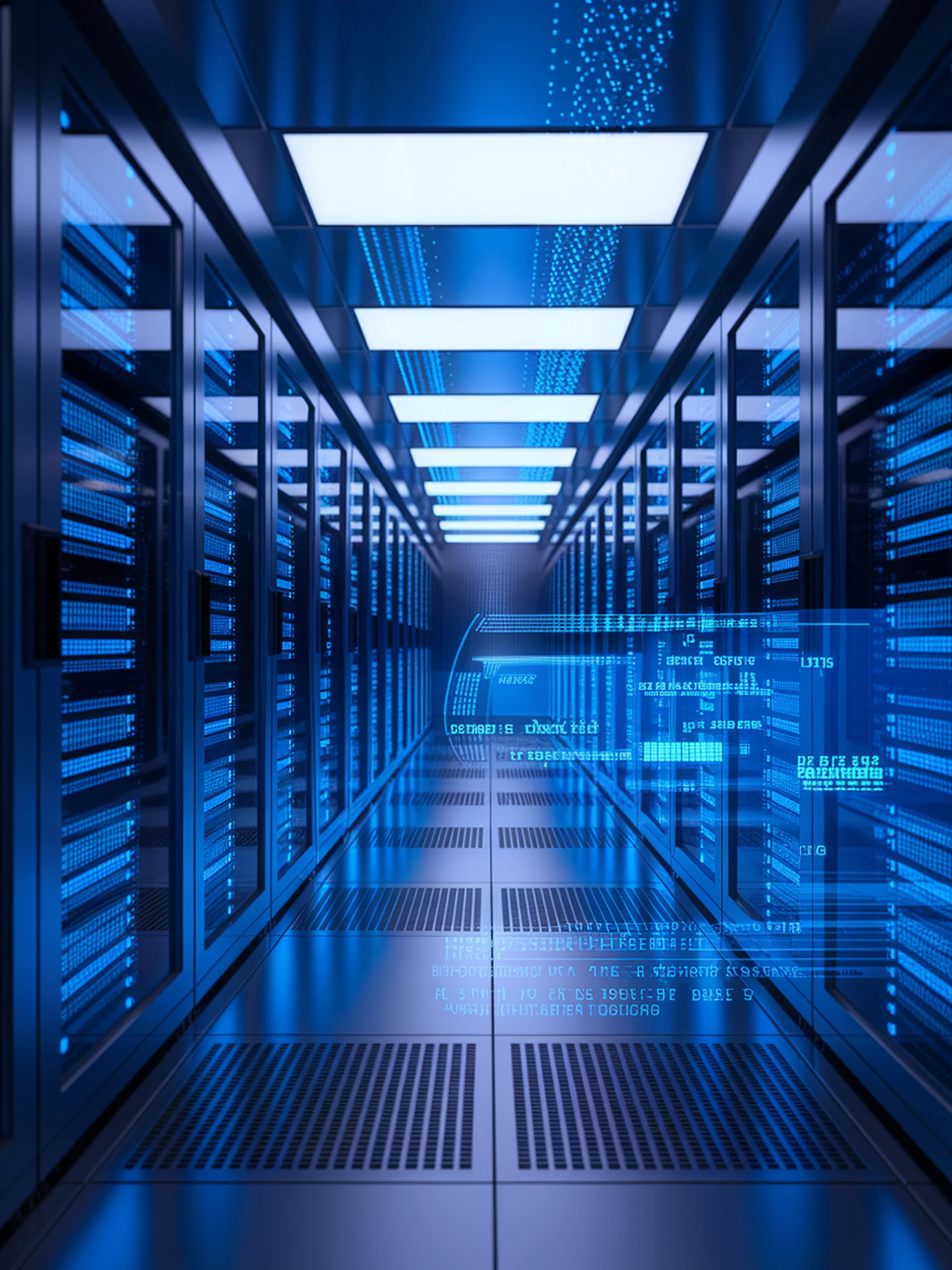
Fast-Track for Long-Context LLMs
Optimizing LLM serving with unified sparse attention
LServe introduces a novel system to dramatically improve long-sequence LLM performance by addressing the two major bottlenecks: attention computation and KV cache memory usage.
- Unifies sparse attention approaches for both prefilling and decoding stages
- Achieves up to 2.3× latency reduction and 5.8× throughput improvement
- Maintains high accuracy while significantly reducing memory footprint
- Demonstrates practical implementation across different LLM architectures
This research resolves a critical engineering challenge by making long-context LLMs more feasible for real-world applications, enabling more efficient text generation while preserving quality.
LServe: Efficient Long-sequence LLM Serving with Unified Sparse Attention