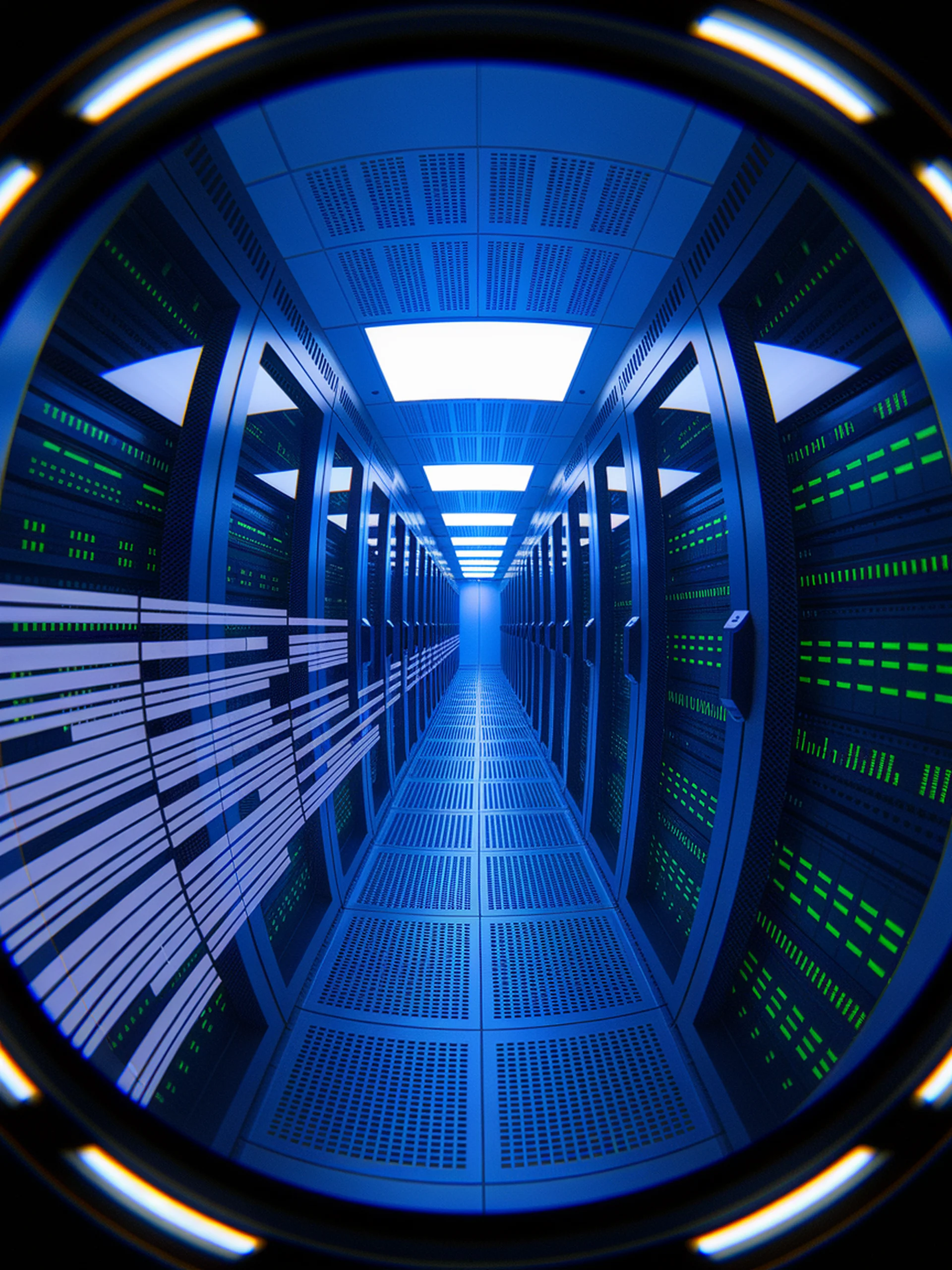
Accelerating LLM Conversations with Round Attention
A targeted approach to reduce memory overhead in multi-turn dialogues
Round Attention introduces a novel mechanism that selectively prunes KV caches in multi-round LLM conversations, significantly improving inference speed without sacrificing quality.
- Identifies a critical watershed layer in LLM architecture where round-level attention becomes less important
- Achieves up to 50% reduction in memory consumption while maintaining output quality
- Enables larger context windows for more complex conversations without hardware upgrades
- Provides a practical solution for deployment in resource-constrained environments
This engineering breakthrough directly addresses the escalating memory demands of modern LLMs, making advanced conversational AI more accessible and cost-effective for production systems.
Round Attention: A Novel Round-Level Attention Mechanism to Accelerate LLM Inference