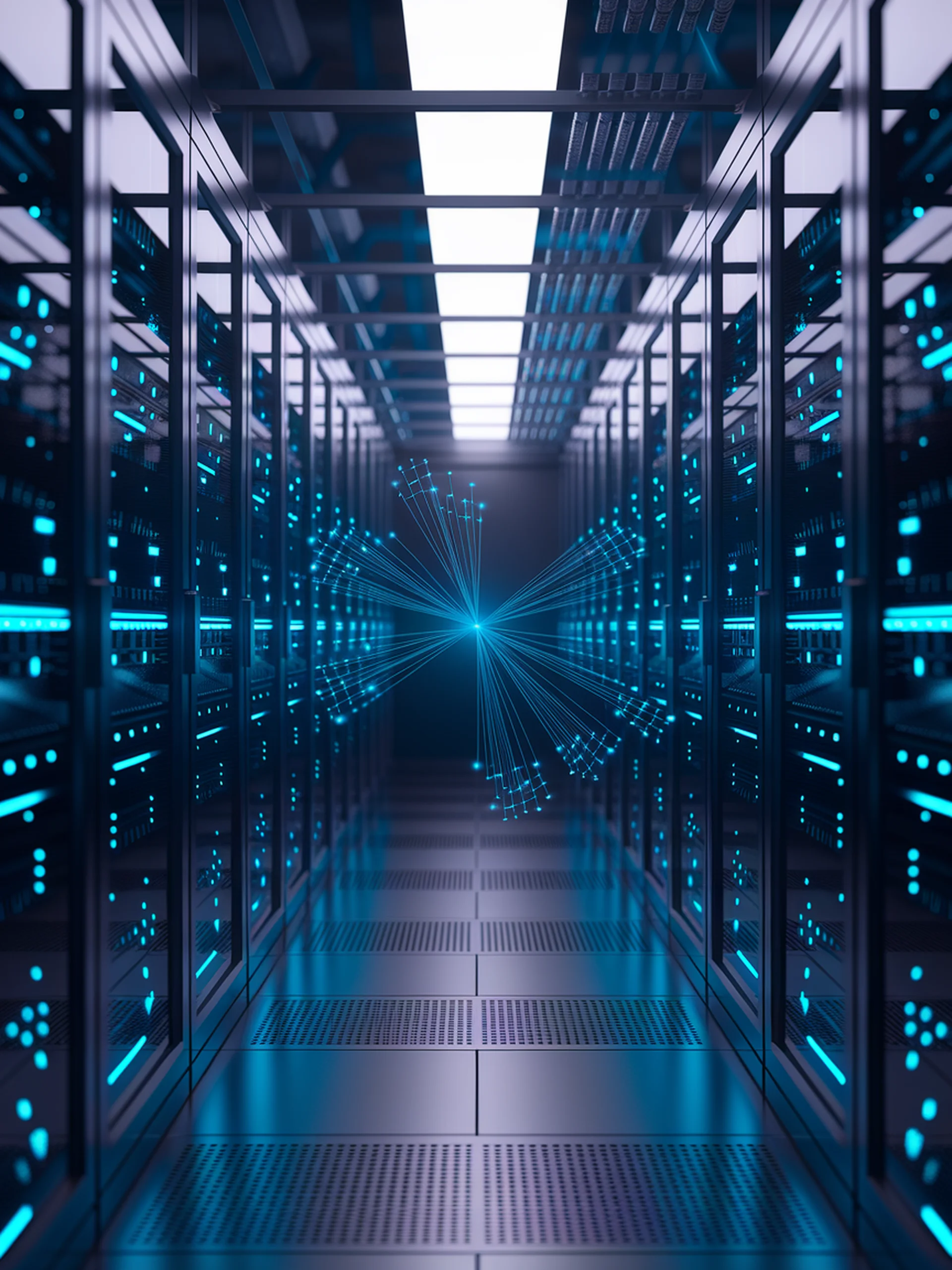
Shrinking MoE Language Models
Innovative compression through delta decompression technique
D²-MoE introduces a novel compression approach for Mixture-of-Experts (MoE) language models by decomposing expert weights into shared base weights and unique delta components.
- Leverages expert similarity patterns to dramatically reduce storage requirements
- Combines delta decompression with SVD and structured pruning techniques
- Demonstrates effective parameter reduction while maintaining model performance
- Addresses critical deployment challenges for resource-intensive MoE architectures
This engineering breakthrough makes advanced MoE models more practical for real-world applications by reducing their prohibitive memory and storage demands.
Original paper: Delta Decompression for MoE-based LLMs Compression