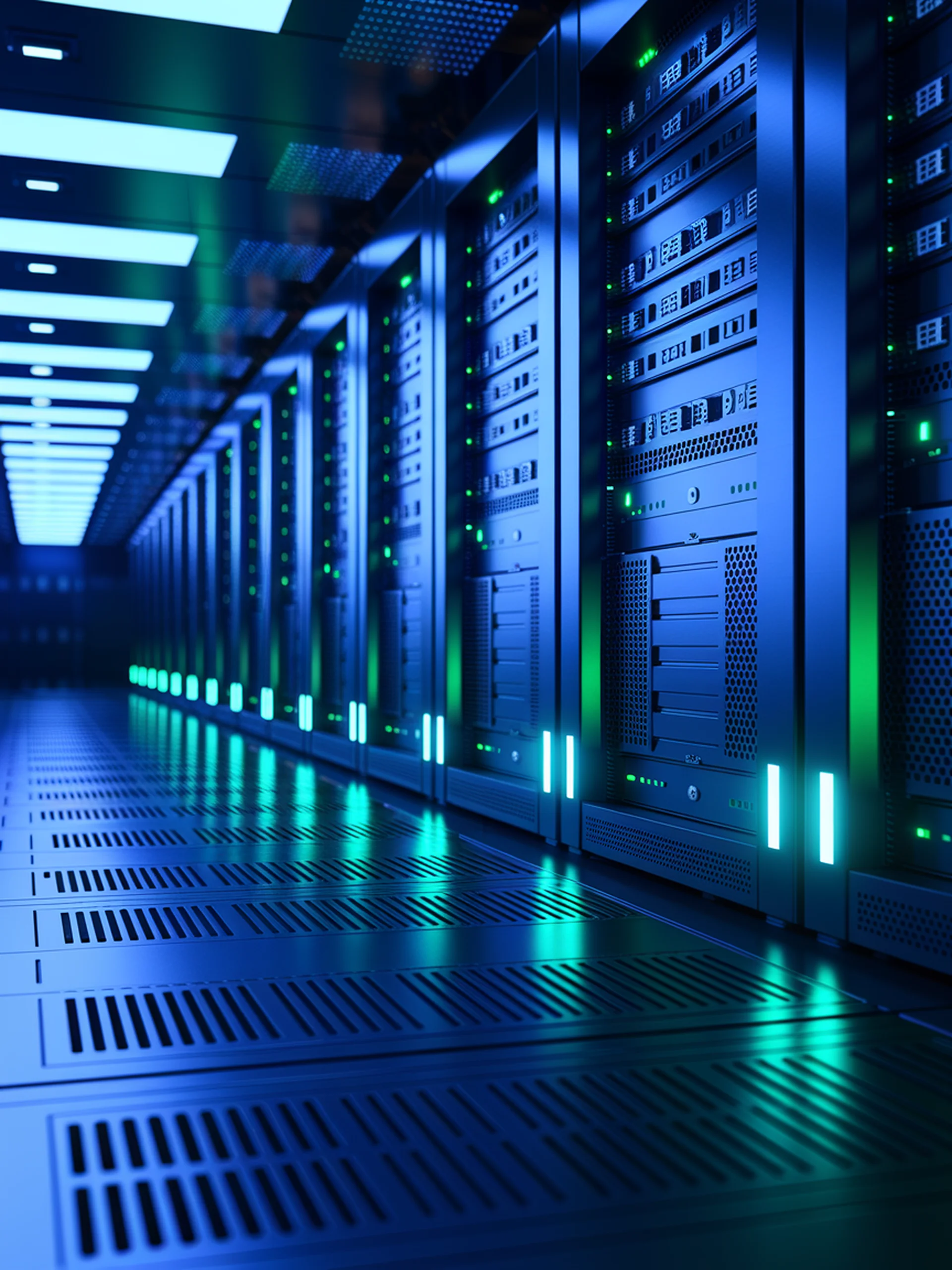
Optimizing LLM Inference Across Multiple GPUs
Reducing Communication Bottlenecks in Tensor Parallelism
Sync-Point Drop (SPD) is a novel optimization technique that selectively eliminates synchronization points in tensor-parallel LLM inference, significantly reducing communication overhead.
- Addresses a critical bottleneck in distributed LLM inference across multiple computing units
- Selectively drops unnecessary synchronization points to improve throughput and latency
- Maintains model accuracy while enhancing inference efficiency
- Enables better scaling of large language models across distributed systems
This engineering breakthrough is particularly valuable as LLMs continue to grow in size, making efficient distributed inference essential for practical deployment in production environments.
SPD: Sync-Point Drop for efficient tensor parallelism of Large Language Models