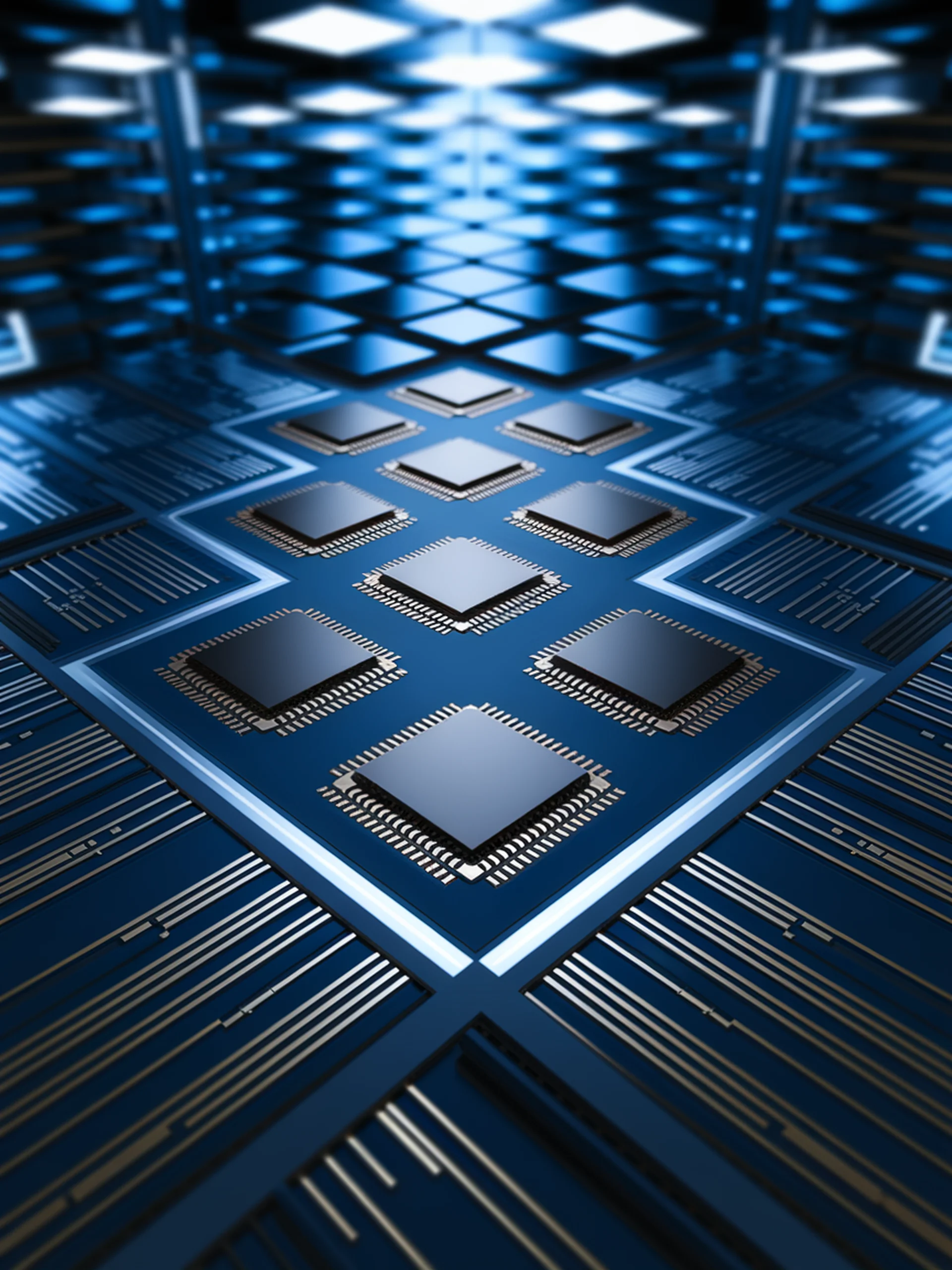
Smart Compression for LLMs
Boosting Efficiency with Adaptive Data Types
This research introduces M-ANT, a breakthrough approach for LLM compression using mathematically adaptive numerical types that significantly improves efficiency without sacrificing accuracy.
- Employs fine-grained group-wise quantization that treats small tensor clusters as compression units
- Achieves superior compression rates with custom adaptive data types specifically designed for LLM weight distributions
- Delivers practical implementation for both hardware and software deployment scenarios
- Enables more efficient LLM operation on resource-constrained devices
For engineering teams, M-ANT provides a ready-to-deploy solution for running large language models with significantly reduced memory and computational requirements, making advanced AI more accessible across different hardware platforms.
M-ANT: Efficient Low-bit Group Quantization for LLMs via Mathematically Adaptive Numerical Type