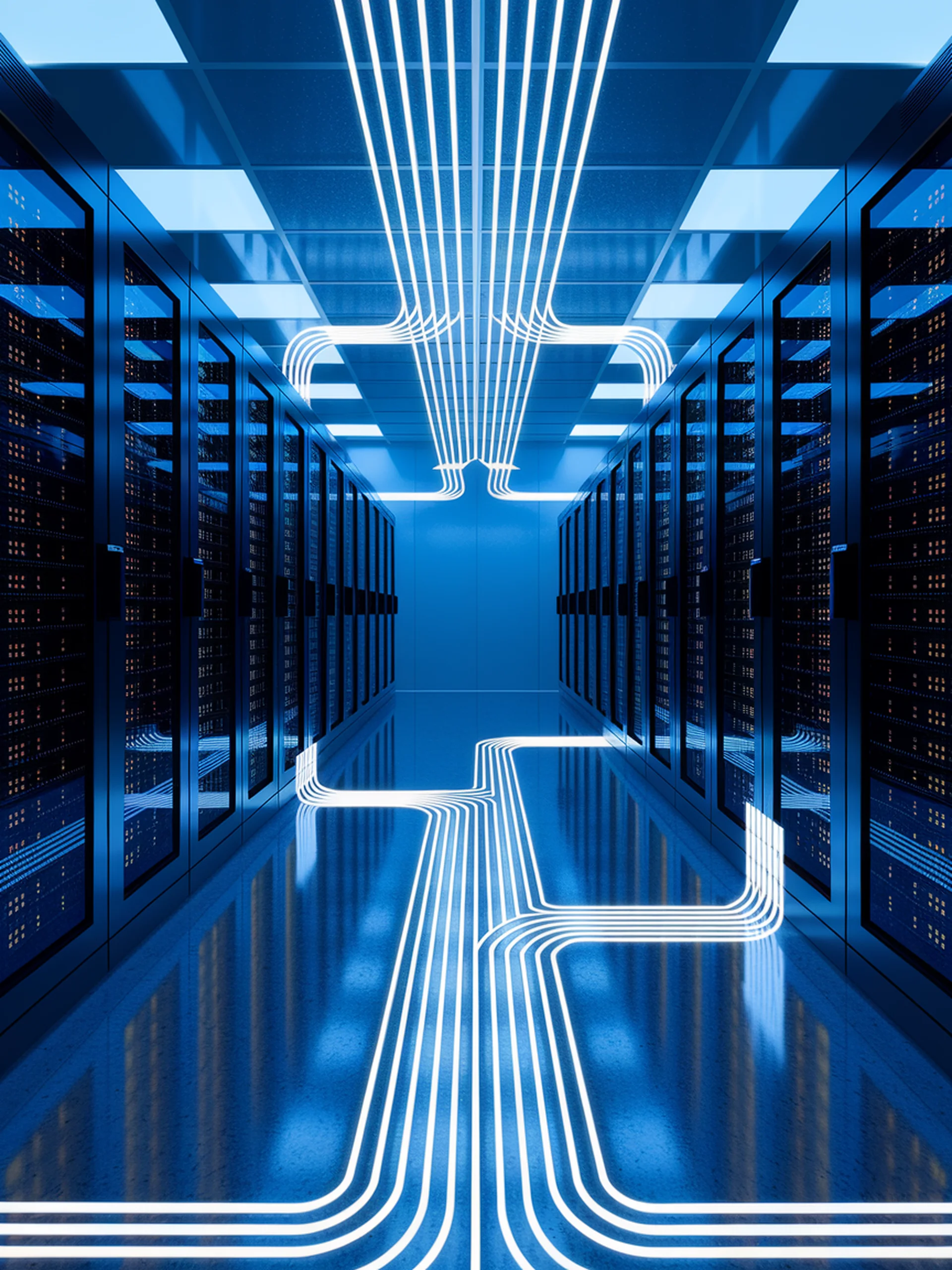
Optimizing LLM Training with SkipPipe
Breaking the sequential pipeline paradigm for faster, cost-effective LLM training
SkipPipe introduces a novel framework that enables partial and reordered pipeline execution for Large Language Model training, significantly improving efficiency in heterogeneous network environments.
- Leverages LLMs' resistance to layer skipping and layer reordering to create flexible training pipelines
- Challenges conventional sequential pipeline execution with a more adaptable approach
- Optimizes communication patterns to reduce training costs while maintaining model quality
- Designed specifically for distributed computing environments with varying hardware capabilities
This breakthrough matters because it addresses one of the core engineering challenges in modern AI: making LLM training more accessible and cost-effective across diverse computing infrastructures, potentially democratizing access to advanced AI development.
SkipPipe: Partial and Reordered Pipelining Framework for Training LLMs in Heterogeneous Networks