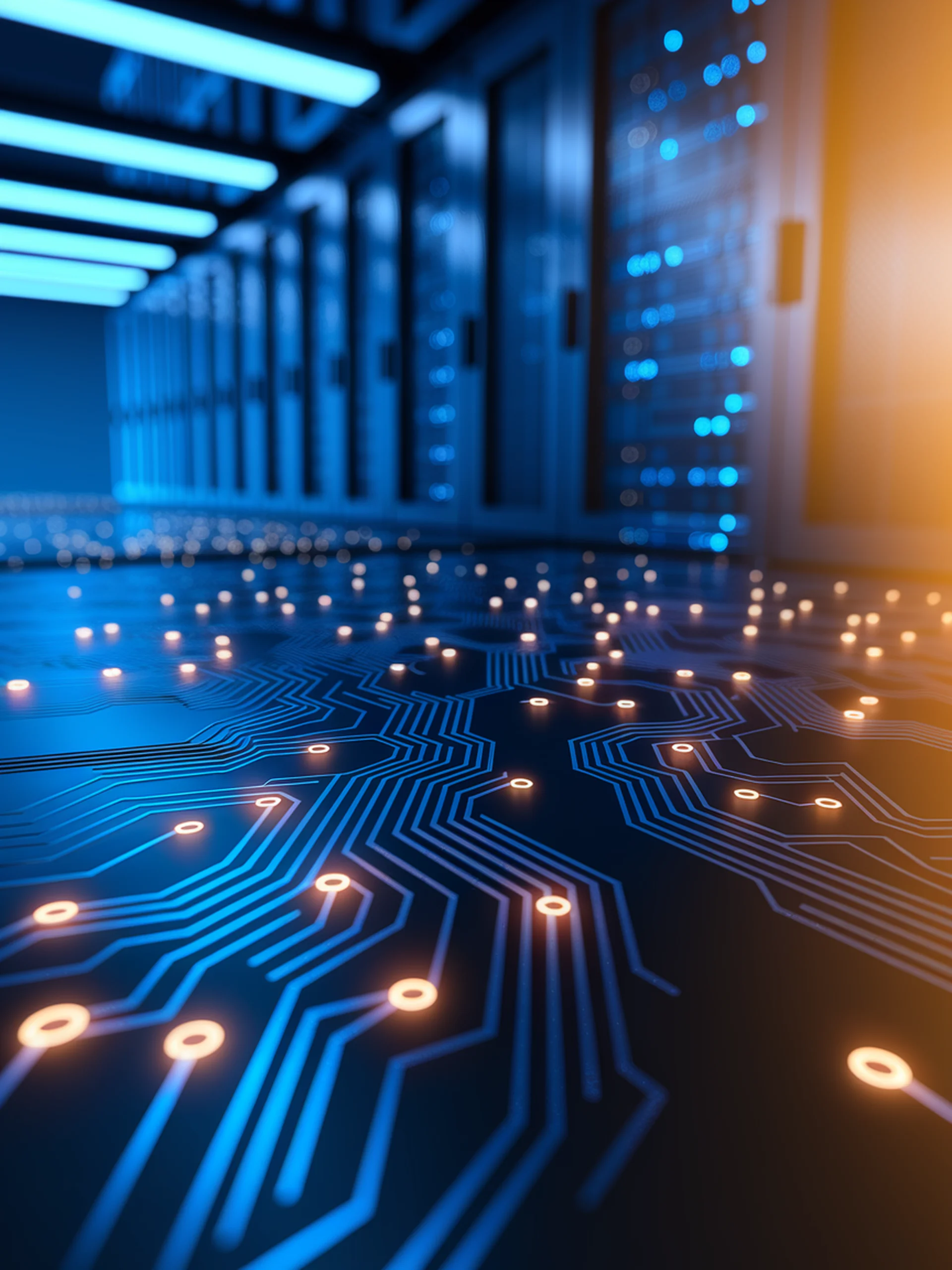
Accelerating LLMs with Sparse Attention
A universal approach to optimizing attention for any model
SpargeAttn introduces a universally applicable sparse attention mechanism that accelerates model inference by efficiently identifying and skipping near-zero attention values.
- Reduces the quadratic time complexity of attention calculations
- Works across different model architectures without model-specific customization
- Combines sparse attention patterns with efficient quantization techniques
- Achieves significant speed improvements while maintaining model accuracy
This engineering breakthrough matters because it addresses one of the key bottlenecks in LLM deployment, potentially enabling faster inference across a wide range of applications with existing models.
SpargeAttn: Accurate Sparse Attention Accelerating Any Model Inference