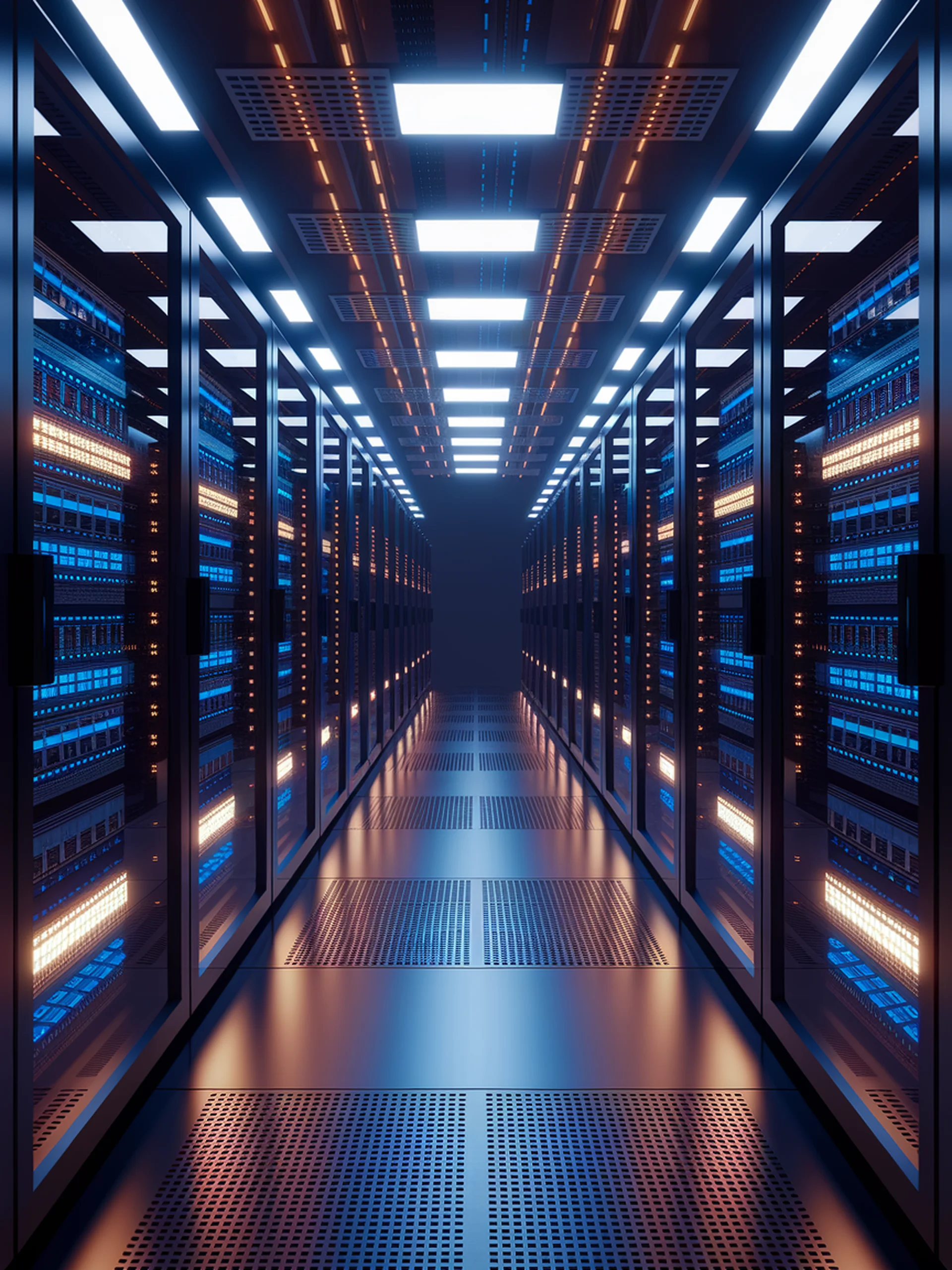
COSMOS: Revolutionizing LLM Optimization
A memory-efficient hybrid approach for training large language models
COSMOS introduces a novel hybrid adaptive optimizer that significantly reduces memory requirements while improving optimization performance for training Large Language Models.
- Addresses critical limitations of AdamW including high memory consumption
- Captures interdependencies between coordinates that traditional optimizers miss
- Balances computational efficiency with optimization performance
- Provides a practical solution for training increasingly larger language models
This research matters for Engineering because it tackles one of the fundamental bottlenecks in scaling AI systems: the memory overhead of optimization algorithms. By making LLM training more efficient, COSMOS enables researchers and companies to build more powerful models with existing computational resources.
COSMOS: A Hybrid Adaptive Optimizer for Memory-Efficient Training of LLMs