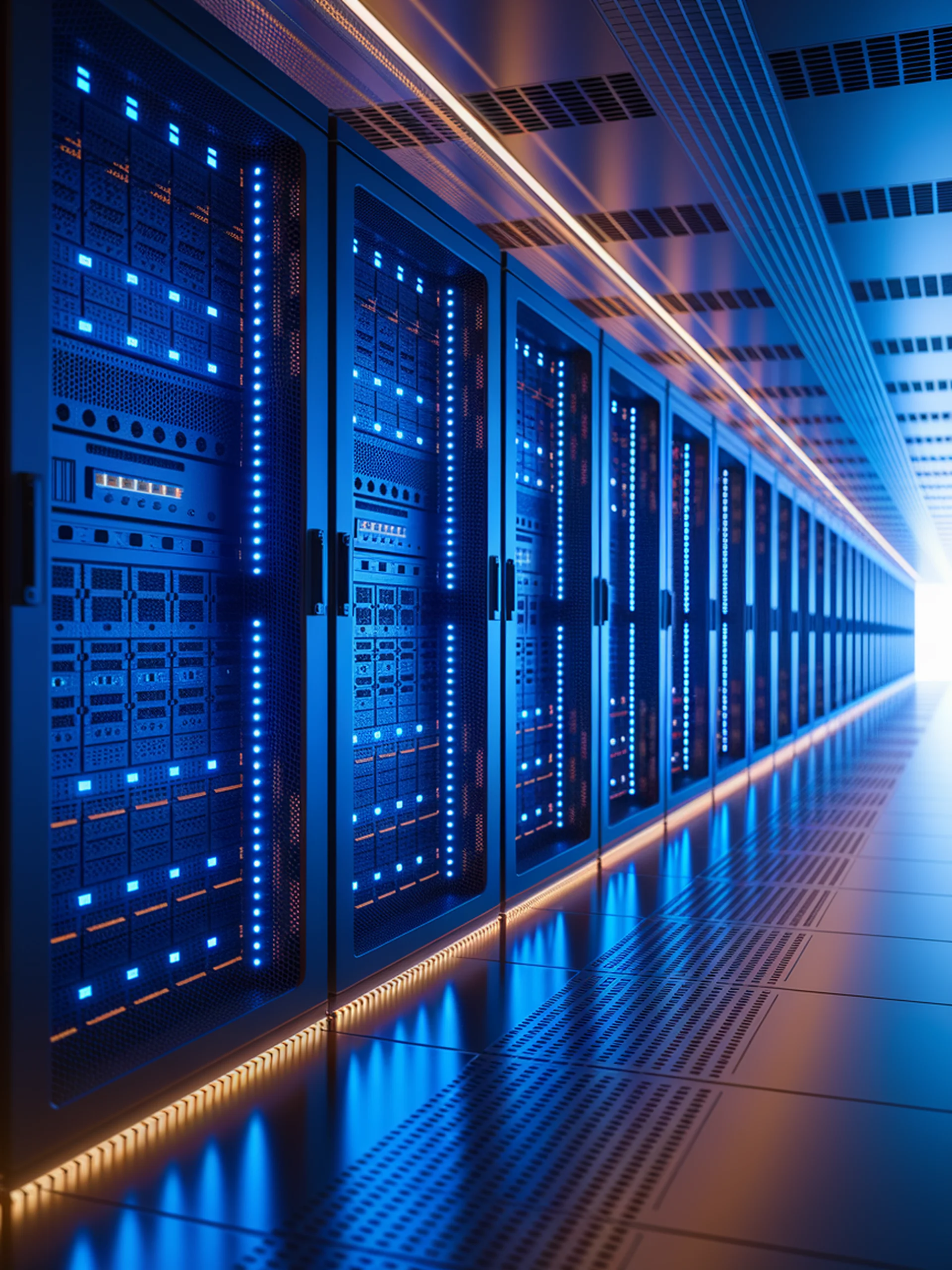
Dynamic Pruning for Faster LLMs
Accelerating large language models through intelligent token-based pruning
Probe Pruning (PP) introduces a framework that dynamically prunes LLMs on-the-fly by identifying which weights matter most for specific inputs.
- Leverages the insight that not all tokens contribute equally to model outputs
- Implements a three-stage approach: probing, history-informed pruning, and full inference
- Achieves computational efficiency through batch-wise pruning
- Maintains model performance while reducing processing requirements
This engineering innovation addresses a critical challenge in LLM deployment: balancing computational demands with performance requirements. By intelligently pruning models based on input characteristics, organizations can deploy more efficient AI systems.
Probe Pruning: Accelerating LLMs through Dynamic Pruning via Model-Probing