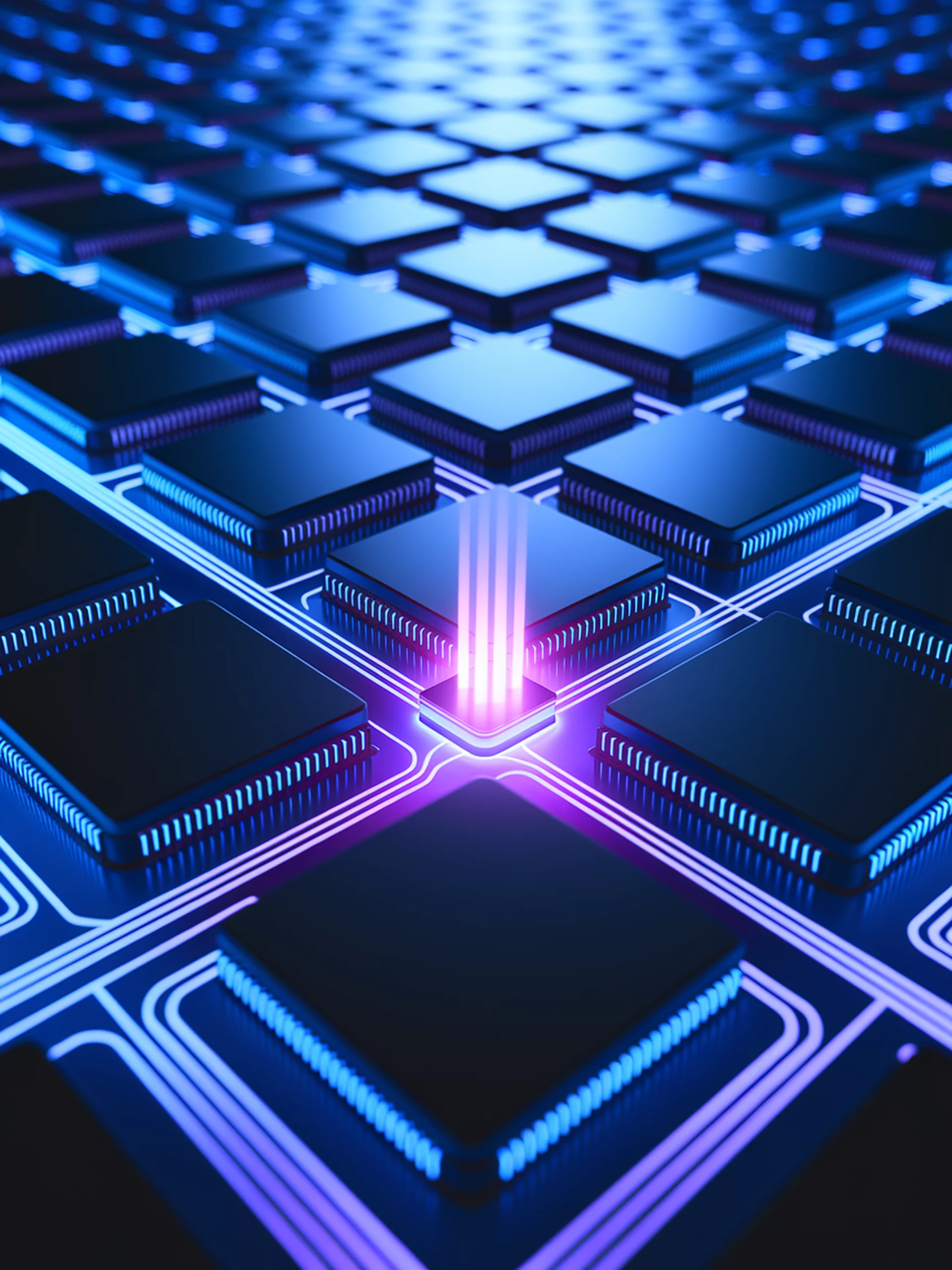
Accelerating LLM Inference with CORAL
Consistent Representation Learning for More Efficient Speculative Decoding
CORAL improves speculative decoding by solving the representation inconsistency problem between draft models and target LLMs, enabling faster inference with lighter models.
- Creates consistent representations across multi-step training phases
- Implements novel weight-grouping mechanism to improve alignment
- Achieves acceleration of 1.7-2.0x compared to baseline techniques
- Enables effective use of smaller draft models while maintaining quality
This engineering breakthrough directly addresses computational efficiency bottlenecks in LLM inference, making deployment more cost-effective while preserving output quality.